Announcing the release of “Deep Learning with R, 2nd Edition,” a book that shows you how to get started with deep learning in R.
Deep Learning with R, 2nd Edition
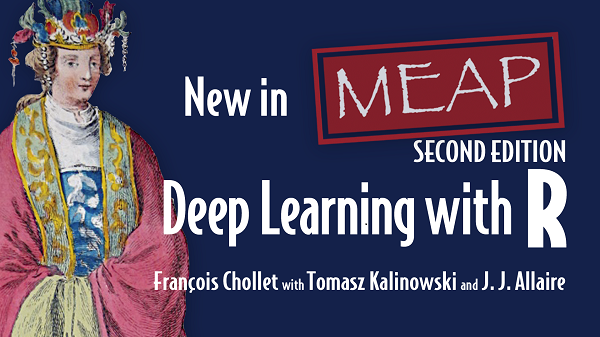
Announcing the release of “Deep Learning with R, 2nd Edition,” a book that shows you how to get started with deep learning in R.
Announcing the release of “Deep Learning with R, 2nd Edition,” a book that shows you how to get started with deep learning in R.
![]() |
submitted by /u/RichardGrant_ [visit reddit] [comments] |
The NVIDIA platform, powered by the A100 Tensor Core GPU, delivers leading performance and versatility for accelerated HPC.
High-performance computing (HPC) has become the essential instrument of scientific discovery.
Whether it is discovering new, life-saving drugs, battling climate change, or creating accurate simulations of our world, these solutions demand an enormous—and rapidly growing—amount of processing power. They are increasingly out of reach of traditional computing approaches.
That is why industry has embraced NVIDIA GPU-accelerated computing. Combined with AI, it is bringing millionfold leaps in performance for scientific advancement. Today, 2,700 applications can benefit from NVIDIA GPU acceleration, and that number continues to rise, backed by a growing community of three million developers.
Delivering the many-fold speedups across the entire breadth of HPC applications takes relentless innovation at every level of the stack. This starts with chips and systems and goes through to the application frameworks themselves.
The NVIDIA platform continues to deliver significant performance improvements each year, with relentless advancements in architecture and across the NVIDIA software stack. Compared to the P100 released just six years ago, the H100 Tensor Core GPU is expected to deliver an estimated 26x higher performance, more than 3x faster than Moore’s Law.
Core to the NVIDIA platform is a feature-rich and high-performance software stack. To facilitate GPU acceleration for the widest range of HPC applications, the platform includes the NVIDIA HPC SDK. The SDK provides unmatched developer flexibility, enabling the creation and porting of GPU-accelerated applications using standard languages, directives, and CUDA.
The power of the NVIDIA HPC SDK lies in a vast suite of highly optimized GPU-accelerated math libraries, enabling you to harness the full performance potential of NVIDIA GPUs. For the best multi-GPU and multi-node performance, the NVIDIA HPC SDK also provides powerful communications libraries:
Altogether, this platform provides the highest performance and flexibility to support the large and growing universe of GPU-accelerated HPC applications.
To showcase how the NVIDIA full-stack innovation translates into the highest performance for accelerated HPC, we compared the performance of a server from HPE with four NVIDIA GPUs with that of a similarly configured server based on an equal number of accelerator modules from another vendor.
We tested a set of five widely used HPC applications using a wide variety of datasets. While the NVIDIA platform accelerates 2,700 applications spanning every industry, the applications we could use in this comparison were limited by the selection of software and application versions that are available for the other vendor’s accelerators.
For all workloads except for NAMD, which is software for molecular dynamics simulation, our results are calculated using the geomean of results across multiple datasets to minimize the influence of outliers and to be representative of customer experiences.
We also tested these applications in multi-GPU and single-GPU scenarios.
In the multi-GPU scenario, with all accelerators in the tested systems being used to run a single simulation, the A100 Tensor Core GPU-based server delivered up to 2.1x higher performance than the alternative offering.
Fueled by continued advances in compute performance, the field of molecular dynamics is moving towards simulating ever-larger systems of atoms for longer periods of simulated time. These advances enable researchers to simulate an increasing set of biochemical mechanisms, such as photosynthetic electron transport and vision signal transduction. These and other processes have long been the subject of scientific debate because they have been beyond the reach of simulation, which is the primary tool for validation. This was due to the prohibitively long amount of time needed to complete the simulations.
However, we recognize that not all users of these applications run them with multiple GPUs per simulation. For optimal throughput, the best execution method is often to assign one GPU per simulation.
When running these same applications on a single accelerator module—a full GPU on the NVIDIA A100 and both compute dies on the alternative product—the NVIDIA A100-based system delivered up to 1.9x faster performance.
Energy costs represent a significant portion of the total cost of ownership (TCO) of data centers and supercomputing centers alike, underscoring the importance of power-efficient computing platforms. Our testing showed that the NVIDIA platform provided up to 2.8x higher throughput-per-watt than the alternative offering.
Efficiency ratio of A100 to MI250 shown – higher is better for NVIDIA. Geomean over multiple datasets (varies) per application. Efficiency is Performance / Power consumption (Watts) as measured for the GPUs using measured using NVIDIA SMI and equivalent functionality in ROCm |
AMD MI250 measured on a GIGABYTE M262-HD5-00 with (2) AMD EPYC 7763 with 4x AMD Instinct MI250 OAM (128 GB HBM2e) 500W GPUs with AMD Infinity Fabric
technology. NVIDIA runs on ProLiant XL645d Gen10 Plus using dual EPYC 7713 CPUs and 4x A100 (80 GB) SXM4
LAMMPS develop_db00b49(AMD) develop_2a35ec2(NVIDIA) datasets ReaxFF/c, Tersoff, Leonard-Jones, SNAP | NAMD 3.0alpha9 dataset STMV_NVE | OpenMM 7.7.0 Ensemble runs for datasets: amber20-stmv, amber20-cellulose, apoa1pme, pme|
GROMACS 2021.1(AMD) 2022(NVIDIA) datasets ADH-Dodec (h-bond), STMV (h-bond) | AMBER 20.xx_rocm_mr_202108(AMD) and 20.12-AT_21.12 (NVIDIA) datasets Cellulose_NVE, STMV_NVE | 1x MI250 has 2x GCD
The excellent performance and power efficiency of the NVIDIA A100 GPU is the result of many years of relentless software-hardware co-optimization to maximize application performance and efficiency. For more information about the NVIDIA Ampere architecture, see the NVIDIA A100 Tensor Core GPU whitepaper.
A100 also presents as a single processor to the operating system, requiring that only one MPI rank be launched to take full advantage of its performance. And, A100 delivers excellent performance at scale thanks to the 600-GB/s NVLink connections between all GPUs in a node.
Just as accelerated computing is bringing many-fold speedups to modeling and simulation applications, the combination of AI and HPC will deliver the next step-function increase in performance to unlock the next wave of scientific discovery.
In the three years between our first MLPerf training submissions and the most recent results, the NVIDIA platform has delivered 20x more deep learning training performance on this industry-standard, peer-reviewed suite of benchmarks. The gains come from a combination of chip, software, and at-scale improvements.
Scientists and researchers are already using the power of AI to deliver dramatic improvements in performance, turbocharging scientific discovery:
Supercomputing centers around the world are continuing to adopt accelerated AI supercomputers.
For more information about the latest performance data, see HPC Application Performance.
The home-buying process can feel like an obstacle course — finding the perfect place, putting together an offer and, the biggest hurdle of all, securing a mortgage. San Francisco-based real-estate technology company Doma is helping prospective homeowners clear that hurdle more quickly with the support of AI. Its machine learning models accelerate properties through the Read article >
The post The Closer: Machine Learning Helps Banks, Buyers Finalize Real Estate Transactions appeared first on NVIDIA Blog.
Learn more about new features and ways to improve system performance using Nsight Compute 2022.2
NVIDIA Nsight Compute is an interactive kernel profiler for CUDA applications. It provides detailed performance metrics and API debugging through a user interface and a command-line tool. Nsight Compute 2022.2 includes features to expand the supported environments and workflows for CUDA kernel profiling and optimization.
The following outlines the feature highlights of Nsight Compute 2022.2.
With the new NVIDIA OptiX acceleration structure viewer, users can inspect the structures they build before launching a ray-tracing pipeline. Acceleration structures describe a rendered scene’s geometries for ray-tracing intersection calculations. Users create these acceleration structures and OptiX translates them to internal data structures. Sometimes the description created by the user is error prone and it can be difficult to understand why the rendered result is not as expected or what is limiting performance.
With this new feature, users can navigate through them in a 3D visualizer and view the parameters used during their creation like build flags, triangle mesh vertices, and AABB coordinates. This viewer is useful to identify overlaps or inefficient hierarchies, resulting in subpar ray-tracing performance.
The latest version adds a new “Issues Detected” column to the summary page for users to sort all profiled kernels by the number of performance issues detected. This gives users guidance on where to focus their efforts across multiple results (kernel profiles). If users are unsure which kernel to focus their optimization efforts on, a long running kernel with a high number of detected issues is a good starting point.
There are improvements to the metric grouping and selection options on the source page to make them easier to use. Additionally, this release adds support for running the Nsight Compute user interface on ARM SBSA and L4T based platforms, for users to profile without needing remote connections or separate host machines for the user interface.
Check out the sessions below released at NVIDIA GTC 2022 showcasing Nsight tool capabilities, support with Jetson Orin, and more.
Google worked with Australia’s national science agency to train ML models that monitor and map harmful coral-eating crown-of-thorns starfish outbreaks along the Great Barrier Reef.
Marine biologists have a new AI tool for monitoring and protecting coral reefs. The project—a collaboration between Google and Australia’s Commonwealth Scientific and Industrial Research Organization (CSIRO)—employs computer vision detection models to pinpoint damaging outbreaks of crown-of-thorns starfish (COTS) through a live camera feed. Keeping a closer eye on reefs helps scientists address growing populations quickly, to protect the valuable Great Barrier Reef ecosystem.
Despite covering less than 1% of the vast ocean floor, coral reefs support about 25% of sea species including fish, invertebrates, and marine mammals. When healthy, these productive marine environments provide commercial and subsistence fishing and income for tourism and recreational businesses. They also protect coastal communities during storm surges and are a rich source of antiviral compounds for drug discovery research.
Assemblages of COTS are found throughout the Indo-Pacific region and feed on coral polyps—the living part of hard coral reefs. They typically occur in low numbers, posing little harm to the ecosystem. However, as outbreaks increase in frequency—in part due to nutrient run-off and a decline in natural predators—they are causing significant damage.
Healthy reefs take about 10 to 20 years to recover from COTS outbreaks, defined by 30 or more adults per 10,000 square meters, or when densities consume coral faster than it can grow. Degraded reefs facing environmental stressors such as climate change, pollution, and destructive fishing practices are less likely to recover, resulting in irreversible damage, diminished coral cover, and biodiversity loss.
Scientists control outbreaks through interventions. Two common approaches involve injecting a starfish with bile salts or removing populations from the water. But, traditional reef surveying, which consists of towing a snorkeler behind a boat for visual identification, is time-consuming, labor-intensive, and less accurate.
According to the project’s TensorFlow post, “CSIRO developed an edge ML platform (built on top of the NVIDIA Jetson AGX Xavier) that can analyze underwater image sequences and map out detections in near real time.” The authors, Megha Malpani an AI/ML product manager at Google, and Ard Oerlemans a Google software engineer, are part of a team of researchers working with CSIRO to build the most accurate and performant models.
Employing an annotated dataset from CSIRO the researchers developed an accurate object detection model that uses a live camera feed rather than a snorkeler to detect the starfish.
It processes images at more than 10 frames per second with precision across a variety of ocean conditions such as lighting, visibility, depth, viewpoint, coral habitat, and the number of COTS present.
According to the post, when a COTS starfish is detected, it is assigned a unique ID tracker, linking detections over time and video frame. “We link detections in subsequent frames to each other by first using optical flow to predict where the starfish will be in the next frame, and then matching detections to predictions based on their Intersection over Union (IoU) score,” Malpani and Oerlemans write.
With the ultimate goal of quickly determining the total number of COTS, the team focused on the entire pipeline’s accuracy. The “current 1080p model using TensorFlow TensorRT runs at 11 FPS on the Jetson AGX Xavier, reaching a sequence-based F2 score of 0.80! We additionally trained a 720p model that runs at 22 FPS on the Jetson module, with a sequence-based F2 score of 0.78,” the researchers write.
According to the study, the project aims to showcase the capability of machine learning and AI technology applications for large-scale surveillance of ocean habitats.
Their work is open source through the crown-of-thorns starfish detection pipeline on GitHub or on the Google Colab. The project is part of Google’s Digital Future Initiative with CSIRO.
Read more. >>
I’m newbie, I’ve working with tensorflow dataset so it’s my first time loading huge external data but there’s a problem, my data set it’s to big for my memory capacity.
I tried wit .flow_from_directory() but it seems to organize the data with classes and classes are the folders inside. This is not the case of my dataset, it’s a train folder -> a lot of folders with random names and inside there is the images, so .flow_from_directory() reads that random name as the label or the class. Is there a way to change that?
I’ve read the tf.data documentation but honestly, I don’t know how to solve my problem yet. I want to load all the data at the same time but it’s too big, so I need help. Please don’t only send me to read the documentation again :(.
submitted by /u/Current_Falcon_3187
[visit reddit] [comments]
Hi guys, my friend recently created a great online course describing how to apply BERT to sentiment analysis using TFX and Vertex AI. We want to reach as many people as possible because we spent several weeks working on it. The course is free, and we’ve also included the entire pipeline and helper library codebase, ready for use as a template in your project.
I’ve tried to share the link with you several times because I think it’s the perfect group (after all, we are showing the possibilities that Tensorflow Extended offers.
Unfortunately, my post gets deleted every time. So, I’m trying again, without the marketing bullshit.
BERT SENTIMENT ANALYSIS ON VERTEX AI USING TFX
Please check out this course and let me know what you think. I hope it will be helpful to some of you. And you think something is missing. Feel free to discuss. We will gladly add the missing parts!
Btw. Any ideas on how to add links to avoid being considered spam?
submitted by /u/Novel_Cryptographer6
[visit reddit] [comments]
The year of the tiger comes into focus this week In the NVIDIA Studio, which welcomes 3D creature artist Massimo Righi. An award-winning 3D artist with two decades of experience in the film industry, Righi has received multiple artist-of-the-month accolades and features in top creative publications.
The post Fantastical 3D Creatures Roar to Life ‘In the NVIDIA Studio’ With Artist Massimo Righi appeared first on NVIDIA Blog.