By developing applications using MPI and standard C++ language features, it is possible to program for GPUs without sacrificing portability or performance.
This is the third post in the Standard Parallel Programming series, about the advantages of using parallelism in standard languages for accelerated computing.
- Developing Accelerated Code with Standard Language Parallelism
- Multi-GPU Programming with Standard Parallel C++, Part 1
In part 1, we explained:
- The basics of C++ parallel programming
- The lattice Boltzmann method (LBM)
- Took the first steps towards refactoring the Palabos library to run efficiently on GPUs using standard C++.
In this post, we continue by optimizing the performance of the ISO C++ algorithms and then use MPI to scale the application across multiple GPUs.
Strive for optimal performance
It may seem natural to expect that the performance of your CPU-to-GPU port will range below that of a dedicated HPC code. After all, you are limited by the constraints of the software architecture, the established API, and the need to account for sophisticated extra features expected by the user base. Not only that, the simplistic programming model of C++ standard parallelism allows for less manual fine-tuning than a dedicated language like CUDA.
In reality, it is often possible to control and limit this loss of performance to an extent that it becomes negligible. The key is to analyze the performance metrics of individual code portions and eliminate performance bottlenecks that do not reflect an actual need of the software framework.
A good practice consists in maintaining a separate proof-of-principle code for core components of your numerical algorithm. The performance of this approach can be more freely optimized and compared with the one of the full, complex software frameworks (like the STLBM library in the case of Palabos). Additionally, a GPU-capable profiler like nvprof
can highlight the origin of performance bottlenecks efficiently.
Typical performance issues and their solutions are highlighted in the following recommendations:
- Do not touch data on the CPU
- Know your algorithms
- Establish a performance model
Do not touch data on the CPU
A frequent source of performance losses are hidden data transfers between CPU and GPU memory, which can be exceedingly slow. With the CUDA unified memory model, this type of transfer occurs whenever you access GPU data from the CPU. Touching a single byte of data can result in a catastrophic performance loss because entire memory pages are transferred at one time.
The obvious solution is to manipulate your data exclusively on the GPU whenever possible. This requires searching your code carefully for all accesses to the data and then wrapping them into a parallel algorithm call. Although this is somewhat robust, this process is needed for even the simplest operations.
Obvious places to look for are post-processing operations or intermediate evaluations of data statistics. Another classical performance bottleneck is found in the MPI communication layer, because you must remember to carry out data packing and unpacking operations on the GPU.
Expressing an algorithm on GPU is easier said than done, as the formalism of for_each
and transform_reduce
is mostly suitable for evenly structured memory accesses.
In the case of irregular data structures, it would be painful to avoid race conditions and guarantee coalesced memory accesses with these two algorithms. In such a case, you should follow up with the next recommendation and familiarize yourself with the family of parallelized algorithms provided in the C++ STL.
Know your algorithms
Up to this point, the parallel STL appears as little more than a way to express parallel for loops
with a fancy functional syntax. In reality, the STL offers a large set of algorithms beyond for_each
and transform_reduce
that are useful to express your numerical method, including sorting and searching algorithms.
The exclusive_scan
algorithm computes cumulative sums and deserves particular mention, as it proves generally useful for reindexing operations of unstructured data. For example, consider a packing algorithm for MPI communication, in which the number of variables contributed to the communication buffer by every grid node is unknown in advance. In this case, global communication among threads is required to determine the index at which every grid node writes into the buffer.
The following code example shows how to solve this type of problem with good parallel efficiency on GPU using parallel algorithms:
// Step 1: compute the number of variables contributed by every node. int* numValuesPtr = allocateMemory(numberOfCells); for_each(execution::par_unseq, numValuesPtr, numValuesPtrl + numberOfCells, [=](int& numValues) { int i = &numValues - numValuesPtr; // Compute number of variables contributed by current node. numValues = computeNumValues(i); } ); // 2. Compute the buffer index for every node. int* indexPtr = allocateMemory(numberOfCells); exclusive_scan(execution::par_unseq, numValuesPtr, numValuesPtr + numberOfCells, indexPtr, 0); // 3. Pack the data into the buffer. for_each(execution::par_unseq, indexPtr, indexPtr + numberOfCells, [=](int& index) { int i = &index - indexPtr; packCellData(i, index); } );
This example lets you enjoy the expressive power of the algorithm-based approach to GPU programming: the code requires no synchronization directives or any other low-level constructs.
Establish a performance model
A performance model establishes an upper bound for the performance of your algorithm through a bottleneck analysis. This typically considers the peak processor performance (measured in FLOPS) and the peak memory bandwidth as the principal limiting hardware characteristics.
As discussed in the Example: Lattice Boltzmann software Palabos section in the previous post, LBM codes have a low ratio of computations to memory accesses and are entirely memory-bound on modern GPUs. That is, at least if you use single-precision arithmetics or a GPU that is optimized for double-precision arithmetics.
The peak performance is simply expressed as a ratio between the memory bandwidth of the GPU and the number of memory accesses performed in the code. As a direct consequence, switching an LBM code from double– to single-precision arithmetics doubles the performance.
Figure 1 shows the performance of the GPU port of Palabos obtained on an NVIDIA A100 (40 GB) GPU for single– and double-precision floats.
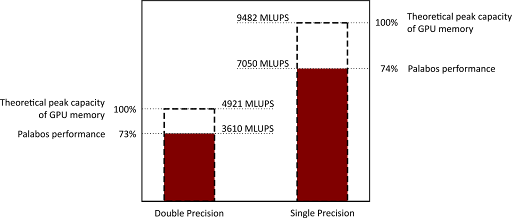
The executed test case, a flow in a lid-driven cavity in a turbulent regime, has a simple cubic geometry. However, this case includes boundary conditions and exhibits a complex flow pattern. The performance is measured in million lattice-node updates per second (MLUPS, more is better) and compared against a theoretical peak value obtained under the assumption that GPU memory is exploited at peak capacity.
The code reaches 73% of peak performance in double-precision and 74% in single-precision. Such performance metrics are common in state-of-the-art implementations of LB models, independently of the language or library used.
Although some implementations may gain a few percentage points and reach a value closer to 80%, it is clear that we are closing in on the hard limit implied by the performance model. From a big-picture standpoint, the single-GPU performance of the code is as good as it gets.
Reuse the existing MPI backend to get a multi-GPU code
As C++ parallel algorithms integrate into an existing software project seamlessly to accelerate critical code portions, nothing prevents you from reusing the project’s communication backend to reach multi-GPU performance. However, you will want to keep an eye on the communication buffer and make sure that it does not take any detours through CPU memory, which would result in costly page faults.
Our first attempt to run the GPU-ported version of Palabos on multiple GPUs, although producing technically correct results, did not exhibit acceptable performance. Instead of a speedup, the switch from one to two GPUs delivered a decrease in speed by an order of magnitude. The issue could be traced to the packing and unpacking of the communicated data. In the original backend, this was carried out on CPUs, and to other instances of unnecessary data access in CPU memory, such as resizing of the communication buffer.
Such issues can be spotted with help of the profiler. The profiler highlights all occurrences of page faults in unified memory, and are fixed by moving the corresponding code portions to a parallel algorithm. The Know your algorithms section explained how to pack and unpack the communication buffer if the data follows an irregular pattern.
At this point, using standard C++ without any extensions other than MPI, you can get a hybrid CPU/GPU software project with state-of-the-art performance on single-GPU and solid parallel performance on multi-GPU.
Unfortunately, the multi-GPU performance remains below the expectations due to the current limitations of the language specifications and corresponding GPU implementations. Pending future improvements to the fairly young technology of C++ standard parallelism, we provide some workarounds in this post based on techniques outside the C++ standard.
Coordinate the multi-CPU and multi-GPU code execution
While this post focuses on hybrid CPU and GPU programming, we can’t avoid addressing the issue of hybrid parallelism (MPI or multithreading) in the CPU parts at some point.
The original version of Palabos, for example, is non-hybrid and uses the MPI communication layer to distribute work among the cores of a CPU as well as across the network. After porting to GPU, the resulting multi-CPU and multi-GPU code spontaneously groups a single CPU core with a full GPU in every MPI task, leaving the CPU relatively underpowered.
This leads to a performance bottleneck whenever it is necessary or convenient to keep a computationally intensive task on the CPU. In fluid dynamics, this is often the case in the preprocessing stage, such as in geometry processing or mesh generation.
The obvious solution involves the use of multithreading to access multiple CPU cores from within an MPI task. The shared memory space of these threads can then be directly shared with the GPU through the CUDA unified memory formalism.
However, C++ parallel algorithms cannot be reused to serve both purposes of GPU and multi-core CPU execution. This is because C++ does not allow choosing the target platform of parallel algorithms from within the language.
While C++ threads do provide a way to solve this problem natively, we found that OpenMP offered the most convenient and least intrusive solution. An OpenMP annotation of a for loop
was sufficient in this case to distribute the grid portions assigned to the current MPI task over multiple threads.
Communicate through pinned memory
With current versions of the HPC SDK, the CUDA unified memory model exhibits another performance issue in combination with MPI.
As the MPI communication layer expects data with a fixed hardware address (so-called pinned memory
), any buffer that resides in the managed memory area is first copied into a pinned memory buffer on the host CPU implicitly. Due to the transfers between the GPU and CPU, this operation can end up being rather costly.
Communication buffers should therefore be explicitly pinned to a GPU memory address. With the nvc++ compiler
, this is achieved by allocating the communication buffer with cudaMalloc
:
// Allocate the communication buffer // vector buffer(N); // double* buffer = buffer.data(); double* buffer; cudaMalloc((void**)&buffer, N * sizeof(double)); for_each(buffer, buffer + N, … // Proceed with data packing
Another solution is to replace the STL vector with a thrust::device_vector
from the Thrust library, which uses pinned GPU memory by default.
In the near future, the HPC SDK will handle these cases more efficiently and automatically for users. This is so that they do not have to reach for cudaMalloc
or thrust::device_vector
. So, stay tuned!
After the various improvements listed in this post, the Palabos library was tested on a DGX A100 (40-GB) workstation with four GPUs, again for the benchmark case of a lid-driven cavity. The obtained performance is shown in Figure 2 and compared to the performance achieved on a 48-core Xeon Gold 6240R CPU:
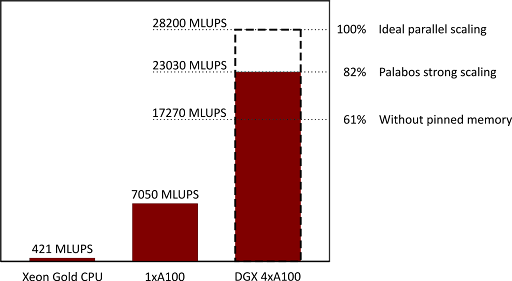
For the Xeon Gold, the original implementation of Palabos proved to be more efficient and was used with 48 MPI tasks, while the single-GPU and the four-GPU execution used the parallel algorithms backend, compiled with nvc++
.
The performance figures show a 3.27-fold speedup of the 4-GPU execution compared to the single-GPU one. This amounts to a quite satisfactory parallel efficiency of 82% in a strong scaling regime, with equal total domain size in both executions. In weak scaling, using a 4x larger problem size for the four-GPU execution, the speedup increases to 3.72 (93% efficiency).
Figure 2 also shows that when using an unpinned communication buffer, such as when the MPI communication buffer is not allocated with cudaMalloc
, the parallel efficiency drops from 82% to 61%.
In the end, the four-GPU DGX workstation runs 55x faster than the Xeon Gold CPU. While the direct comparison may not be fair due to the different scope of the two machines, it provides a sense of the acceleration obtained by porting a code to GPU. The DGX is a desktop workstation connected to a common power plug, yet it delivers a performance that, on a CPU cluster, could only be obtained with thousands of CPU cores.
Conclusion
You’ve seen that C++ standard language parallelism can be used to port a library like Palabos to GPU with an astounding increase in the code’s performance.
- For end users of the Palabos library, this performance gain is obtained with a single-line change to switch from the CPU to the GPU backend.
- For Palabos library developers, some work was required to develop the corresponding GPU backend.
However, this work didn’t require learning a new domain-specific language nor did it depend on a detailed knowledge of the GPU architecture.
This two-part post has provided you with guidelines that you can apply to achieve similar results with your own code. For more information, we encourage you to check out the following resources:
- Learn more about the compiler support on the HPC SDK page.
- Read the Developing Accelerated Code with Standard Language Parallelism post
- Download the HPC SDK for free
- Learn to use C++ standard parallelism with MPI by reading the code for the 2D heat equation.
- Learn to implement LBM on GPUs through a simple, self-contained example or by downloading the full STLBM library.
- Visit the project page of the Palabos GPU port
- Download Palabos