The most important soft skill for ML practitioners and Data Scientists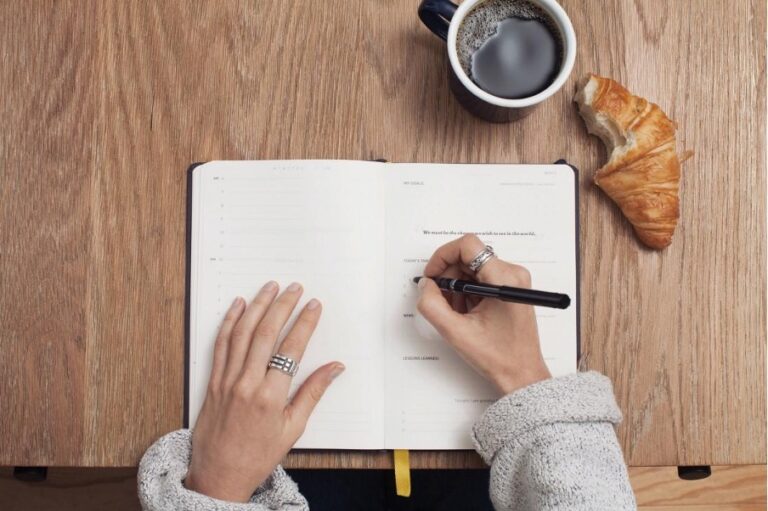
Editor’s Note: If you’re interested sharing your data science and AI expertise, you can apply to write for our blog here.
Data Science as a discipline and profession demands its practitioners possess various skills, ranging from soft skills such as communication, leadership to hard skills such as deductive reasoning, algorithmic thinking, programming, and so on. But there’s a crucial skill that should be attained by Data Scientists, irrespective of their experience, and that is writing.
Even Data Scientists working in technical fields such as quantum computing, or healthcare research need to write. It takes time to develop strong writing ability, and there are challenges that Data Scientists confront that might prevent them from expressing their thoughts easily. That’s why this article contains a variety of writing strategies and explanations of how they benefit Data Science and Machine Learning professionals.
1. Short-form writing
Let’s start with the most typical accessible styles of writing we encounter. Writing in a short form is typically low effort and doesn’t take up too much time. Machine Learning and Data science contents written On Twitter, LinkedIn, Facebook, Quora, and StackOverflow, all fall into this category.
Long-form content, such as books, articles, and essays, is usually the most valuable material in the ML field. All require time to write, read, and analyze. Short-form content on social media platforms, on the other hand, can provide information while using far less effort and time than long form content.
Currently, we have the privilege to witness discourse and ideas shared between AI pioneers and reputable machine learning practitioners, without having to wait for them to write and publish a research paper or an essay. Writing short-form posts on social media platforms provides insight into opinions and views that are not easily expressed verbally and your voice can participate and opinions shared.
For those who want to experiment with connecting with other ML experts through social media postings, I recommend following some people who post genuine and relevant information about Machine learning and Data Science. Take some time to read the tone of the discussions and contributions on posts, and if you have anything valuable to contribute, speak up.
To get you started, here is a list of individuals that post AI-related content (among other interesting things): Andrew Ng, Geoffrey Hinton, Allie, K Miller, Andrej Karpathy, Jeremy Howard, Francois Chollet, Aurélien Geron, Lex Fridman. There are plenty more individuals to follow, but content from these individuals should keep you busy for a while.
Questions/Answer platforms
Questions/Answers as a form of writing has the lowest entry barrier and does not consume as much time, depending on your ability to answer proposed questions.
Given your profession, I’m sure you’ve heard of StackOverflow, the internet’s most popular resource for engineers. When it comes to asking questions on StackOverflow, things aren’t as simple; clarity and transparency are required. Writing queries properly is such an important component of StackOverflow that they’ve published a comprehensive guide on the subject.
Here’s the key takeaway in this section: asking and answering questions on StackOverflow helps you become concise and clear when posing queries, as well as thorough when responding.
2. Emails and Messages
Writing emails and messages is nothing specific to machine learning but Data Scientists and Machine-Learning practitioners that practice the art of composing effective messages tend to flourish within corporations and teams for obvious reasons, some of which are the ability to contribute, network, and get things done.
Composing well-written messages and emails can land you a new role, get your project funded or get you into an academic institution. Purvanshi Mehta wrote an article that explores the effective methods of cold messaging individuals on LinkedIn to build networks. Purvanshi article is a step-by-step instruction on adoptable cold messaging etiquette.
3. Blogs and Articles
Many experts believe that blogs and articles have a unique role in the machine learning community. Articles are how professionals stay up to date on software releases, learn new methods, and communicate ideas.
Technical and non-technical ML articles are the two most frequent sorts of articles you’ll encounter. Technical articles are composed of descriptive text coupled with code snippets or gists that describe the implementation of particular features. Non-technical articles include more descriptive language and pictures to illustrate ideas and concepts.
4. Newsletters
Starting and maintaining a newsletter might not be for Data scientists, but this sort of writing has shown to provide professional and financial advantages to those who are willing to put in the effort.
A newsletter is a key strategic play for DS/ML professionals to increase awareness and presence in the AI sector. A newsletter’s writing style is not defined, so you may write it however so you choose. You might start a formal, lengthy, and serious newsletter or a short, informative, and funny one.
The lesson to be drawn from this is that creating a newsletter may help you develop a personal brand in your field, business, or organization. Those who like what you do will continue to consume and promote your material.
There are a thousand reasons why you should not start a newsletter today, but to spark some inspiration, below are some ideas you can base your newsletter on, and I’ve also included some AI newsletters you should subscribe to.
Newsletter Ideas related to AI:
- A collection of AI/ML videos to watch, with your input on each video.
- A collection of AI/ML articles to read.
- Job postings in your areas that job seekers might be interested in.
- Up-to-date relevant AI news for ML practitioners interested in the more practical application of AI.
Remember that the frequency, length, and content of your newsletter are all defined by you. You could start a monthly newsletter if you feel you don’t have much time or a daily newsletter to churn out content like a machine.
Machine Learning and Data Science Newsletter to subscribe to:
5. Documentation
Documentation, both technical and non-technical, is a common activity among software engineering occupations. Data Scientists are not exempt from the norm, and documentation that explains software code or individual features is recommended and considered best practice.
When is a project successful? Some might consider that it’s when your model achieves an acceptable accuracy on a test dataset?
Experienced Data Scientists understand that project success is influenced by a number of variables, including software maintainability, longevity, and knowledge transfer. Software documentation is a task that can improve the prospects of a project beyond the capabilities of a single team member not to mention, it provides an extra layer of software quality and maintainability.
One of the main advantages of documentation that Data Scientists should be aware of is its role in reducing queries concerning source code from new project members or novice Data Analysts. The majority of questions about source code are concerned with file locations, coding standards and best practices. This data can all be recorded once and referenced by many individuals.
Here are some ideas of items you could document
- Code Documentation: It’s critical to standardize implementation style and format in order to guarantee uniformity across applications. This conformity makes the transition for new developers into a codebase easier since coding standards are given through code documentation.
- Research and Analysis: Given the importance of software product features, successful development is always dependent on thorough study and analysis. Any ML expert who has worked on a project at the start will have handled the plethora of feature requests from stakeholders. Documenting information surrounding feature requests enables other parties involved in the project to get a more straightforward overview of the requirement and usefulness of the proposed feature. It also enforces the feature requester to conduct better research and analysis.
- Database Configurations / Application Information: Documenting information particular to applications, such as configuration parameters and environment variables, is critical for any software team, especially if you move to a new job or company.
- How-tos: Installation of software libraries and packages may be difficult, but the fact is that there could be different installation processes for various operating systems or even versions. It’s not uncommon to discover missing dependencies in official library documentation and quirks you must go through to install the program.
- API Documentation: When teams develop internal and external APIs (Application Programming Interfaces), they should document the components of methods, functions, and data resources needed by those APIs. There’s nothing more annoying than working with a non-documented API; the whole process becomes a guessing game, and you’ll spend time researching the parameters, internal workings, and outputs of an undocumented API. Save your team and clients time by creating a smooth experience when consuming the technical resources you make.
There’s no question that extensive resources allow organizations to conduct many types of documentation, and some even hire technical writers. Although those are all viable options, it is critical for machine learning experts who wish to take software completeness seriously to practice documenting programs and software developed in order to promote the idea that they can provide thorough explanations.
A quick Google search on “how to write good software documentation” provided good resources that all shared the same messages and best practices on documentation.
6. Research Papers
In 2020, I published an article on how to read research papers, which became a huge hit. When it comes to utilizing ML algorithms and models, we have to optimize the way we read these papers in much the same way that seasoned machine-learning experts do.
Writing machine-learning research papers is the other side of the coin. I’ve never written a research paper, and I don’t intend to start now. However, some Machine-learning specialties are very concerned with writing and publishing research studies. As a metric of career success, research institutions and firms use the number of papers published by an individual or group.
There’s an art to writing research papers; researchers and scientists must think about the structure and content of the data to ensure that a message, breakthrough, or idea is delivered effectively. Most of us are probably not writing research papers anytime soon, but there’s value in adopting the practice of writing good research papers. For example, having an abstract, introduction, and conclusion is a writing structure transferable to other writing pieces.
Go ahead and read some research papers; take note of the language, structure and use of visual images the authors are using. Try and adopt any good practice you identify in your next written piece.
7. Books and E-books
There’s no doubt that ML/DS books are the most authoritative texts on machine learning theory and hands-on expertise. I’m not suggesting that all data scientists and ML engineers should write a book. But bear with me.
I looked through several of the authors on my shelf who wrote books in AI/ML, and they all have extensive experience in their fields.
Writing non-fiction, technical books about machine learning is very difficult. It requires a high level of theoretical and practical industry knowledge that can only be attained through total immersion in study, research, and implementation. To educate hundreds of ML Engineers and Data Scientists, your reputation must be based on solid academic, commercial, or research credentials. Not to mention that writers require creativity when delivering well-written books. More specifically, they have to master the art of conveying sophisticated topics in books.
My argument is that to create a timeless machine learning book, you must go down the road of expertise. This does not sound inviting, but I’d want you to consider the fact that setting a long-term objective of writing a book will push you to delve more into the subject of machine intelligence or chosen field, which will enhance your general understanding of AI.
Books for Data Scientist and Machine Learning practitioners:
You will find that most authors listed preceding have produced the majority if not all forms of writing listed in this article, regardless of their domain specialty, hence why I consider writing a vital skill for Machine Learning practitioners and Data Scientists to master.
Conclusion
Whenever I’m asked what life decision provided me with the most benefit, either financial, academic or career, I usually answer with my decision to write.
Throughout this post, you’ve seen several advantages Data Scientists and Machine Learning experts may obtain if they write AI-related material on a regular basis. This section centralizes all the benefits listed throughout this article to make sure it all hits home.
- ML professionals employ writing to communicate complicated subjects in a simple way. By reading a well-written blog post by Andrej Karpathy, I was able to acquire a greater appreciation for the practical application of convolutional neural networks.
- Various types of writing can help you improve your creativity and critical thinking. I recently read AI 2041 by Kai-Fu Lee and Chen Qiufan, in which the authors examine AI technologies and their effects on human lives through well-written fictional stories and thorough explanations of AI technologies. Both writers have written for many years and have authored other books. It’s reasonable to conclude that their writing abilities allowed the writers to express future situations involving AI technology and explore the unknown societal impact of AI integration through critical and logical predictions based on current AI development.
- Writing in the form of storytelling gives life to projects. Good stories are spoken, but great stories are written. The retelling of machine-learning projects to stakeholders such as customers, investors, or project managers takes a positive and exciting turn when coupled with the art of storytelling. A Data Scientist explaining to stakeholders why a new state-of-the-art cancer detection deep-learning model should be leveraged across federal hospitals becomes more impactful and relatable when coupled with the story of an early diagnosis of a patient.
- Within the machine learning community, writing is a successful method of knowledge transfer. Most of the information you’ll get in the DS/ML world will be through written content. Articles, essays, and research papers are all repositories of years worth of knowledge organized into succinct chapters with clear explanations and digestible formats. Writing is an efficient way to condense years of knowledge and experience.
Did you know that AI pioneers and experts we admire and learn from also publish regularly? In this article, I compile a shortlist of individuals in the AI field and provide samples of their work, emphasizing the value and consequence of their work.
Thanks for reading.