The first open-source release of GPU kernel modules for the Linux community help improve NVIDIA GPU driver quality and security.
NVIDIA is now publishing Linux GPU kernel modules as open source with dual GPL/MIT license, starting with the R515 driver release. You can find the source code for these kernel modules in the NVIDIA Open GPU Kernel Modules repo on GitHub.
This release is a significant step toward improving the experience of using NVIDIA GPUs in Linux, for tighter integration with the OS and for developers to debug, integrate, and contribute back. For Linux distribution providers, the open-source modules increase ease of use. They also improve the out-of-the-box user experience to sign and distribute the NVIDIA GPU driver. Canonical and SUSE are able to immediately package the open kernel modules with Ubuntu and SUSE Linux Enterprise Distributions.
Developers can trace into code paths and see how kernel event scheduling is interacting with their workload for faster root cause debugging. In addition, enterprise software developers can now integrate the driver seamlessly into the customized Linux kernel configured for their project.
This will further help improve NVIDIA GPU driver quality and security with input and reviews from the Linux end-user community.
With each new driver release, NVIDIA publishes a snapshot of the source code on GitHub. Community submitted patches are reviewed and if approved, integrated into a future driver release.
Refer to the NVIDIA contribution guidelines and overview of the driver release cadence and life-cycle documentation for more information.
Supported functionality
The first release of the open GPU kernel modules is R515. Along with the source code, fully-built and packaged versions of the drivers are provided.
For data center GPUs in the NVIDIA Turing and NVIDIA Ampere architecture families, this code is production ready. This was made possible by the phased rollout of the GSP driver architecture over the past year, designed to make the transition easy for NVIDIA customers. We focused on testing across a wide variety of workloads to ensure feature and performance parity with the proprietary kernel-mode driver.
In the future, functionality such as HMM will be a foundational component for confidential computing on the NVIDIA Hopper architecture.
In this open-source release, support for GeForce and Workstation GPUs is alpha quality. GeForce and Workstation users can use this driver on Turing and NVIDIA Ampere architecture GPUs to run Linux desktops and use features such as multiple displays, G-SYNC, and NVIDIA RTX ray tracing in Vulkan and NVIDIA OptiX. Users can opt in using the kernel module parameter NVreg_EnableUnsupportedGpus
as highlighted in the documentation. More robust and fully featured GeForce and Workstation support will follow in subsequent releases and the NVIDIA Open Kernel Modules will eventually supplant the closed-source driver.
Customers with Turing and Ampere GPUs can choose which modules to install. Pre-Turing customers will continue to run the closed source modules.
The open-source kernel-mode driver works with the same firmware and the same user-mode stacks such as CUDA, OpenGL, and Vulkan. However, all components of the driver stack must match versions within a release. For instance, you cannot take a release of the source code, build, and run it with the user-mode stack from a previous or future release.
Refer to the driver README document for instructions on installing the right versions and additional troubleshooting steps.
Installation opt in
The R515 release contains precompiled versions of both the closed-source driver and the open-source kernel modules. These versions are mutually exclusive, and the user can make the choice at install time. The default option ensures that silent installs will pick the optimal path for NVIDIA Volta and older GPUs versus Turing+ GPUs.
Users can build kernel modules from the source code and install them with the relevant user-mode drivers.
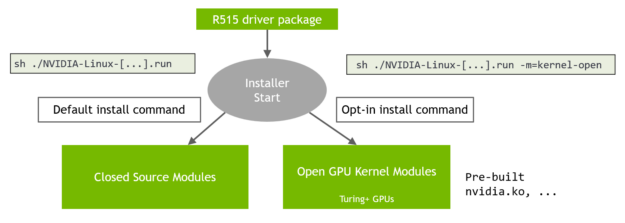
Partner ecosystem
NVIDIA has been working with Canonical, Red Hat, and SUSE for better packaging, deployment, and support models for our mutual customers.
Canonical
“The new NVIDIA open-source GPU kernel modules will simplify installs and increase security for Ubuntu users, whether they’re AI/ML developers, gamers, or cloud users,” commented Cindy Goldberg, VP of Silicon alliances at Canonical. “As the makers of Ubuntu, the most popular Linux-based operating system for developers, we can now provide even better support to developers working at the cutting edge of AI and ML by enabling even closer integration with NVIDIA GPUs on Ubuntu.”
In the coming months, the NVIDIA Open GPU kernel modules will make their way into the recently launched Canonical Ubuntu 22.04 LTS.
SUSE
“We at SUSE are excited that NVIDIA is releasing their GPU kernel-mode driver as open source. This is a true milestone for the open-source community and accelerated computing. SUSE is proud to be the first major Linux distribution to deliver this breakthrough with SUSE Linux Enterprise 15 SP4 in June. Together, NVIDIA and SUSE power your GPU-accelerated computing needs across cloud, data center, and edge with a secure software supply chain and excellence in support.” — Markus Noga, General Manager, Business Critical Linux at SUSE
Red Hat
“Enterprise open source can spur innovation and improve customers’ experience, something that Red Hat has always championed. We applaud NVIDIA’s decision to open source its GPU kernel driver. Red Hat has collaborated with NVIDIA for many years, and we are excited to see them take this next step. We look forward to bringing these capabilities to our customers and to improve interoperability with NVIDIA hardware.” — Mike McGrath, Vice President, Linux Engineering at Red Hat
Upstream approach
NVIDIA GPU drivers have been designed over the years to share code across operating systems, GPUs and Jetson SOCs so that we can provide a consistent experience across all our supported platforms. The current codebase does not conform to the Linux kernel design conventions and is not a candidate for Linux upstream.
There are plans to work on an upstream approach with the Linux kernel community and partners such as Canonical, Red Hat, and SUSE.
In the meantime, published source code serves as a reference to help improve the Nouveau driver. Nouveau can leverage the same firmware used by the NVIDIA driver, exposing many GPU functionalities, such as clock management and thermal management, bringing new features to the in-tree Nouveau driver.
Stay tuned for more developments in future driver releases and collaboration on GitHub.
Frequently asked questions
Where can I download the R515 driver?
You can download the R515 development driver as part of CUDA Toolkit 11.7, or from the driver downloads page under “Beta” drivers. The R515 data center driver will follow in subsequent releases per our usual cadence.
Can open GPU Kernel Modules be distributed?
Yes, the NVIDIA open kernel modules are licensed under a dual GPL/MIT license; and the terms of licenses govern the distribution and repackaging grants.
Will the source for user-mode drivers such as CUDA be published?
These changes are for the kernel modules; while the user-mode components are untouched. So the user-mode will remain closed source and published with pre-built binaries in the driver and the CUDA toolkit.
Which GPUs are supported by Open GPU Kernel Modules?
Open kernel modules support all Ampere and Turing GPUs. Datacenter GPUs are supported for production, and support for GeForce and Workstation GPUs is alpha quality. Please refer to the Datacenter, NVIDIA RTX, and GeForce product tables for more details (Turing and above have compute capability of 7.5 or greater).
How to report bugs
Problems can be reported through the GitHub repository issue tracker or through our existing end-user support forum. Please report security issues through the channels listed on the GitHub repository security policy.
What is the process for patch submission and SLA/CLA for patches?
We encourage community submissions through pull requests on the GitHub page. The submitted patches will be reviewed and if approved, integrated with possible modifications into a future driver release. Please refer to the NVIDIA driver lifecycle document.
The published source code is a snapshot generated from a shared codebase, so contributions may not be reflected as separate Git commits in the GitHub repo. We are working on a process for acknowledging community contributions. We also advise against making significant reformatting of the code for the same reasons.
The process for submitting pull requests is described on our GitHub page and such contributions are covered under the contributor license agreement (CLA).
More detailed FAQs are also available on the GitHub page.