![]() |
submitted by /u/ZnVjayBhY25l [visit reddit] [comments] |
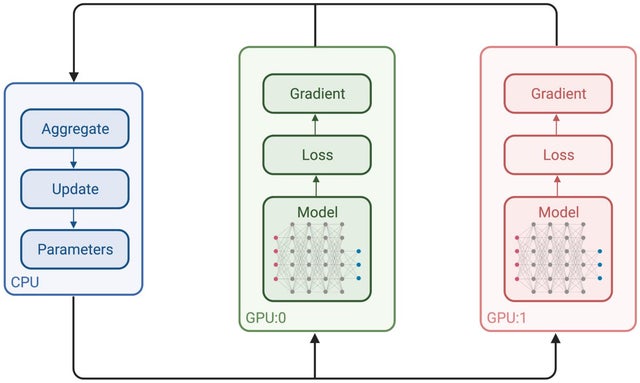
![]() |
submitted by /u/ZnVjayBhY25l [visit reddit] [comments] |
SANTA CLARA, Calif., June 01, 2021 (GLOBE NEWSWIRE) — NVIDIA will present at the following events for the financial community: Evercore ISI Inaugural TMT ConferenceMonday, June 7, at 10:15 …
My company is solving very specific task of semantic segmentation using small UNet and we need to speed up training. Our current workstation has single Tesla v100 and we are looking for new workstation with several A100 but can’t measure how much speed up we will get with the increase of GPU number with new Ampere architecture. The second question is do we need NVSwitch or NVLink for training UNet and what speed improvement it would give us. According to our budget we can possibly get DGX A100(40GB A100) or custom configuration without useless options for our task, for example NVSwitch. The only thing I find is NVidia Unet industrial performance but the evaluation has been done on DGX-1 and DGX A100 with NVLink/NVSwitch both so the impact of GPU interconnection is not obvious.
submitted by /u/gogasius
[visit reddit] [comments]
Situation:
I have a dataset (<class ‘tensorflow.python.data.ops.dataset_ops.MapDataset’>) which is a result of some neural network output. In order to get my final prediction, I am currently iterating over it as follows:
for row in dataset: ap_distance, an_distance = row y_pred.append(int(ap_distance.numpy() > an_distance.numpy()))
The dataset has two columns, each holding a scalar wrapped in a tensor.
Problem:
The loop body is very simple, it takes < 1e-5 seconds to compute. Sadly, one iteration takes ca. 0.3 seconds, so fetching the next row from the dataset seems to take almost 0.3s! This behavior is very weird given my hardware (running on a rented GPU server with 16 AMD EPYC cores and 258GB RAM) and the fact that a colleague on his laptop can finish an iteration in 1-2 orders of magnitude less time than I can. The dataset has ca. 60k rows, hence it is unacceptable to wait for so long.
What I tried:
I tried mapping the above loop body onto every row of the dataset object, but sadly .numpy() is not available inside dataset.map()!
Questions:
submitted by /u/iMrFelix
[visit reddit] [comments]
In January 2020 we released the fly “hemibrain” connectome — an online database providing the morphological structure and synaptic connectivity of roughly half of the brain of a fruit fly (Drosophila melanogaster). This database and its supporting visualization has reframed the way that neural circuits are studied and understood in the fly brain. While the fruit fly brain is small enough to attain a relatively complete map using modern mapping techniques, the insights gained are, at best, only partially informative to understanding the most interesting object in neuroscience — the human brain.
Today, in collaboration with the Lichtman laboratory at Harvard University, we are releasing the “H01” dataset, a 1.4 petabyte rendering of a small sample of human brain tissue, along with a companion paper, “A connectomic study of a petascale fragment of human cerebral cortex.” The H01 sample was imaged at 4nm-resolution by serial section electron microscopy, reconstructed and annotated by automated computational techniques, and analyzed for preliminary insights into the structure of the human cortex. The dataset comprises imaging data that covers roughly one cubic millimeter of brain tissue, and includes tens of thousands of reconstructed neurons, millions of neuron fragments, 130 million annotated synapses, 104 proofread cells, and many additional subcellular annotations and structures — all easily accessible with the Neuroglancer browser interface. H01 is thus far the largest sample of brain tissue imaged and reconstructed in this level of detail, in any species, and the first large-scale study of synaptic connectivity in the human cortex that spans multiple cell types across all layers of the cortex. The primary goals of this project are to produce a novel resource for studying the human brain and to improve and scale the underlying connectomics technologies.
What is the Human Cortex?
The cerebral cortex is the thin surface layer of the brain found in vertebrate animals that has evolved most recently, showing the greatest variation in size among different mammals (it is especially large in humans). Each part of the cerebral cortex is six layered (e.g., L2), with different kinds of nerve cells (e.g., spiny stellate) in each layer. The cerebral cortex plays a crucial role in most higher level cognitive functions, such as thinking, memory, planning, perception, language, and attention. Although there has been some progress in understanding the macroscopic organization of this very complicated tissue, its organization at the level of individual nerve cells and their interconnecting synapses is largely unknown.
Human Brain Connectomics: From Surgical Biopsy to a 3D Database
Mapping the structure of the brain at the resolution of individual synapses requires high-resolution microscopy techniques that can image biochemically stabilized (fixed) tissue. We collaborated with brain surgeons at Massachusetts General Hospital in Boston (MGH) who sometimes remove pieces of normal human cerebral cortex when performing a surgery to cure epilepsy in order to gain access to a site in the deeper brain where an epileptic seizure is being initiated. Patients anonymously donated this tissue, which is normally discarded, to our colleagues in the Lichtman lab. The Harvard researchers cut the tissue into ~5300 individual 30 nanometer sections using an automated tape collecting ultra-microtome, mounted those sections onto silicon wafers, and then imaged the brain tissue at 4 nm resolution in a customized 61-beam parallelized scanning electron microscope for rapid image acquisition.
Imaging the ~5300 physical sections produced 225 million individual 2D images. Our team then computationally stitched and aligned this data to produce a single 3D volume. While the quality of the data was generally excellent, these alignment pipelines had to robustly handle a number of challenges, including imaging artifacts, missing sections, variation in microscope parameters, and physical stretching and compression of the tissue. Once aligned, a multiscale flood-filling network pipeline was applied (using thousands of Google Cloud TPUs) to produce a 3D segmentation of each individual cell in the tissue. Additional machine learning pipelines were applied to identify and characterize 130 million synapses, classify each 3D fragment into various “subcompartments” (e.g., axon, dendrite, or cell body), and identify other structures of interest such as myelin and cilia. Automated reconstruction results were imperfect, so manual efforts were used to “proofread” roughly one hundred cells in the data. Over time, we expect to add additional cells to this verified set through additional manual efforts and further advances in automation.
The H01 volume: roughly one cubic millimeter of human brain tissue captured in 1.4 petabytes of images. |
The imaging data, reconstruction results, and annotations are viewable through an interactive web-based 3D visualization interface, called Neuroglancer, that was originally developed to visualize the fruit fly brain. Neuroglancer is available as open-source software, and widely used in the broader connectomics community. Several new features were introduced to support analysis of the H01 dataset, in particular support for searching for specific neurons in the dataset based on their type or other properties.
![]() |
![]() |
![]() |
||
The volume spans all six cortical layers | Highlighting Layer 2 interneurons | Excitatory and inhibitory incoming synapses |
![]() |
![]() |
![]() |
||
Neuronal subcompartments classified | A Chandelier cell and some of the Pyramidal neurons it inhibits | Blood vessels traced throughout the volume |
![]() |
![]() |
![]() |
||
Serial contact between a pair of neurons | An axon with elaborate whorl structures | A neuron with unusual propensity for self-contact (Credit: Rachael Han) |
The Neuroglancer interface to the H01 volume and annotations. The user can select specific cells on the basis of their layer and type, can view incoming and outgoing synapses for the cell, and much more. (Click on the images above to take you to the Neuroglancer view shown.) |
Analysis of the Human Cortex
In a companion preprint, we show how H01 has already been used to study several interesting aspects of the organization of the human cortex. In particular, new cell types have been discovered, as well as the presence of “outlier” axonal inputs, which establish powerful synaptic connections with target dendrites. While these findings are a promising start, the vastness of the H01 dataset will provide a basis for many years of further study by researchers interested in the human cortex.
In order to accelerate the analysis of H01, we also provide embeddings of the H01 data that were generated by a neural network trained using a variant of the SimCLR self-supervised learning technique. These embeddings provide highly informative representations of local parts of the dataset that can be used to rapidly annotate new structures and develop new ways of clustering and categorizing brain structures according to purely data-driven criteria. We trained these embeddings using Google Cloud TPU pods and then performed inference at roughly four billion data locations spread throughout the volume.
Managing Dataset Size with Improved Compression
H01 is a petabyte-scale dataset, but is only one-millionth the volume of an entire human brain. Serious technical challenges remain in scaling up synapse-level brain mapping to an entire mouse brain (500x bigger than H01), let alone an entire human brain. One of these challenges is data storage: a mouse brain could generate an exabyte worth of data, which is costly to store. To address this, we are today also releasing a paper, “Denoising-based Image Compression for Connectomics”, that details how a machine learning-based denoising strategy can be used to compress data, such as H01, at least 17-fold (dashed line in the figure below), with negligible loss of accuracy in the automated reconstruction.
![]() |
Reconstruction quality of noisy and denoised images as a function of compression rate for JPEG XL (JXL) and AV Image Format (AVIF) codecs. Points and lines show the means, and the shaded area covers ±1 standard deviation around the mean. |
Random variations in the electron microscopy imaging process lead to image noise that is difficult to compress even in principle, as the noise lacks spatial correlations or other structure that could be described with fewer bytes. Therefore we acquired images of the same piece of tissue in both a “fast” acquisition regime (resulting in high amounts of noise) and a “slow” acquisition regime (resulting in low amounts of noise) and then trained a neural network to infer “slow” scans from “fast” scans. Standard image compression codecs were then able to (lossily) compress the “virtual” slow scans with fewer artifacts compared to the raw data. We believe this advance has the potential to significantly mitigate the costs associated with future large scale connectomics projects.
Next Steps
But storage is not the only problem. The sheer size of future data sets will require developing new strategies for researchers to organize and access the rich information inherent in connectomic data. These are challenges that will require new modes of interaction between humans and the brain mapping data that will be forthcoming.
Microsoft Azure has announced the general availability of the ND A100 v4 VM series, their most powerful virtual machines for supercomputer-class AI and HPC workloads, powered by NVIDIA A100 Tensor Core GPUs and NVIDIA HDR InfiniBand. NVIDIA collaborated with Azure to architect this new scale-up and scale-out AI platform, which brings together groundbreaking NVIDIA Ampere Read article >
The post Microsoft Azure Announces General Availability of NVIDIA A100 GPU VMs appeared first on The Official NVIDIA Blog.
The streaming device with the most 4K HDR content available now has even more as Apple TV joins the lineup on NVIDIA SHIELD. Starting today, SHIELD owners can stream the Apple TV app, including Apple TV+, and its impressive lineup of award-winning series, compelling dramas, groundbreaking documentaries, kids’ shows, comedies and more. The Apple TV Read article >
The post App-full: SHIELD TV Now Streaming More Hit Movies and Shows with Apple TV appeared first on The Official NVIDIA Blog.
NVIDIA today announced dozens of new servers certified to run NVIDIA AI Enterprise software, marking a rapid expansion of the NVIDIA-Certified Systems™ program, which has grown to include more than 50 systems from the world’s leading manufacturers.
Several of the world’s top server manufacturers today at COMPUTEX 2021 announced new systems powered by NVIDIA BlueField-2 data processing units. While systems with NVIDIA GPUs are already available now with the option of adding BlueField-2 DPUs, many applications and customer use cases may not require a GPU but can still benefit from a DPU. Read article >
The post NVIDIA Partners Unveil New Servers Featuring NVIDIA BlueField DPUs appeared first on The Official NVIDIA Blog.
Content creators are getting a slew of new tools in their creative arsenal at COMPUTEX with the announcement of new NVIDIA GeForce RTX 3080 Ti and 3070 Ti GPUs. These GPUs deliver massive time savings for freelancers and creatives specializing in video editing, 3D animation or architectural visualization. In addition, HP and Acer announced new Read article >
The post From Hours to Minutes with New Rendering Powerhouses: GeForce RTX 3080 Ti and 3070 Ti appeared first on The Official NVIDIA Blog.