Posted by Jonathan Mallinson and Aliaksei Severyn, Research Scientists, Google Research
Sequence-to-sequence (seq2seq) models have become a favoured approach for tackling natural language generation tasks, with applications ranging from machine translation to monolingual generation tasks, such as summarization, sentence fusion, text simplification, and machine translation post-editing. However these models appear to be a suboptimal choice for many monolingual tasks, as the desired output text often represents a minor rewrite of the input text. When accomplishing such tasks, seq2seq models are both slower because they generate the output one word at a time (i.e., autoregressively), and wasteful because most of the input tokens are simply copied into the output.
Instead, text-editing models have recently received a surge of interest as they propose to predict edit operations – such as word deletion, insertion, or replacement – that are applied to the input to reconstruct the output. However, previous text-editing approaches have limitations. They are either fast (being non-autoregressive), but not flexible, because they use a limited number of edit operations, or they are flexible, supporting all possible edit operations, but slow (autoregressive). In either case, they have not focused on modeling large structural (syntactic) transformations, for example switching from active voice, “They ate steak for dinner,” to passive, “Steak was eaten for dinner.” Instead, they’ve focused on local transformations, deleting or replacing short phrases. When a large structural transformation needs to occur, they either can’t produce it or insert a large amount of new text, which is slow.
In “FELIX: Flexible Text Editing Through Tagging and Insertion”, we introduce FELIX, a fast and flexible text-editing system that models large structural changes and achieves a 90x speed-up compared to seq2seq approaches whilst achieving impressive results on four monolingual generation tasks. Compared to traditional seq2seq methods, FELIX has the following three key advantages:
- Sample efficiency: Training a high precision text generation model typically requires large amounts of high-quality supervised data. FELIX uses three techniques to minimize the amount of required data: (1) fine-tuning pre-trained checkpoints, (2) a tagging model that learns a small number of edit operations, and (3) a text insertion task that is very similar to the pre-training task.
- Fast inference time: FELIX is fully non-autoregressive, avoiding slow inference times caused by an autoregressive decoder.
- Flexible text editing: FELIX strikes a balance between the complexity of learned edit operations and flexibility in the transformations it models.
In short, FELIX is designed to derive the maximum benefit from self-supervised pre-training, being efficient in low-resource settings, with little training data.
Overview
To achieve the above, FELIX decomposes the text-editing task into two sub-tasks: tagging to decide on the subset of input words and their order in the output text, and insertion, where words that are not present in the input are inserted. The tagging model employs a novel pointer mechanism, which supports structural transformations, while the insertion model is based on a Masked Language Model. Both of these models are non-autoregressive, ensuring the model is fast. A diagram of FELIX can be seen below.
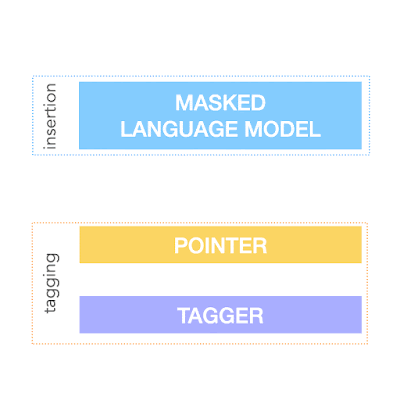 |
An example of FELIX trained on data for a text simplification task. Input words are first tagged as KEEP (K), DELETE (D) or KEEP and INSERT (I). After tagging, the input is reordered. This reordered input is then fed to a masked language model. |
The Tagging Model
The first step in FELIX is the tagging model, which consists of two components. First the tagger determines which words should be kept or deleted and where new words should be inserted. When the tagger predicts an insertion, a special MASK token is added to the output. After tagging, there is a reordering step where the pointer reorders the input to form the output, by which it is able to reuse parts of the input instead of inserting new text. The reordering step supports arbitrary rewrites, which enables modeling large changes. The pointer network is trained such that each word in the input points to the next word as it will appear in the output, as shown below.
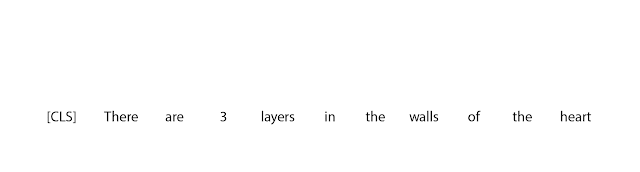 |
Realization of the pointing mechanism to transform “There are 3 layers in the walls of the heart” into “the heart MASK 3 layers”. |
The Insertion Model
The output of the tagging model is the reordered input text with deleted words and MASK tokens predicted by the insertion tag. The insertion model must predict the content of MASK tokens. Because FELIX’s insertion model is very similar to the pretraining objective of BERT, it can take direct advantage of the pre-training, which is particularly advantageous when data is limited.
 |
Example of the insertion model, where the tagger predicts two words will be inserted and the insertion model predicts the content of the MASK tokens. |
Results
We evaluated FELIX on sentence fusion, text simplification, abstractive summarization, and machine translation post-editing. These tasks vary significantly in the types of edits required and dataset sizes under which they operate. Below are the results on the sentence fusion task (i.e., merging two sentences into one), comparing FELIX against a large pre-trained seq2seq model (BERT2BERT) and a text-editing model (LaserTager), under a range of dataset sizes. We see that FELIX outperforms LaserTagger and can be trained on as little as a few hundred training examples. For the full dataset, the autoregressive BERT2BERT outperforms FELIX. However, during inference, this model takes significantly longer.
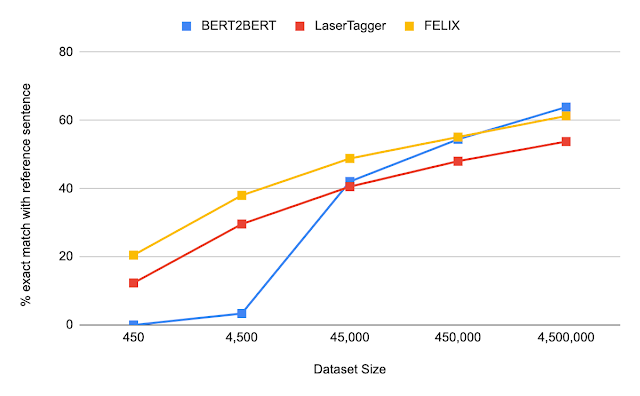 |
A comparison of different training dataset sizes on the DiscoFuse dataset. We compare FELIX (using the best performing model) against BERT2BERT and LaserTagger. |
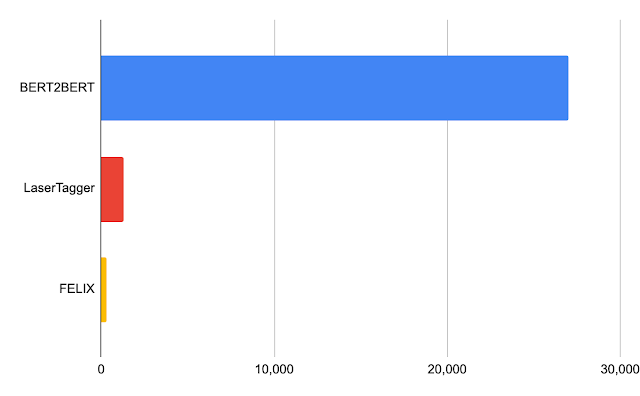 |
Latency in milliseconds for a batch of 32 on a Nvidia Tesla P100. |
Conclusion
We have presented FELIX, which is fully non-autoregressive, providing even faster inference times, while achieving state-of-the-art results. FELIX also minimizes the amount of required training data with three techniques — fine-tuning pre-trained checkpoints, learning a small number of edit operations, and an insertion task that mimics masked language model task from the pre-training. Lastly, FELIX strikes a balance between the complexity of learned edit operations and the percentage of input-output transformations it can handle. We have open-sourced the code for FELIX and hope it will provide researchers with a faster, more efficient, and more flexible text-editing model.
Acknowledgements
This research was conducted by Jonathan Mallinson, Aliaksei Severyn (equal contribution), Eric Malmi, Guillermo Garrido. We would like to thank Aleksandr Chuklin, Daniil Mirylenka, Ryan McDonald, and Sebastian Krause for useful discussions, running early experiments and paper suggestions.
