○ TensorRT is an SDK for high-performance deep learning inference, and TensorRT 8.0 introduces support for sparsity that uses sparse tensor cores on NVIDIA Ampere GPUs. It can accelerate networks by reducing the computation of zeros present in GEMM operations in neural networks. You get a performance gain compared to dense networks by just following the steps in this post.
This post was updated July 20, 2021 to reflect NVIDIA TensorRT 8.0 updates.
When deploying a neural network, it’s useful to think about how the network could be made to run faster or take less space. A more efficient network can make better predictions in a limited time budget, react more quickly to unexpected input, or fit into constrained deployment environments.
Sparsity is one optimization technique that holds the promise of meeting these goals. If there are zeros in the network, then you don’t need to store or operate on them. The benefits of sparsity only seem straightforward. There have long been three challenges to realizing the promised gains.
- Acceleration—Fine-grained, unstructured, weight sparsity lacks structure and cannot use the vector and matrix instructions available in efficient hardware to accelerate common network operations. Standard sparse formats are inefficient for all but high sparsities.
- Accuracy—To achieve a useful speedup with fine-grained, unstructured sparsity, the network must be made sparse, which often causes accuracy loss. Alternate pruning methods that attempt to make acceleration easier, such as coarse-grained pruning that removes blocks of weights, channels, or entire layers, can run into accuracy trouble even sooner. This limits the potential performance benefit.
- Workflow—Much of the current research in network pruning serves as useful existence proofs. It has been shown that network A can achieve Sparsity X. The trouble comes when you try to apply Sparsity X to network B. It may not work due to differences in the network, task, optimizer, or any hyperparameter.
In this post, we discuss how the NVIDIA Ampere Architecture addresses these challenges. Today, NVIDIA is releasing TensorRT version 8.0, which introduces support for the Sparse Tensor Cores available on the NVIDIA Ampere Architecture GPUs.
TensorRT is an SDK for high-performance deep learning inference, which includes an optimizer and runtime that minimizes latency and maximizes throughput in production. Using a simple training workflow and deploying with TensorRT 8.0, Sparse Tensor Cores can eliminate unnecessary calculations in neural networks, resulting in over 30% performance/watt gain compared to dense networks.
Sparse Tensor Cores accelerate 2:4 fine-grained structured sparsity
The NVIDIA A100 GPU adds support for fine-grained structured sparsity to its Tensor Cores. Sparse Tensor Cores accelerate a 2:4 sparsity pattern. In each contiguous block of four values, two values must be zero. This naturally leads to a sparsity of 50%, which is fine-grained. There are no vector or block structures pruned together. Such a regular pattern is easy to compress and has a low metadata overhead (Figure 1).
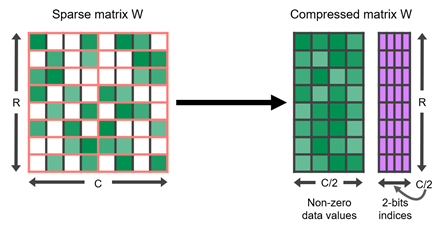
Sparse Tensor Cores accelerate this format by operating only on the nonzero values in the compressed matrix. They use the metadata that is stored with the nonzeros to pull only the necessary values from the other, uncompressed operand. So, for a sparsity of 2x, they can complete the same effective calculation in half the time. Table 1 shows details on the wide variety of data types supported by Sparse Tensor Cores.
Input Operands | Accumulator | Dense TOPS | vs. FFMA | Sparse TOPS | vs. FFMA |
FP32 | FP32 | 19.5 | – | – | – |
TF32 | FP32 | 156 | 8X | 312 | 16X |
FP16 | FP32 | 312 | 16X | 624 | 32X |
BF16 | FP32 | 312 | 16X | 624 | 32X |
FP16 | FP16 | 312 | 16X | 624 | 32X |
INT8 | INT32 | 624 | 32X | 1248 | 64X |
2:4 structured sparse networks maintain accuracy
Of course, performance is pointless without good accuracy. We’ve developed a simple training workflow that can easily generate a 2:4 structured sparse network matching the accuracy of the dense network:
- Start with a dense network. The goal is to start with a known-good model whose weights have converged to give useful results.
- On the dense network, prune the weights to satisfy the 2:4 structured sparsity criteria. Out of every four elements, remove just two.
- Repeat the original training procedure.
This workflow uses one-shot pruning in Step 2. After the pruning stage, the sparsity pattern is fixed. There are many ways to make pruning decisions. Which weights should stay, and which should be forced to zero? We’ve found that a simple answer works well: weight magnitude. We prefer to prune values that are already close to zero.
As you might expect, suddenly turning half of the weights in a network to zero can affect the network’s accuracy. Step 3 recovers that accuracy with enough weight update steps to let the weights converge and a high enough learning rate to let the weights move around sufficiently. This recipe works incredibly well. Across a wide range of networks, it generates a sparse model that maintains the accuracy of the dense network from Step 1.
Table 2 has a sample of FP16 accuracy results that we obtained using this workflow implemented in the PyTorch Library Automatic SParsity (ASP). For more information about the full results for both FP16 and INT8, see the Accelerating Sparse Deep Neural Networks whitepaper.
Network | Data Set | Metric | Dense FP16 | Sparse FP16 |
ResNet-50 | ImageNet | Top-1 | 76.1 | 76.2 |
ResNeXt-101_32x8d | ImageNet | Top-1 | 79.3 | 79.3 |
Xception | ImageNet | Top-1 | 79.2 | 79.2 |
SSD-RN50 | COCO2017 | bbAP | 24.8 | 24.8 |
MaskRCNN-RN50 | COCO2017 | bbAP | 37.9 | 37.9 |
FairSeq Transformer | EN-DE WMT’14 | BLEU | 28.2 | 28.5 |
BERT-Large | SQuAD v1.1 | F1 | 91.9 | 91.9 |
Case study: ResNeXt-101_32x8d
Here’s how easy the workflow is to use with ResNeXt-101_32x8d as a target.
Generating the sparse model
You use the torchvision pretrained model, so step 1 is done already. Because you’re using ASP, the first code change is to import the library:
try: from apex.contrib.sparsity import ASP except ImportError: raise RuntimeError("Failed to import ASP. Please install Apex from https:// github.com/nvidia/apex .")
Load the pretrained model for this training run. Instead of training the dense weights, though, prune the model and prepare the optimizer before the training loop (step 2 of the workflow):
ASP.prune_trained_model(model, optimizer) print("Start training") start_time = time.time() for epoch in range(args.start_epoch, args.epochs): ...
That’s it. The training loop proceeds as normal with the default command augmented to begin with the pretrained model, which reuses the original hyperparameters and optimizer settings for the retraining:
python -m torch.distributed.launch --nproc_per_node=8 --use_env train.py --model resnext101_32x8d --epochs 100 --pretrained True
When training completes (Step 3), the network accuracy should have recovered to match that of the pretrained model, as shown in Table 2. As usual, the best-performing checkpoint may not be from the final epoch.
Preparing for inference
For inference, use TensorRT 8.0 to import the trained model’s sparse checkpoint. The model needs to be converted from the native framework format into the ONNX format before importing into TensorRT. Conversion can be done by following the notebooks in the quickstart/IntroNotebooks GitHub repo.
We have already converted the sparse ResNeXt-101_32x8d to ONNX format. You can download this model from NGC. If you don’t have NGC installed, use the following command to install NGC:
cd /usr/local/bin && wget https://ngc.nvidia.com/downloads/ngccli_cat_linux.zip && unzip ngccli_cat_linux.zip && chmod u+x ngc && rm ngccli_cat_linux.zip ngc.md5 && echo "no-apikeynasciin" | ngc config set
After NGC is installed, download sparse ResNeXt-101_32x8d in ONNX format by running the following command:
ngc registry model download-version nvidia/resnext101_32x8d_dense_onnx:1"
To import the ONNX model into TensorRT, clone the TensorRT repo and set up the Docker environment, as mentioned in the NVIDIA/TensorRT readme.
After you are in the TensorRT root directory, convert the sparse ONNX model to TensorRT engine using trtexec
. Make a directory to store the model and engine:
cd /workspace/TensorRT/ mkdir model
Copy the downloaded ResNext ONNX model to the /workspace/TensorRT/model
directory and then execute the trtexec
command as follows:
./workspace/TensorRT/build/out/trtexec --onnx=/workspace/TensorRT/model/resnext101_32x8d_sparse_fp32.onnx --saveEngine=/workspace/TensorRT/model/resnext101_engine.trt --explicitBatch --sparsity=enable --fp16
A new file named resnext101_engine.trt is created at /workspace/TensorRT/model/. The resnext101_engine.trt file can now be serialized to perform inference by one of the following methods:
- TensorRT runtime in C++ or Python, as shown in this example notebook
- NVIDIA Triton Inference Server
Performance in TensorRT 8.0
Benchmarking this sparse model in TensorRT 8.0 on an A100 GPU at various batch sizes shows two important trends:
- Performance benefits increase with the amount of work that the A100 is doing. Larger batch sizes generally lead to larger improvements, approaching 20% at the high end.
- At smaller batch sizes, where the A100 clock speeds can stay low, using sparsity allows them to be pushed even lower for the same performance, which results in power efficiency improvements greater than the performance itself, leading to up to a 36% performance/watt gain.
Don’t forget, this network has the exact same accuracy as the dense baseline. This extra efficiency and performance doesn’t require penalizing accuracy.
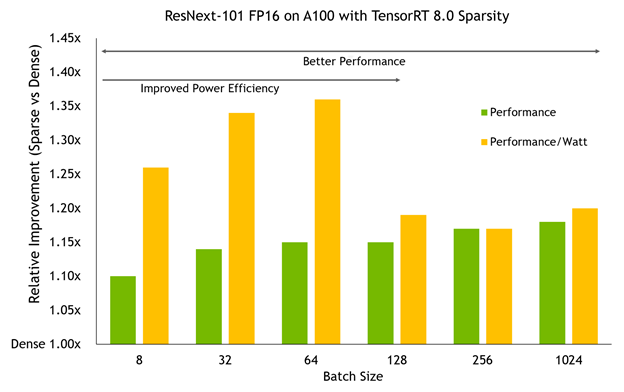
Summary
Sparsity is popular in neural network compression and simplification research. Until now, though, fine-grained sparsity has not delivered on its promise of performance andaccuracy. We developed 2:4 fine-grained structured sparsity and built support directly into NVIDIA Ampere Architecture Sparse Tensor Cores. With this simple, three-step sparse retraining workflow, you can generate sparse neural networks that match the baseline accuracy, and TensorRT 8.0 accelerates them by default.
For more information, see the Making the Most of Structured Sparsity in the NVIDIA Ampere Architecture GTC2021 session, all about accelerating sparsity in the NVIDIA Ampere Architecture, or read the Accelerating Sparse Deep Neural Networks whitepaper.
Ready to jump in and try 2:4 sparsity on your own networks? The Automatic SParsity (ASP) PyTorch library makes it easy to generate a sparse network, and TensorRT 8.0 can deploy them efficiently.
To learn more about TensorRT 8.0 and it’s new features, see the Accelerate Deep Learning Inference with TensorRT 8.0 GTC’21 session or the TensorRT page.