Posted by Sharan Narang and Aakanksha Chowdhery, Software Engineers, Google Research
In recent years, large neural networks trained for language understanding and generation have achieved impressive results across a wide range of tasks. GPT-3 first showed that large language models (LLMs) can be used for few-shot learning and can achieve impressive results without large-scale task-specific data collection or model parameter updating. More recent LLMs, such as GLaM, LaMDA, Gopher, and Megatron-Turing NLG, achieved state-of-the-art few-shot results on many tasks by scaling model size, using sparsely activated modules, and training on larger datasets from more diverse sources. Yet much work remains in understanding the capabilities that emerge with few-shot learning as we push the limits of model scale.
Last year Google Research announced our vision for Pathways, a single model that could generalize across domains and tasks while being highly efficient. An important milestone toward realizing this vision was to develop the new Pathways system to orchestrate distributed computation for accelerators. In “PaLM: Scaling Language Modeling with Pathways”, we introduce the Pathways Language Model (PaLM), a 540-billion parameter, dense decoder-only Transformer model trained with the Pathways system, which enabled us to efficiently train a single model across multiple TPU v4 Pods. We evaluated PaLM on hundreds of language understanding and generation tasks, and found that it achieves state-of-the-art few-shot performance across most tasks, by significant margins in many cases.
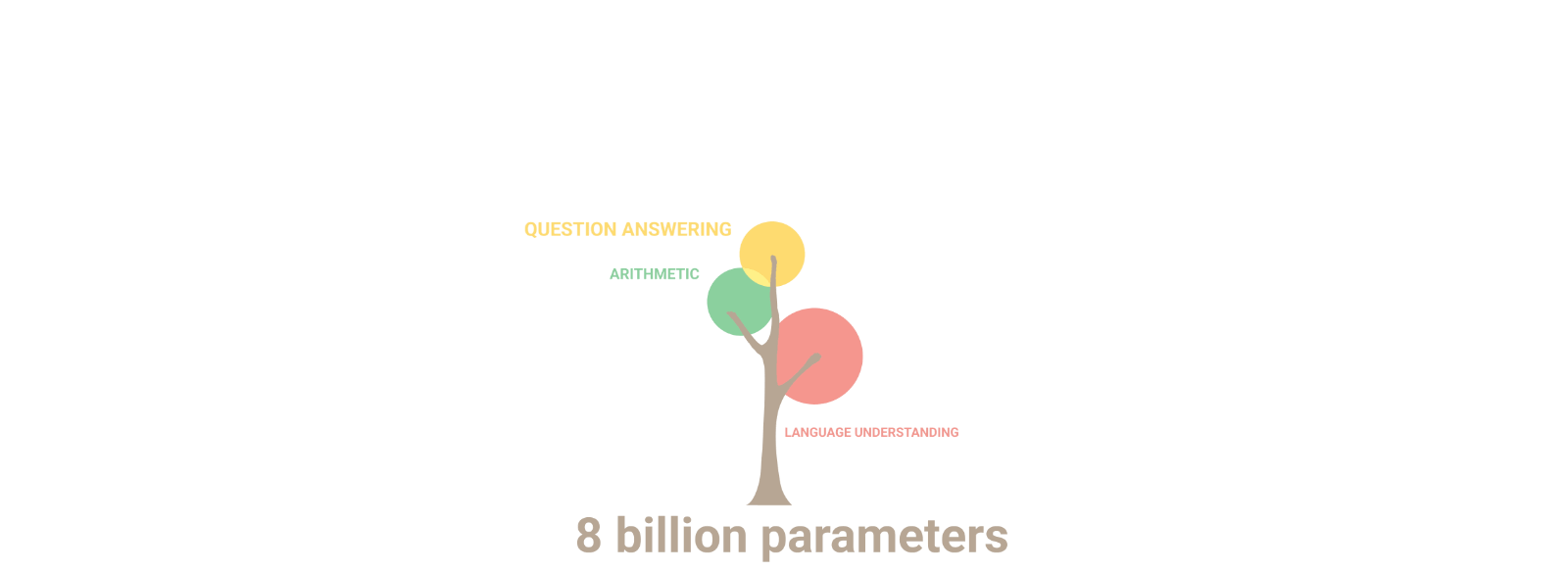 |
As the scale of the model increases, the performance improves across tasks while also unlocking new capabilities. |
Training a 540-Billion Parameter Language Model with Pathways
PaLM demonstrates the first large-scale use of the Pathways system to scale training to 6144 chips, the largest TPU-based system configuration used for training to date. The training is scaled using data parallelism at the Pod level across two Cloud TPU v4 Pods, while using standard data and model parallelism within each Pod. This is a significant increase in scale compared to most previous LLMs, which were either trained on a single TPU v3 Pod (e.g., GLaM, LaMDA), used pipeline parallelism to scale to 2240 A100 GPUs across GPU clusters (Megatron-Turing NLG) or used multiple TPU v3 Pods (Gopher) with a maximum scale of 4096 TPU v3 chips.
PaLM achieves a training efficiency of 57.8% hardware FLOPs utilization, the highest yet achieved for LLMs at this scale. This is due to a combination of the parallelism strategy and a reformulation of the Transformer block that allows for attention and feedforward layers to be computed in parallel, enabling speedups from TPU compiler optimizations.
PaLM was trained using a combination of English and multilingual datasets that include high-quality web documents, books, Wikipedia, conversations, and GitHub code. We also created a “lossless” vocabulary that preserves all whitespace (especially important for code), splits out-of-vocabulary Unicode characters into bytes, and splits numbers into individual tokens, one for each digit.
Breakthrough Capabilities on Language, Reasoning, and Code Tasks
PaLM shows breakthrough capabilities on numerous very difficult tasks. We highlight a few examples for language understanding and generation, reasoning, and code-related tasks below.
Language Understanding and Generation
We evaluated PaLM on 29 widely-used English natural language processing (NLP) tasks. PaLM 540B surpassed few-shot performance of prior large models, such as GLaM, GPT-3, Megatron-Turing NLG, Gopher, Chinchilla, and LaMDA, on 28 of 29 of tasks that span question-answering tasks (open-domain closed-book variant), cloze and sentence-completion tasks, Winograd-style tasks, in-context reading comprehension tasks, common-sense reasoning tasks, SuperGLUE tasks, and natural language inference tasks.
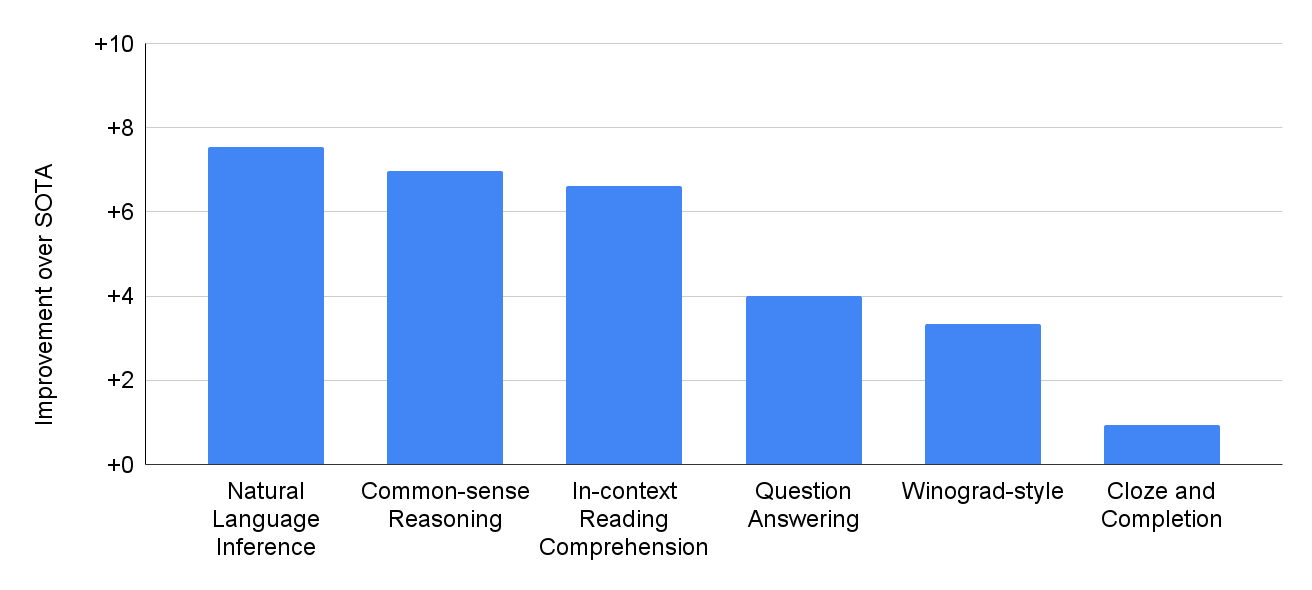 |
PaLM 540B performance improvement over prior state-of-the-art (SOTA) results on 29 English-based NLP tasks. |
In addition to English NLP tasks, PaLM also shows strong performance on multilingual NLP benchmarks, including translation, even though only 22% of the training corpus is non-English.
We also probe emerging and future capabilities of PaLM on the Beyond the Imitation Game Benchmark (BIG-bench), a recently released suite of more than 150 new language modeling tasks, and find that PaLM achieves breakthrough performance. We compare the performance of PaLM to Gopher and Chinchilla, averaged across a common subset of 58 of these tasks. Interestingly, we note that PaLM’s performance as a function of scale follows a log-linear behavior similar to prior models, suggesting that performance improvements from scale have not yet plateaued. PaLM 540B 5-shot also does better than the average performance of people asked to solve the same tasks.
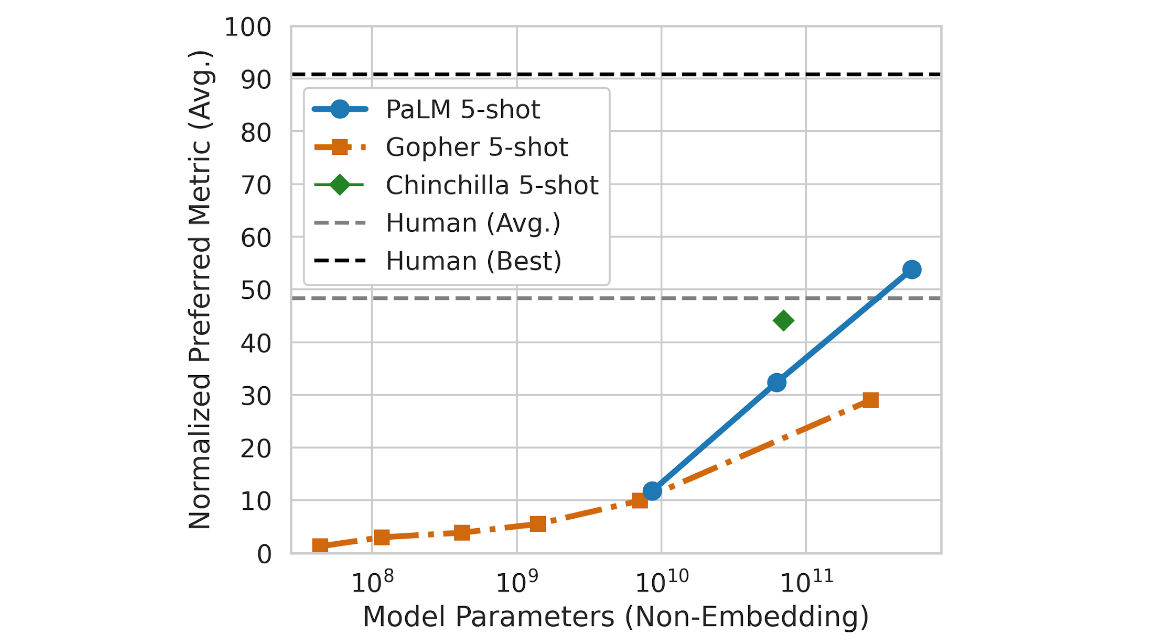 |
Scaling behavior of PaLM on a subset of 58 BIG-bench tasks. |
PaLM demonstrates impressive natural language understanding and generation capabilities on several BIG-bench tasks. For example, the model can distinguish cause and effect, understand conceptual combinations in appropriate contexts, and even guess the movie from an emoji.
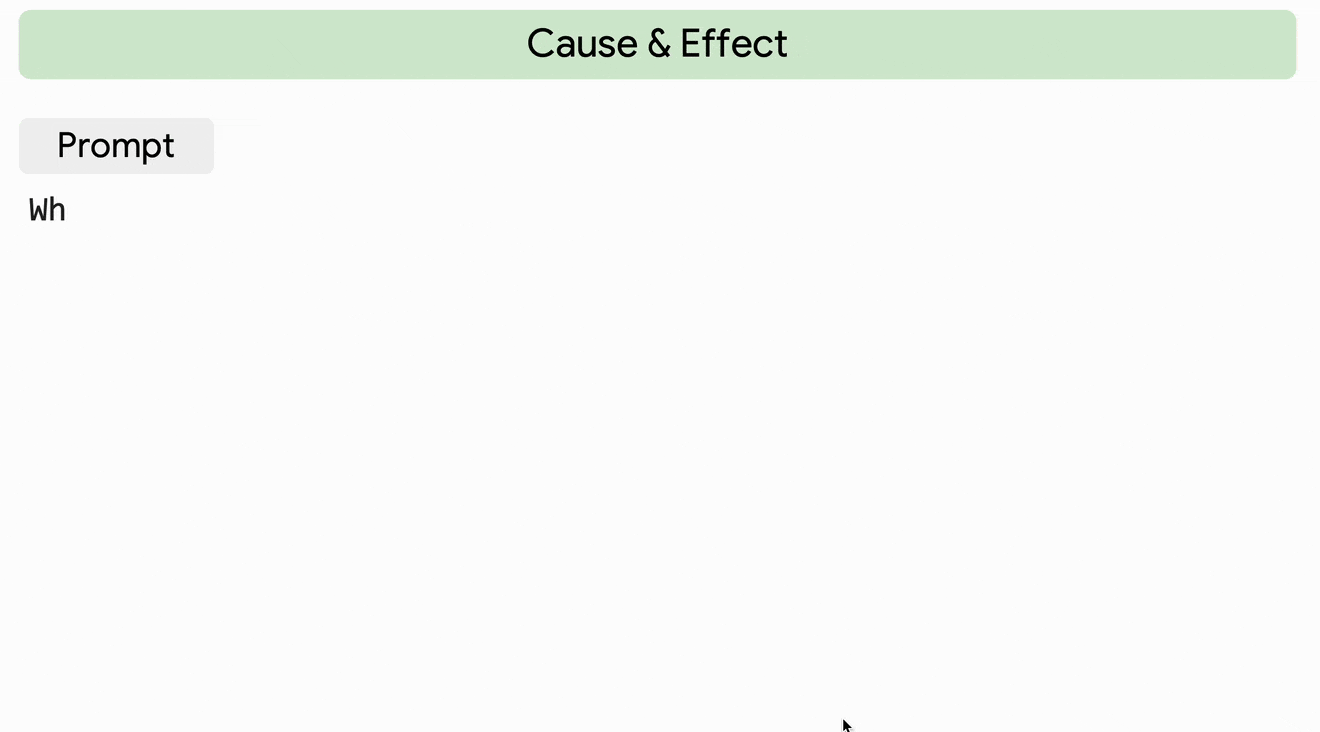 |
Examples that showcase PaLM 540B 1-shot performance on BIG-bench tasks: labeling cause and effect, conceptual understanding, guessing movies from emoji, and finding synonyms and counterfactuals. |
Reasoning
By combining model scale with chain-of-thought prompting, PaLM shows breakthrough capabilities on reasoning tasks that require multi-step arithmetic or common-sense reasoning. Prior LLMs, like Gopher, saw less benefit from model scale in improving performance.
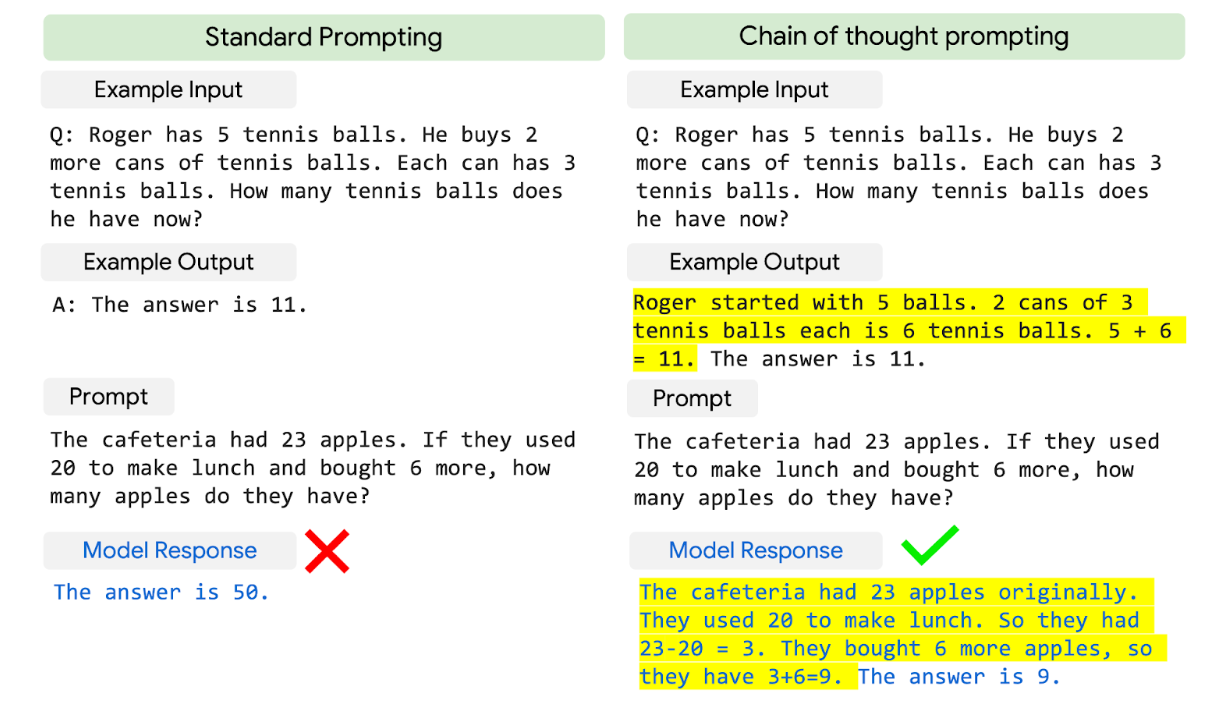 |
Standard prompting versus chain-of-thought prompting for an example grade-school math problem. Chain-of-thought prompting decomposes the prompt for a multi-step reasoning problem into intermediate steps (highlighted in yellow), similar to how a person would approach it. |
We observed strong performance from PaLM 540B combined with chain-of-thought prompting on three arithmetic datasets and two commonsense reasoning datasets. For example, with 8-shot prompting, PaLM solves 58% of the problems in GSM8K, a benchmark of thousands of challenging grade school level math questions, outperforming the prior top score of 55% achieved by fine-tuning the GPT-3 175B model with a training set of 7500 problems and combining it with an external calculator and verifier.
This new score is especially interesting, as it approaches the 60% average of problems solved by 9-12 year olds, who are the target audience for the question set. We suspect that separate encoding of digits in the PaLM vocabulary helps enable these performance improvements.
Remarkably, PaLM can even generate explicit explanations for scenarios that require a complex combination of multi-step logical inference, world knowledge, and deep language understanding. For example, it can provide high quality explanations for novel jokes not found on the web.
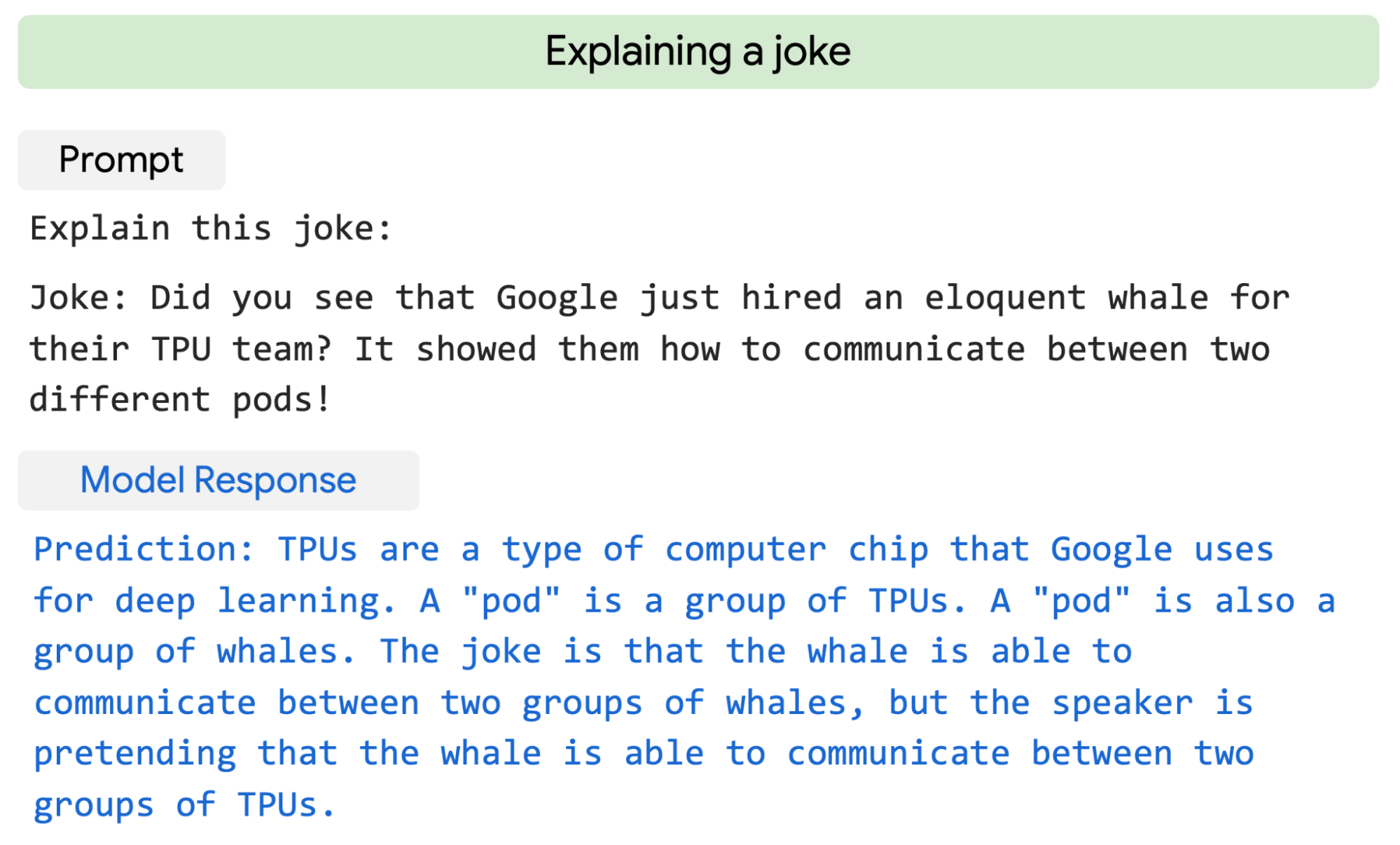 |
PaLM explains an original joke with two-shot prompts. |
Code Generation
LLMs have also been shown [1, 2, 3, 4] to generalize well to coding tasks, such as writing code given a natural language description (text-to-code), translating code from one language to another, and fixing compilation errors (code-to-code).
PaLM 540B shows strong performance across coding tasks and natural language tasks in a single model, even though it has only 5% code in the pre-training dataset. Its few-shot performance is especially remarkable because it is on par with the fine-tuned Codex 12B while using 50 times less Python code for training. This result reinforces earlier findings that larger models can be more sample efficient than smaller models because they better transfer learning from other programming languages and natural language data.
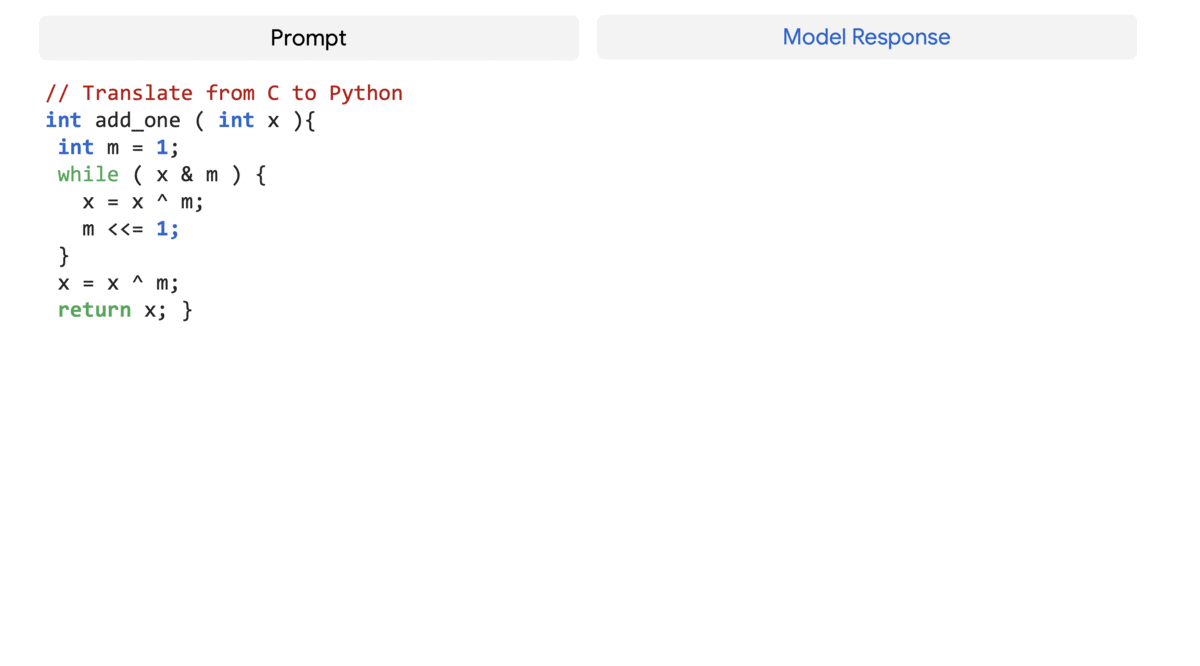 |
Examples of a fine-tuned PaLM 540B model on text-to-code tasks, such as GSM8K-Python and HumanEval, and code-to-code tasks, such as Transcoder. |
We also see a further increase in performance by fine-tuning PaLM on a Python-only code dataset, which we refer to as PaLM-Coder. For an example code repair task called DeepFix, where the objective is to modify initially broken C programs until they compile successfully, PaLM-Coder 540B demonstrates impressive performance, achieving a compile rate of 82.1%, which outperforms the prior 71.7% state of the art. This opens up opportunities for fixing more complex errors that arise during software development.
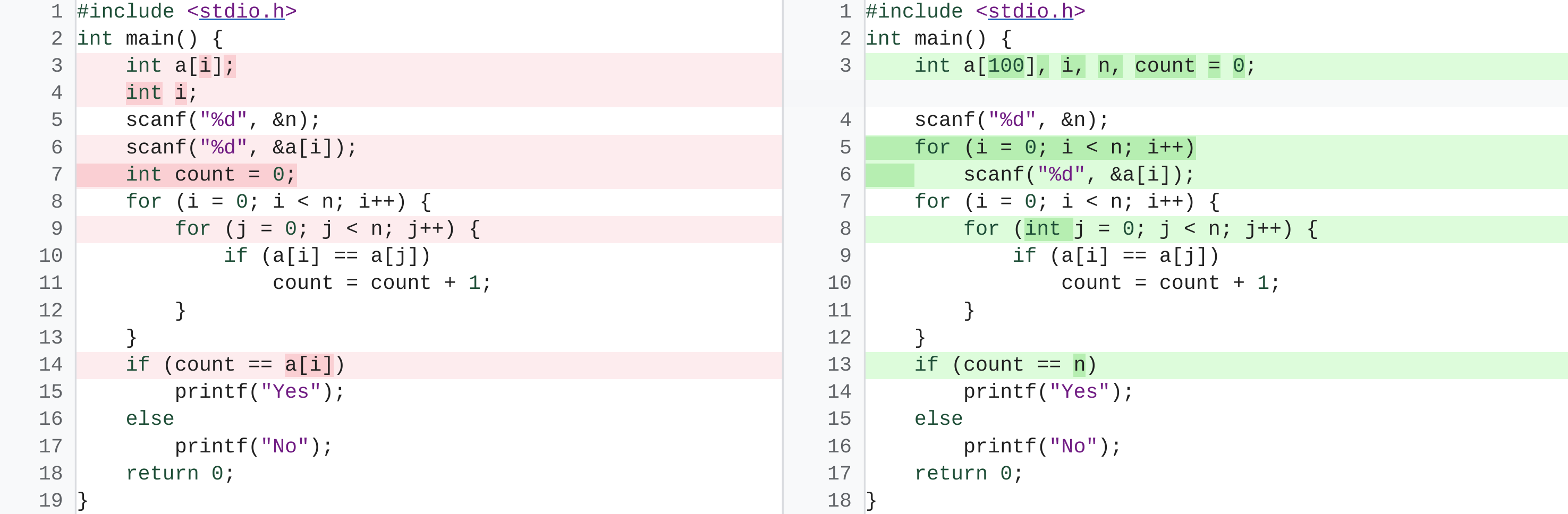 |
An example from the DeepFix Code Repair task. The fine-tuned PaLM-Coder 540B fixes compilation errors (left, in red) to a version of code that compiles (right). |
Ethical Considerations
Recent research has highlighted various potential risks associated with LLMs trained on web text. It is crucial to analyze and document such potential undesirable risks through transparent artifacts such as model cards and datasheets, which also include information on intended use and testing. To this end, our paper provides a datasheet, model card and Responsible AI benchmark results, and it reports thorough analyses of the dataset and model outputs for biases and risks. While the analysis helps outline some potential risks of the model, domain- and task-specific analysis is essential to truly calibrate, contextualize, and mitigate possible harms. Further understanding of risks and benefits of these models is a topic of ongoing research, together with developing scalable solutions that can put guardrails against malicious uses of language models.
Conclusion and Future Work
PaLM demonstrates the scaling capability of the Pathways system to thousands of accelerator chips across two TPU v4 Pods by training a 540-billion parameter model efficiently with a well-studied, well-established recipe of a dense decoder-only Transformer model. Pushing the limits of model scale enables breakthrough few-shot performance of PaLM across a variety of natural language processing, reasoning, and code tasks.
PaLM paves the way for even more capable models by combining the scaling capabilities with novel architectural choices and training schemes, and brings us closer to the Pathways vision:
“Enable a single AI system to generalize across thousands or millions of tasks, to understand different types of data, and to do so with remarkable efficiency.” |
Acknowledgements
PaLM is the result of a large, collaborative effort by many teams within Google Research and across Alphabet. We’d like to thank the entire PaLM team for their contributions: Jacob Devlin, Maarten Bosma, Gaurav Mishra, Adam Roberts, Paul Barham, Hyung Won Chung, Charles Sutton, Sebastian Gehrmann, Parker Schuh, Kensen Shi, Sasha Tsvyashchenko, Joshua Maynez, Abhishek Rao, Parker Barnes, Yi Tay, Noam Shazeer, Vinodkumar Prabhakaran, Emily Reif, Nan Du, Ben Hutchinson, Reiner Pope, James Bradbury, Jacob Austin, Michael Isard, Guy Gur-Ari, Pengcheng Yin, Toju Duke, Anselm Levskaya, Sanjay Ghemawat, Sunipa Dev, Henryk Michalewski, Xavier Garcia, Vedant Mishra, Kevin Robinson, Liam Fedus, Denny Zhou, Daphne Ippolito, David Luan, Hyeontaek Lim, Barret Zoph, Alexander Spiridonov, Ryan Sepassi, David Dohan, Shivani Agrawal, Mark Omernick, Andrew Dai, Thanumalayan Sankaranarayana Pillai, Marie Pellat, Aitor Lewkowycz, Erica Moreira, Rewon Child, Oleksandr Polozov, Katherine Lee, Zongwei Zhou, Xuezhi Wang, Brennan Saeta, Mark Diaz, Orhan Firat, Michele Catasta, and Jason Wei. PaLM builds on top of work by many, many teams at Google and we would especially like to recognize the T5X team, the Pathways infrastructure team, the JAX team, the Flaxformer team, the XLA team, the Plaque team, the Borg team, and the Datacenter networking infrastructure team. We’d like to thank our co-authors on this blog post, Alexander Spiridonov and Maysam Moussalem, as well as Josh Newlan and Tom Small for the images and animations in this blog post. Finally, we would like to thank our advisors for the project: Noah Fiedel, Slav Petrov, Jeff Dean, Douglas Eck, and Kathy Meier-Hellstern.