NVIDIA is accelerating the field of genomics and drug discovery with the help of GPUs. We sit down with the lab lead to learn more about their work.
The following post provides a deep dive into some of the accomplishments and current focus of drug discovery and genomics work by NVIDIA. A leader in innovations within healthcare and life sciences, NVIDIA is looking to add AI, deep learning, simulation, and drug discovery researchers and engineers to the team. If what you read aligns with your career goals please review the current job postings.
NVIDIA is tapping into the latest technology as it pairs high-performance computing (HPC) with genome and drug discovery research. As genomic testing becomes more mainstream, the amount of data that requires analysis has increased. Drug discovery has also entered a new era of research, as AI and deep learning open the door to discovering thousands of new compounds that serve as the base of drug discovery.
NVIDIA researchers and engineers, like group-lead Johnny Israeli, are supercharging genomics and drug discovery research. Developing software like NVIDIA Clara Parabricks, which is a GPU-accelerated computational genomics application framework that delivers end-to-end analysis workflows for whole genomes, exomes, cancer genomes, and RNA sequencing data. Leading NVIDIA Research content marketing, I sat down with Johnny to learn more about what he does with his group.
Nathan Horrocks
Hey Johnny, it’s great to finally connect with you. Let’s jump right in. I wanted to ask, given that NVIDIA is a tech company, how does working in your group here differ from working at a biotech company?
Johnny Israeli
Hey Nate, thank you for reaching out. There are a couple of ways to think about the differences. Oftentimes in biotech, there is a very specific technology goal or problem. You use whichever technology, or combination of technologies, to solve that problem. You may be married to the problem or the goal, but not so married to the type of technology you might use. Here we pursue products that leverage our expertise in accelerated computing and AI technologies and have more flexibility in terms of our goals for our products.
For example, a few years ago we worked on genomics, but we didn’t build any kind of product for drug discovery. Today, we are building a product for that specific area. The reason for that is that drug discovery as a field is changing. We see an opportunity for us to pursue a new goal. So I would say we have a track record of chasing new opportunities as they become available to our unique positioning and our unique skill set.
Nathan Horrocks
Could you give me an example of a unique opportunity that differentiates you from a traditional biotech company?
Johnny Israeli
I would highlight the intensity of our AI-oriented work in drug discovery. Quite a few companies in the drug discovery space work with AI but the level and focus of investment may be different. For biotech companies, AI is one of several technology options in a broader technology arsenal to pursue drug discovery programs.
At NVIDIA, we know that we are uniquely positioned to do a great job with AI and accelerated computing. So we’re incentivized to invest in this work with greater intensity and focus than most other companies can, both because of our positioning and because of our scale. So engineers and scientists interested in the intersection of AI and drug discovery, and parallel computing would find our areas of work interesting.
Nathan Horrocks
You mentioned your work on genomics, could you tell me how your past work in genomics is impacting your current work in drug discovery?
Johnny Israeli
The drug discovery space is multidisciplinary and it’s a long and complicated process. At the very early stage of the drug discovery process is the stage of target identification. Most of the drug discovery work out there is what’s called target-based drug discovery workflows, where you figure out what is the target, the protein target to go after, and then develop a drug.
Our genomics work contributes greatly to the target identification problem. You can build these genome-wide data sets across many individuals and then analyze them to figure out which mutations are associated with different kinds of diseases. By identifying these mutations and analyzing them, we can then figure out protein targets that are relevant for a given disease. And then build out the rest of the drug discovery workflow from there.
So we use our software called NVIDIA Clara Parabricks to map data from genomics instruments, identify genomic variants, and annotate them. By simplifying these genomics workflows into push-button software solutions and accelerating that software, we are reducing the time and cost to generate large-scale genomics datasets. These large-scale genomics datasets across many individuals are then used to identify protein targets that can impact disease outcomes, and the structures of those proteins are used with our NVIDIA Clara Discovery software to generate and simulate drug compounds and their interaction with those proteins.
Nathan Horrocks
So you’re using Clara Parabricks to fuel protein identification in genomics and then using Clara Discovery to simulate compounds that could potentially be used as a drug?
Johnny Israeli
Exactly, in the context of drug discovery, we help figure out the most promising compounds for a given drug discovery program, and this is something we are really excited about. We started around a year and a half ago looking into drug discovery. We announced at GTC– in the fall of 2020 I believe– that we were going to build this software called NVIDIA Clara Discovery. That it would be an NVIDIA framework for all things pertaining to computational drug discovery. And that’s where there is all this cutting-edge work happening, and where we are actually looking to hire at the moment.
Nathan Horrocks
Do you want to dive into that? If we’re looking for engineers and researchers in this area, they might find it interesting to know more about what work you are focusing on.
Johnny Israeli
Absolutely, yeah. Drug discovery is a long, complicated process involving multiple disciplines. When you think about computational drug discovery, there are three dynamics taking place that could reshape the industry from a computational standpoint. Those three dynamics are what you are trying to do at the core of the computational drug discovery loop. You have a protein –a target– that you want to impact, you have a compound, which is potentially a drug to be developed. Then given a compound and the protein structure you can do all kinds of simulations. You are trying to predict if it would be a useful interaction.
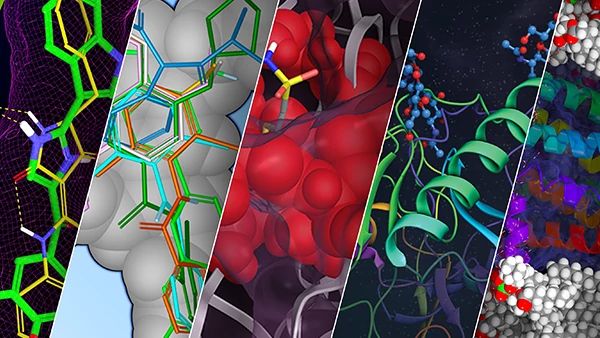
Traditionally you would have a database of these compounds. All kinds of companies are cataloging and producing these databases, and there are billions of compounds today. Then you have the world of protein structures, which is produced by a whole bunch of groups doing structural biology work.
Now, three things are happening that we think could reshape everything. First is the breakthrough work by DeepMind and other groups in the form of AlphaFold and so on. We’re now using deep learning to predict protein structure. So if that’s true, we’re going to have many more protein structures to work within the coming years than we have had up to this point. That is dynamic number one.
Dynamic number two is through our work here in Clara Discovery, and also others in the industry, we are building the capability to generate compounds. Imagine using deep learning—not so different from StyleGAN and Gaugan—that can generate a seemingly infinite number of generated images. Turns out you can generate all kinds of compounds as well. We have software with a graphical user interface where you click and compounds come out. So that means in the coming years as this capability matures, we’re going to have a million X more compounds than before. Before we had a billion and in another few years, we’ll have a million billion compounds to work with. And that’s still scratching the surface because the number of potential compounds out there in the universe of such compounds could be 10 to the 60. That’s dynamic number two.
So the first dynamic is happening at large within the industry and NVIDIA is enabling it. For dynamic number two, we’re building a product for that. We have Clara Discovery and we have a specific workflow and a technology we are using called MegaMolBART.
MegaMolBART is the adoption of Megatron, which was initially developed for natural language processing (NLP) at scale, and we repurposed Megatron for the language of chemistry because there is a way to represent molecules using a string format. So you can repurpose all this NLP technology, and the same technology that is bringing Megatron to market is the same technology powering this part of our drug discovery work. It’s the same piece of software called NeMoMegatron.
Dynamic number three is if you have a million more compounds than before, and you have tens more protein structures than before, then the combination that you want to simulate is millions more than ever before.
Now, simulation, as we know it computationally, can be a very intensive problem. In fact, one of the early use cases of CUDA was in molecular dynamics and scientific computing in this kind of simulation. But the question is how, how do you enable a million X more of it? We are building a team to figure out that simulation capability and we are hiring experts in molecular dynamics, force field development, high-performance computing, and deep learning applications to simulation. We are also hiring cheminformatics experts, deep learning researchers, and engineers to advance our technologies for compound generation and interaction with proteins using AI.
And I think that captures what we do here. It is a unique group, in that we push products out and also have scope for product-driven research. We work extensively with engineering groups across the company to leverage technologies that can advance these products, and we collaborate with a variety of research groups to leverage AI breakthroughs across the company.
Nathan Horrocks
Would you expand on what you just said? What do you mean it differs from other NVIDIA research areas?
Johnny Israeli
I would say most research labs have more flexibility than we have in terms of the kind of research we’re pursuing. Our organization keeps a healthy balance between engineering and research so that we can ship products but also have the bandwidth to pursue innovative opportunities. But that does mean that our research goals or research agenda may be somewhat constrained by the objectives of the product in a way that the typical research lab might not be constrained. In a typical academic lab or even an industry research group, I would expect more flexibility, but it’s a tradeoff. It’s a tradeoff between flexibility and the intense focus that is needed to ship a software product.
Nathan Horrocks
That’s what I was going to ask. What value is there for a researcher then to want to join your group?
Johnny Israeli
Great question. I would say we tend to attract researchers who are interested in innovative research and are passionate about making sure that their research has a business impact. And for those individuals this tradeoff makes sense. They are willing to constrain and focus the research as needed to have that kind of business impact that they desire.
Nathan Horrocks
So their research is more focused on improving Clara Discovery and Clara Megamolbart?
Johnny Israeli
That’s correct. So we need to align the research activities with the product goals.
Nathan Horrocks
You’ve mentioned that the larger portion of your work involves engineers, how knowledgeable do you think these engineers need to be in biotechnology?
Johnny Israeli
A great question. I find that many up from the engineering background learn this on the job. What’s more important is not so much the knowledge of the industry, but genuine interest. We have multiple examples here of engineers who may be studied some of this stuff in college, or they just read about some of the stuff and they have the right engineering background.
And you know, a year or two years in they know their industry really well because they work with our partners and collaborators. So I would say interest matters most.
Nathan Horrocks
I remember you mentioning at the beginning anyone interested in the intersection of AI, simulation, and drug discovery would find this work interesting.
Johnny Israeli
Exactly. This is exciting and incredibly challenging work, and we are just scratching the surface. I am looking forward to what the next few years will bring as we dive deeper into NVIDIA Clara and its potential to contribute to the biotech community.
Additional Resources
If you are interested in learning more about NVIDIA Genomics, check out our Genomics page.
To stay informed about new research being done at NVIDIA, visit NVIDIA Research.