Learn how financial institutions are using high-quality synthetic data to validate explainable AI models and comply with data privacy regulations.
Data sits at the heart of model explainability. Explainable AI (XAI) is a rapidly advancing field looking to provide insights into the complex decision-making processes of AI algorithms.
Where AI has a significant impact on individuals’ lives, like credit risk scoring, managers and consumers alike rightfully demand insight into these decisions. leading financial institutions are already leveraging XAI for validating their models. Similarly, regulators are also demanding insight into financial institutions’ algorithmic environment. But how is it possible to do that in practice?
Pandora’s closed box
The more advanced AI gets, the more important data becomes for explainability.
Modern day ML algorithms have ensemble methods and deep learning that result in thousands, if not millions of model parameters. They are impossible to grasp without seeing them in action when applied to actual data.
The need for broad access to data is apparent even and especially in cases where the training data is sensitive. Financial and healthcare data used for credit scoring and insurance pricing are some of the most frequently used, but also some of the most sensitive data types in AI.
It’s a conundrum of opposing needs: You want your data protected and you want a transparent decision.
Explainable AI needs data
So, how can these algorithms be made transparent? How can you judge model decisions made by machines? Given their complexity, disclosing the mathematical model, implementation, or the full training data won’t serve the purpose.
Instead, you have to explore a system’s behavior by observing its decisions across a variety of actual cases and probe its sensitivity with respect to modifications. These sample-based, what-if explorations help our understanding of what drives the decision of a model.
This simple yet powerful concept of systematically exploring changes in model output given variations of input data is also referred to as local interpretability and can be performed domain– and model-agnostic at scale. Thus, the same principle can be applied to help interpret credit-scoring systems, sales demand forecasts, fraud detection systems, text classifiers, recommendation systems, and more.
However, local interpretability methods like SHAP require access not only to the model but also to a large number of representative and relevant data samples.
Figure 1 shows a basic demonstration, performed on a model, predicting customer response to marketing activity within the finance industry. Looking at the corresponding Python calls reveals the need for the trained model, as well as a representative dataset for performing these types of analyses. However, what if that data is actually sensitive and can’t be accessed by AI model validators?

Synthetic data for scaling XAI across teams
In the early days of AI adoption, it was typically the same group of engineers who developed models and validated them. In both cases, they used real-world production data.
Given the real-world impact of algorithms on individuals, it is now increasingly understood that independent groups should inspect and assess models and their implications. These people would ideally bring diverse perspectives to the table from engineering and non-engineering backgrounds.
External auditors and certification bodies are being contracted to establish additional confidence that the algorithms are fair, unbiased, and nondiscriminative. However, privacy concerns and modern-day data protection regulations, like GDPR, limit access to representative validation data. This severely hampers model validation being broadly conducted.
Fortunately, model validation can be performed using high-quality AI-generated synthetic data that serves as a highly accurate, anonymized, drop-in replacement for sensitive data. For example, MOSTLY AI’s synthetic data platform enables organizations to generate synthetic datasets in a fully self-service, automated manner.
Figure 2 shows the XAI analysis being performed for the model with synthetic data. There are barely any discernible differences in results when comparing Figure 1 and Figure 2. The same insights and inspections are possible by leveraging MOSTLY AI’s privacy-safe synthetic data, which finally enables true collaboration to perform XAI at scale and on a continuous basis.
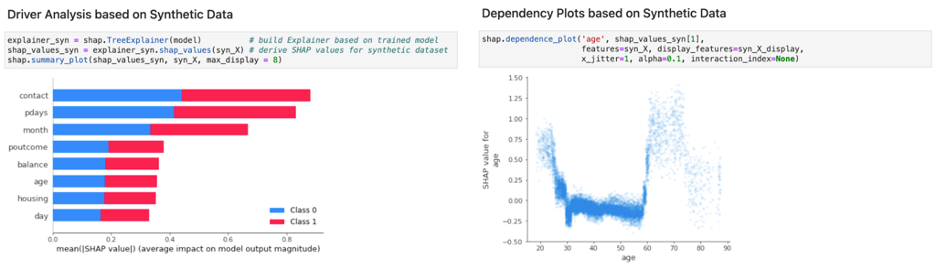
Figure 3 shows the process of scaling model validation across teams. An organization runs a state-of-the-art synthetic data solution within their controlled compute environment. It continuously generates synthetic replicas of their data assets, which can be shared with a diverse team of internal and external AI validators.
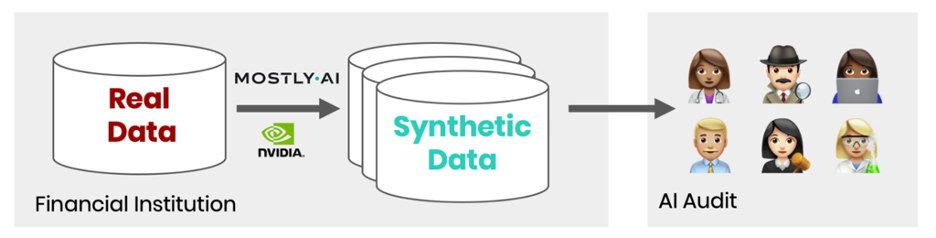
Scaling to real-world data volumes with GPUs
GPU-accelerated libraries, like RAPIDS and Plotly, enable model validation at the scale required for real-world use cases encountered in practice. The same applies to generating synthetic data, where AI-powered synthetization solutions such as MOSTLY AI can benefit significantly from running on top of a full-stack accelerated computing platform. For more information, see Accelerating Trustworthy AI for Credit Risk Management.
To demonstrate, we turned to the mortgage loan dataset published by Fannie Mae (FNMA) for the purpose of validating an ML model for loan delinquencies. We started by generating a statistical representative synthetic replica of the training data, consisting of tens of millions of synthetic loans, composed of dozens of synthetic attributes (Figure 4).
All data is being artificially created and no single record can be linked back to any actual record from the original dataset. However, the structure, patterns, and correlations of the data are faithfully retained in the synthetic dataset.
This ability to capture the diversity and richness of data is critical for model validation. The process seeks to validate model behavior not only on the dominant majority classes but also on under-represented and most vulnerable minority segments within a population.
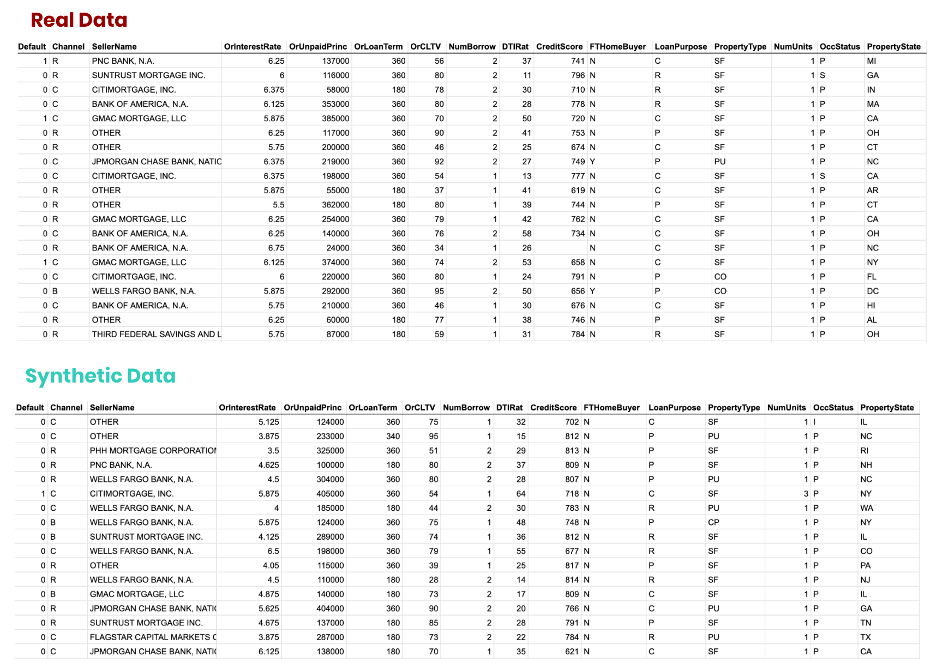
Given the generated synthetic data, you can then use GPU-accelerated XAI libraries to compute statistics of interest to assess model behavior.
Figure 5, for example, displays a side-by-side comparison of SHAP values: the loan delinquency model being explained on the real data and after being explained on the synthetic data. The same conclusions regarding the model can be reliably derived by using high-quality synthetic data as a drop-in alternative to the sensitive original data.

Figure 5 shows that synthetic data serves as a safe drop-in replacement for the actual data for explaining model behavior.
Further, the ability of synthetic data generators to yield an arbitrary amount of new data enables you to improve the model validation significantly for smaller groups.
Figure 6 shows a side-by-side comparison of SHAP values for a specific ZIP code found within the dataset. While the original data had less than 100 loans for a given geography, we leverage 10x the data volume to inspect the model behavior in that area, enabling more detail and richer insights.
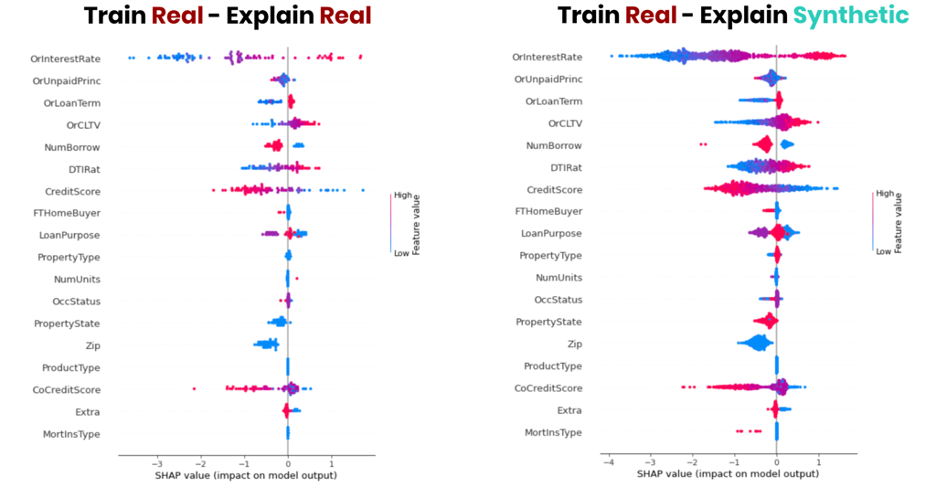
Individual-level inspection with synthetic samples
While summary statistics and visualizations are key to analyzing the general model behavior, our understanding of models further benefits from inspecting individual samples on a case-by-case basis.
XAI tooling reveals the impact of multiple signals on the final model decision. These cases need not necessarily be actual cases, as long as synthetic data is realistic and representative.
Figure 7 displays four randomly generated synthetic cases with their final model predictions and corresponding decomposition for each of the input variables. This enables you to gain insights on what factor contributed to what extent and what direction to the model decision for unlimited potential cases without exposing the privacy of any individual.
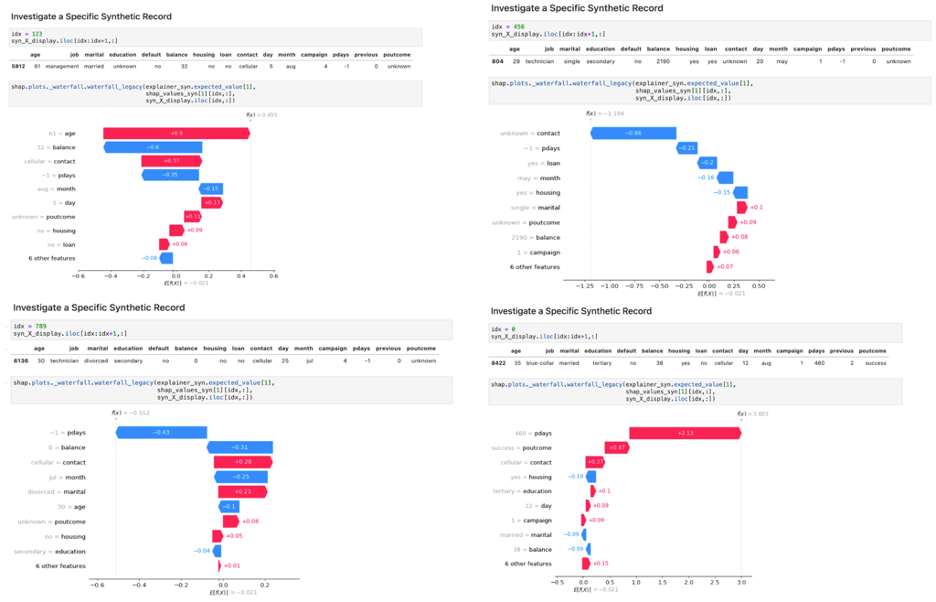
Effective AI governance with synthetic data
AI-powered services are becoming more present across private and public sectors, playing an ever bigger role in our daily lives. Yet, we are only at the dawn of AI governance.
While regulations, like Europe’s proposed AI Act, will take time to manifest, developers and decision-makers must act responsibly today and adopt XAI best practices. Synthetic data enables a collaborative, broad setting, without putting the privacy of customers at risk. It’s a powerful, novel tool to support the development and governance of fair and robust AI.
For more information about AI explainability in banking, see the following resources: