Posted by Alireza Fathi, Research Scientist and Rui Huang, AI Resident, Google Research
The growing ubiquity of 3D sensors (e.g., Lidar, depth sensing cameras and radar) over the last few years has created a need for scene understanding technology that can process the data these devices capture. Such technology can enable machine learning (ML) systems that use these sensors, like autonomous cars and robots, to navigate and operate in the real world, and can create an improved augmented reality experience on mobile devices. The field of computer vision has recently begun making good progress in 3D scene understanding, including models for mobile 3D object detection, transparent object detection, and more, but entry to the field can be challenging due to the limited availability tools and resources that can be applied to 3D data.
In order to further improve 3D scene understanding and reduce barriers to entry for interested researchers, we are releasing TensorFlow 3D (TF 3D), a highly modular and efficient library that is designed to bring 3D deep learning capabilities into TensorFlow. TF 3D provides a set of popular operations, loss functions, data processing tools, models and metrics that enables the broader research community to develop, train and deploy state-of-the-art 3D scene understanding models.
TF 3D contains training and evaluation pipelines for state-of-the-art 3D semantic segmentation, 3D object detection and 3D instance segmentation, with support for distributed training. It also enables other potential applications like 3D object shape prediction, point cloud registration and point cloud densification. In addition, it offers a unified dataset specification and configuration for training and evaluation of the standard 3D scene understanding datasets. It currently supports the Waymo Open, ScanNet, and Rio datasets. However, users can freely convert other popular datasets, such as NuScenes and Kitti, into a similar format and use them in the pre-existing or custom created pipelines, and can leverage TF 3D for a wide variety of 3D deep learning research and applications, from quickly prototyping and trying new ideas to deploying a real-time inference system.
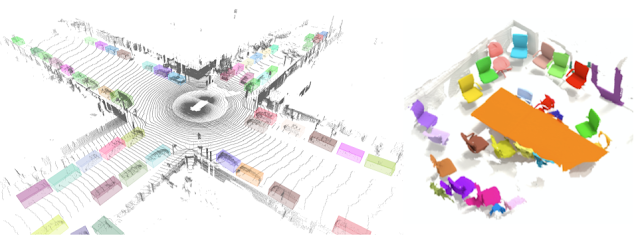 |
An example output of the 3D object detection model in TF 3D on a frame from Waymo Open Dataset is shown on the left. An example output of the 3D instance segmentation model on a scene from ScanNet dataset is shown on the right. |
Here, we will present the efficient and configurable sparse convolutional backbone that is provided in TF 3D, which is the key to achieving state-of-the-art results on various 3D scene understanding tasks. Furthermore, we will go over each of the three pipelines that TF 3D currently supports: 3D semantic segmentation, 3D object detection and 3D instance segmentation.
3D Sparse Convolutional Network
The 3D data captured by sensors often consists of a scene that contains a set of objects of interest (e.g. cars, pedestrians, etc.) surrounded mostly by open space, which is of limited (or no) interest. As such, 3D data is inherently sparse. In such an environment, standard implementation of convolutions would be computationally intensive and consume a large amount of memory. So, in TF 3D we use submanifold sparse convolution and pooling operations, which are designed to process 3D sparse data more efficiently. Sparse convolutional models are core to the state-of-the-art methods applied in most outdoor self-driving (e.g. Waymo, NuScenes) and indoor benchmarks (e.g. ScanNet).
We also use various CUDA techniques to speed up the computation (e.g., hashing, partitioning / caching the filter in shared memory, and using bit operations). Experiments on the Waymo Open dataset shows that this implementation is around 20x faster than a well-designed implementation with pre-existing TensorFlow operations.
TF 3D then uses the 3D submanifold sparse U-Net architecture to extract a feature for each voxel. The U-Net architecture has proven to be effective by letting the network extract both coarse and fine features and combining them to make the predictions. The U-Net network consists of three modules, an encoder, a bottleneck, and a decoder, each of which consists of a number of sparse convolution blocks with possible pooling or un-pooling operations.
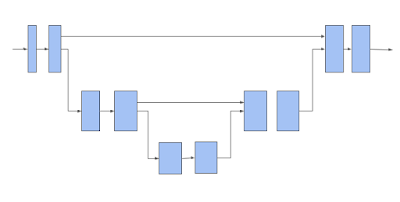 |
A 3D sparse voxel U-Net architecture. Note that a horizontal arrow takes in the voxel features and applies a submanifold sparse convolution to it. An arrow that is moving down performs a submanifold sparse pooling. An arrow that is moving up will gather back the pooled features, concatenate them with the features coming from the horizontal arrow, and perform a submanifold sparse convolution on the concatenated features. |
The sparse convolutional network described above is the backbone for the 3D scene understanding pipelines that are offered in TF 3D. Each of the models described below uses this backbone network to extract features for the sparse voxels, and then adds one or multiple additional prediction heads to infer the task of interest. The user can configure the U-Net network by changing the number of encoder / decoder layers and the number of convolutions in each layer, and by modifying the convolution filter sizes, which enables a wide range of speed / accuracy tradeoffs to be explored through the different backbone configurations
3D Semantic Segmentation
The 3D semantic segmentation model has only one output head for predicting the per-voxel semantic scores, which are mapped back to points to predict a semantic label per point.
3D Instance Segmentation
In 3D instance segmentation, in addition to predicting semantics, the goal is to group the voxels that belong to the same object together. The 3D instance segmentation algorithm used in TF 3D is based on our previous work on 2D image segmentation using deep metric learning. The model predicts a per-voxel instance embedding vector as well as a semantic score for each voxel. The instance embedding vectors map the voxels to an embedding space where voxels that correspond to the same object instance are close together, while those that correspond to different objects are far apart. In this case, the input is a point cloud instead of an image, and it uses a 3D sparse network instead of a 2D image network. At inference time, a greedy algorithm picks one instance seed at a time, and uses the distance between the voxel embeddings to group them into segments.
3D Object Detection
The 3D object detection model predicts per-voxel size, center, and rotation matrices and the object semantic scores. At inference time, a box proposal mechanism is used to reduce the hundreds of thousands of per-voxel box predictions into a few accurate box proposals, and then at training time, box prediction and classification losses are applied to per-voxel predictions. We apply a Huber loss on the distance between predicted and the ground-truth box corners. Since the function that estimates the box corners from its size, center and rotation matrix is differentiable, the loss will automatically propagate back to those predicted object properties. We use a dynamic box classification loss that classifies a box that strongly overlaps with the ground-truth as positive and classifies the non-overlapping boxes as negative.
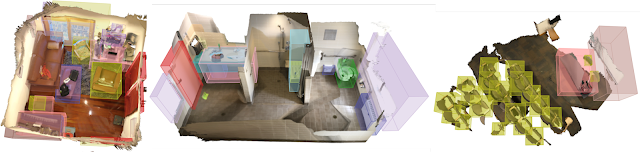 |
Our 3D object detection results on ScanNet dataset. |
In our recent paper, “DOPS: Learning to Detect 3D Objects and Predict their 3D Shapes”, we describe in detail the single-stage weakly supervised learning algorithm used for object detection in TF 3D. In addition, in a follow up work, we extended the 3D object detection model to leverage temporal information by proposing a sparse LSTM-based multi-frame model. We go on to show that this temporal model outperforms the frame-by-frame approach by 7.5% in the Waymo Open dataset.
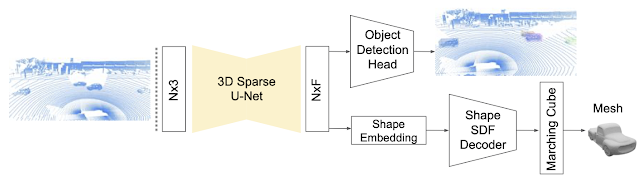 |
The 3D object detection and shape prediction model introduced in the DOPS paper. A 3D sparse U-Net is used to extract a feature vector for each voxel. The object detection module uses these features to propose 3D boxes and semantic scores. At the same time, the other branch of the network predicts a shape embedding that is used to output a mesh for each object. |
Ready to Get Started?
We’ve certainly found this codebase to be useful for our 3D computer vision projects, and we hope that you will as well. Contributions to the codebase are welcome and please stay tuned for our own further updates to the framework. To get started please visit our github repository.
Acknowledgements
The release of the TensorFlow 3D codebase and model has been the result of widespread collaboration among Google researchers with feedback and testing from product groups. In particular we want to highlight the core contributions by Alireza Fathi and Rui Huang (work performed while at Google), with special additional thanks to Guangda Lai, Abhijit Kundu, Pei Sun, Thomas Funkhouser, David Ross, Caroline Pantofaru, Johanna Wald, Angela Dai and Matthias Niessner.
