The verdict is in: A GeForce NOW Ultimate membership raises the bar on gaming. Members have been tackling the Ultimate KovvaK’s challenge head-on and seeing for themselves how the power of Ultimate improves their gaming with 240 frames per second streaming. The popular training title that helps gamers improve their aim fully launches in the Read article >
Wanna help judge SoME3?
There has been great progress towards adapting large language models (LLMs) to accommodate multimodal inputs for tasks including image captioning, visual question answering (VQA), and open vocabulary recognition. Despite such achievements, current state-of-the-art visual language models (VLMs) perform inadequately on visual information seeking datasets, such as Infoseek and OK-VQA, where external knowledge is required to answer the questions.
![]() |
Examples of visual information seeking queries where external knowledge is required to answer the question. Images are taken from the OK-VQA dataset. |
In “AVIS: Autonomous Visual Information Seeking with Large Language Models”, we introduce a novel method that achieves state-of-the-art results on visual information seeking tasks. Our method integrates LLMs with three types of tools: (i) computer vision tools for extracting visual information from images, (ii) a web search tool for retrieving open world knowledge and facts, and (iii) an image search tool to glean relevant information from metadata associated with visually similar images. AVIS employs an LLM-powered planner to choose tools and queries at each step. It also uses an LLM-powered reasoner to analyze tool outputs and extract key information. A working memory component retains information throughout the process.
![]() |
An example of AVIS’s generated workflow for answering a challenging visual information seeking question. The input image is taken from the Infoseek dataset. |
Comparison to previous work
Recent studies (e.g., Chameleon, ViperGPT and MM-ReAct) explored adding tools to LLMs for multimodal inputs. These systems follow a two-stage process: planning (breaking down questions into structured programs or instructions) and execution (using tools to gather information). Despite success in basic tasks, this approach often falters in complex real-world scenarios.
There has also been a surge of interest in applying LLMs as autonomous agents (e.g., WebGPT and ReAct). These agents interact with their environment, adapt based on real-time feedback, and achieve goals. However, these methods do not restrict the tools that can be invoked at each stage, leading to an immense search space. Consequently, even the most advanced LLMs today can fall into infinite loops or propagate errors. AVIS tackles this via guided LLM use, influenced by human decisions from a user study.
Informing LLM decision making with a user study
Many of the visual questions in datasets such as Infoseek and OK-VQA pose a challenge even for humans, often requiring the assistance of various tools and APIs. An example question from the OK-VQA dataset is shown below. We conducted a user study to understand human decision-making when using external tools.
![]() |
We conducted a user study to understand human decision-making when using external tools. Image is taken from the OK-VQA dataset. |
The users were equipped with an identical set of tools as our method, including PALI, PaLM, and web search. They received input images, questions, detected object crops, and buttons linked to image search results. These buttons offered diverse information about the detected object crops, such as knowledge graph entities, similar image captions, related product titles, and identical image captions.
We record user actions and outputs and use it as a guide for our system in two key ways. First, we construct a transition graph (shown below) by analyzing the sequence of decisions made by users. This graph defines distinct states and restricts the available set of actions at each state. For example, at the start state, the system can take only one of these three actions: PALI caption, PALI VQA, or object detection. Second, we use the examples of human decision-making to guide our planner and reasoner with relevant contextual instances to enhance the performance and effectiveness of our system.
![]() |
AVIS transition graph. |
General framework
Our approach employs a dynamic decision-making strategy designed to respond to visual information-seeking queries. Our system has three primary components. First, we have a planner to determine the subsequent action, including the appropriate API call and the query it needs to process. Second, we have a working memory that retains information about the results obtained from API executions. Last, we have a reasoner, whose role is to process the outputs from the API calls. It determines whether the obtained information is sufficient to produce the final response, or if additional data retrieval is required.
The planner undertakes a series of steps each time a decision is required regarding which tool to employ and what query to send to it. Based on the present state, the planner provides a range of potential subsequent actions. The potential action space may be so large that it makes the search space intractable. To address this issue, the planner refers to the transition graph to eliminate irrelevant actions. The planner also excludes the actions that have already been taken before and are stored in the working memory.
Next, the planner collects a set of relevant in-context examples that are assembled from the decisions previously made by humans during the user study. With these examples and the working memory that holds data collected from past tool interactions, the planner formulates a prompt. The prompt is then sent to the LLM, which returns a structured answer, determining the next tool to be activated and the query to be dispatched to it. This design allows the planner to be invoked multiple times throughout the process, thereby facilitating dynamic decision-making that gradually leads to answering the input query.
We employ a reasoner to analyze the output of the tool execution, extract the useful information and decide into which category the tool output falls: informative, uninformative, or final answer. Our method utilizes the LLM with appropriate prompting and in-context examples to perform the reasoning. If the reasoner concludes that it’s ready to provide an answer, it will output the final response, thus concluding the task. If it determines that the tool output is uninformative, it will revert back to the planner to select another action based on the current state. If it finds the tool output to be useful, it will modify the state and transfer control back to the planner to make a new decision at the new state.
![]() |
AVIS employs a dynamic decision-making strategy to respond to visual information-seeking queries. |
Results
We evaluate AVIS on Infoseek and OK-VQA datasets. As shown below, even robust visual-language models, such as OFA and PaLI, fail to yield high accuracy when fine-tuned on Infoseek. Our approach (AVIS), without fine-tuning, achieves 50.7% accuracy on the unseen entity split of this dataset.
![]() |
AVIS visual question answering results on Infoseek dataset. AVIS achieves higher accuracy in comparison to previous baselines based on PaLI, PaLM and OFA. |
Our results on the OK-VQA dataset are shown below. AVIS with few-shot in-context examples achieves an accuracy of 60.2%, higher than most of the previous works. AVIS achieves lower but comparable accuracy in comparison to the PALI model fine-tuned on OK-VQA. This difference, compared to Infoseek where AVIS outperforms fine-tuned PALI, is due to the fact that most question-answer examples in OK-VQA rely on common sense knowledge rather than on fine-grained knowledge. Therefore, PaLI is able to encode such generic knowledge in the model parameters and doesn’t require external knowledge.
![]() |
Visual question answering results on A-OKVQA. AVIS achieves higher accuracy in comparison to previous works that use few-shot or zero-shot learning, including Flamingo, PaLI and ViperGPT. AVIS also achieves higher accuracy than most of the previous works that are fine-tuned on OK-VQA dataset, including REVEAL, ReVIVE, KAT and KRISP, and achieves results that are close to the fine-tuned PaLI model. |
Conclusion
We present a novel approach that equips LLMs with the ability to use a variety of tools for answering knowledge-intensive visual questions. Our methodology, anchored in human decision-making data collected from a user study, employs a structured framework that uses an LLM-powered planner to dynamically decide on tool selection and query formation. An LLM-powered reasoner is tasked with processing and extracting key information from the output of the selected tool. Our method iteratively employs the planner and reasoner to leverage different tools until all necessary information required to answer the visual question is amassed.
Acknowledgements
This research was conducted by Ziniu Hu, Ahmet Iscen, Chen Sun, Kai-Wei Chang, Yizhou Sun, David A. Ross, Cordelia Schmid and Alireza Fathi.
Take a Free NVIDIA Technical Training Course
Join the free NVIDIA Developer Program and enroll in a course from the NVIDIA Deep Learning Institute.
Join the free NVIDIA Developer Program and enroll in a course from the NVIDIA Deep Learning Institute.
Demand for real-time insights and autonomous decision-making is growing in various industries. To meet this demand, we need scalable edge-solution platforms…
Demand for real-time insights and autonomous decision-making is growing in various industries. To meet this demand, we need scalable edge-solution platforms that can effectively process AI-enabled sensor data right at the source and scale out to on-premises or cloud compute resources.
However, developers face many challenges in using AI and sensor processing at the edge:
- Real-time latency requirements
- The complexity of building and maintaining custom pipelines for AI-enabled sensor processing
- The need for hardware-agnostic solutions to meet heterogeneous hardware needs at the edge
- Multimodality and processing of various sensory modalities
- Integration from edge to on-premises to a cloud-distributed network
- Long-term whole-stack stability
Before NVIDIA Holoscan, no singular platform offered a comprehensive solution that effectively addressed the multitude of edge AI challenges. By seamlessly integrating data movement, accelerated computing, real-time visualization, and AI inferencing, Holoscan ensures optimal application performance. It abstracts away complexities for developers, reduces time to market, and offers the convenience of coding in Python and C++, all in a low-code, high-performance infrastructure.
“The Holoscan platform enables new SaMD (software as a medical device) to be quickly productized with seamless integration with the development environment to enable SaMD productization and fast-track deployment,” said Nhan Ngo Dinh, president of Cosmo Intelligent Medical Devices. “This accelerates the time to market with integrated development programmable using the NVIDIA Holoscan SDK, easy to transition from development to production.”
For edge developers, navigating the heterogeneous landscape of edge devices with varying hardware requirements and architectures can be daunting. Holoscan simplifies this complexity through its hardware-agnostic approach.
The platform provides a unified stack, accommodating a wide range of devices from x86 to aarch64 and NVIDIA Jetson Orin AGX to NVIDIA IGX Orin, catering to different power, size, cost, compute, and configuration needs. This versatility liberates you from hardware constraints while promoting interoperability, maintainability, and scalability across applications.
The v0.6 release of NVIDIA Holoscan introduces new features that empower you to reach new levels of scalability, productivity, and ease of use when building AI-streaming solutions. Specifically, this new set of features enables the following benefits:
- Scalability at the edge through a distributed computing architecture
- Portability, collaboration, and interoperability for platform development
- Advanced profiling with data frame flow tracking for optimal performance
Scalability through distributed computing architecture
For developers with heavy workloads or interested in scaling up and out, the Holoscan v0.6 multi-GPU, multi-node support enables distributed computing. Specifically, you can now deploy distributed applications and use all the resources available on the edge with multiple GPUs on a single node. Or you can deploy a single Holoscan application on separate physical nodes with optimized network communication.
Multi-GPU, multi-node enables sensor processing applications to scale with ever-increasing compute requirements and grants you more flexibility and scalability in your designs. For users, it opens new possibilities with increased processing power, parallel processing, separating workloads based on criticality, post-deployment scale-up without replacing existing units, fault tolerance, and reliability.

Portability, collaboration, and interoperability for platform developers
If you are developing a platform instead of creating standalone products from scratch, Holoscan v0.6 provides a set of new features enabling more scalability and flexibility for broader use and ease of integration:
- App packager: Grants portability through the easy containerization and deployment of the apps, enhancing collaboration and contributions to the platform.
- Multi-backend: Streamlines the transition from model training to AI app building enabled by the plug-and-play deployment of already trained PyTorch models.
- Holoviz volumetric rendering: Provides built-in volumetric rendering in support of medical imaging visualization.
Advanced profiling with data frame flow tracking
The upcoming release includes a data frame flow tracking feature that enables you to measure performance through data frame tracking so that you can quickly adjust bottlenecks. In addition, the new multithreaded scheduler enables applications to run operators in parallel. As a result, the applications have optimized the use of system resources.
Use cases and success stories
The NVIDIA Holoscan SDK has rapidly evolved into an accelerated, full-stack infrastructure, making significant contributions to scalable, software-defined, and real-time processing across various domains.
The following healthcare companies have embraced and built on this technology:
- Medtronic, the largest medical device company in the world, is building a next-generation AI-assisted colonoscopy system (pending FDA approval) on the Holoscan platform.
- Moon Surgical, a Paris-based robotic surgery company, is finalizing the productization of Maestro, an accessible, adaptive surgical-assistant robotics system built on Holoscan and IGX.
- ORSI Academy, a surgical training center in Belgium, has used Holoscan to support first-in-human real-world, robot-assisted surgery for critical operations like the removal of cancerous kidneys.
“Holoscan abstracts away the challenges in building safe and reliable sensor data processing pipelines for medical device applications. Traditionally such pipelines required dedicated teams of specialists to develop and maintain,” said David Noonan, CTO at Moon Surgical. Using Holoscan, we’re able to launch innovative features for our Maestro surgical robotics system with short development timelines while maintaining a lean R&D team.”
Other use cases include AR/VR, radar technology, and scientific instrumentation.
Magic Leap is working on a Holoscan-based solution that combines AI with true augmented reality to revolutionize physician and surgeon training, visualization, and complex procedure performance.
Researchers from the Georgia Tech Research Institute used Holoscan to develop a real-time radar application used in defense, aerospace, meteorology, navigation, and surveillance.
- NVIDIA and Analog Devices collaborated to build a 5G Instrumentation application that leverages Holoscan for compute-intensive signal processing at over 120 Gbps on the NVIDIA IGX platform.
- At Diamond Light Source, a world-renowned synchrotron in the UK, developers used Holoscan and Jax-based Holoscan operators to easily connect Holoscan to existing Ptychography software libraries to speed up image processing and reconstruction.
“With Holoscan, we’ve created an end-to-end data streaming pipeline that enables live ptychographic image processing at the I08-1 beam line, considerably enriching the overall user interaction,” said Paul Quinn, Imaging and Microscopy Science group leader, Diamond Light Source.
Get started with Holoscan 0.6
The release of NVIDIA Holoscan 0.6 marks a significant milestone in the development of edge AI solutions, offering unprecedented scalability, flexibility, and performance. With its diverse range of applications and success stories, Holoscan is shaping the future of AI-enabled sensor processing at the edge, opening new possibilities for various industries worldwide.
To get started developing, see the NVIDIA Holoscan SDK.
Modern neural networks have achieved impressive performance across a variety of applications, such as language, mathematical reasoning, and vision. However, these networks often use large architectures that require lots of computational resources. This can make it impractical to serve such models to users, especially in resource-constrained environments like wearables and smartphones. A widely used approach to mitigate the inference costs of pre-trained networks is to prune them by removing some of their weights, in a way that doesn’t significantly affect utility. In standard neural networks, each weight defines a connection between two neurons. So after weights are pruned, the input will propagate through a smaller set of connections and thus requires less computational resources.
![]() |
Original network vs. a pruned network. |
Pruning methods can be applied at different stages of the network’s training process: post, during, or before training (i.e., immediately after weight initialization). In this post, we focus on the post-training setting: given a pre-trained network, how can we determine which weights should be pruned? One popular method is magnitude pruning, which removes weights with the smallest magnitude. While efficient, this method doesn’t directly consider the effect of removing weights on the network’s performance. Another popular paradigm is optimization-based pruning, which removes weights based on how much their removal impacts the loss function. Although conceptually appealing, most existing optimization-based approaches seem to face a serious tradeoff between performance and computational requirements. Methods that make crude approximations (e.g., assuming a diagonal Hessian matrix) can scale well, but have relatively low performance. On the other hand, while methods that make fewer approximations tend to perform better, they appear to be much less scalable.
In “Fast as CHITA: Neural Network Pruning with Combinatorial Optimization”, presented at ICML 2023, we describe how we developed an optimization-based approach for pruning pre-trained neural networks at scale. CHITA (which stands for “Combinatorial Hessian-free Iterative Thresholding Algorithm”) outperforms existing pruning methods in terms of scalability and performance tradeoffs, and it does so by leveraging advances from several fields, including high-dimensional statistics, combinatorial optimization, and neural network pruning. For example, CHITA can be 20x to 1000x faster than state-of-the-art methods for pruning ResNet and improves accuracy by over 10% in many settings.
Overview of contributions
CHITA has two notable technical improvements over popular methods:
- Efficient use of second-order information: Pruning methods that use second-order information (i.e., relating to second derivatives) achieve the state of the art in many settings. In the literature, this information is typically used by computing the Hessian matrix or its inverse, an operation that is very difficult to scale because the Hessian size is quadratic with respect to the number of weights. Through careful reformulation, CHITA uses second-order information without having to compute or store the Hessian matrix explicitly, thus allowing for more scalability.
- Combinatorial optimization: Popular optimization-based methods use a simple optimization technique that prunes weights in isolation, i.e., when deciding to prune a certain weight they don’t take into account whether other weights have been pruned. This could lead to pruning important weights because weights deemed unimportant in isolation may become important when other weights are pruned. CHITA avoids this issue by using a more advanced, combinatorial optimization algorithm that takes into account how pruning one weight impacts others.
In the sections below, we discuss CHITA’s pruning formulation and algorithms.
A computation-friendly pruning formulation
There are many possible pruning candidates, which are obtained by retaining only a subset of the weights from the original network. Let k be a user-specified parameter that denotes the number of weights to retain. Pruning can be naturally formulated as a best-subset selection (BSS) problem: among all possible pruning candidates (i.e., subsets of weights) with only k weights retained, the candidate that has the smallest loss is selected.
Solving the pruning BSS problem on the original loss function is generally computationally intractable. Thus, similar to previous work, such as OBD and OBS, we approximate the loss with a quadratic function by using a second-order Taylor series, where the Hessian is estimated with the empirical Fisher information matrix. While gradients can be typically computed efficiently, computing and storing the Hessian matrix is prohibitively expensive due to its sheer size. In the literature, it is common to deal with this challenge by making restrictive assumptions on the Hessian (e.g., diagonal matrix) and also on the algorithm (e.g., pruning weights in isolation).
CHITA uses an efficient reformulation of the pruning problem (BSS using the quadratic loss) that avoids explicitly computing the Hessian matrix, while still using all the information from this matrix. This is made possible by exploiting the low-rank structure of the empirical Fisher information matrix. This reformulation can be viewed as a sparse linear regression problem, where each regression coefficient corresponds to a certain weight in the neural network. After obtaining a solution to this regression problem, coefficients set to zero will correspond to weights that should be pruned. Our regression data matrix is (n x p), where n is the batch (sub-sample) size and p is the number of weights in the original network. Typically n << p, so storing and operating with this data matrix is much more scalable than common pruning approaches that operate with the (p x p) Hessian.
Scalable optimization algorithms
CHITA reduces pruning to a linear regression problem under the following sparsity constraint: at most k regression coefficients can be nonzero. To obtain a solution to this problem, we consider a modification of the well-known iterative hard thresholding (IHT) algorithm. IHT performs gradient descent where after each update the following post-processing step is performed: all regression coefficients outside the Top-k (i.e., the k coefficients with the largest magnitude) are set to zero. IHT typically delivers a good solution to the problem, and it does so iteratively exploring different pruning candidates and jointly optimizing over the weights.
Due to the scale of the problem, standard IHT with constant learning rate can suffer from very slow convergence. For faster convergence, we developed a new line-search method that exploits the problem structure to find a suitable learning rate, i.e., one that leads to a sufficiently large decrease in the loss. We also employed several computational schemes to improve CHITA’s efficiency and the quality of the second-order approximation, leading to an improved version that we call CHITA++.
Experiments
We compare CHITA’s run time and accuracy with several state-of-the-art pruning methods using different architectures, including ResNet and MobileNet.
Run time: CHITA is much more scalable than comparable methods that perform joint optimization (as opposed to pruning weights in isolation). For example, CHITA’s speed-up can reach over 1000x when pruning ResNet.
Post-pruning accuracy: Below, we compare the performance of CHITA and CHITA++ with magnitude pruning (MP), Woodfisher (WF), and Combinatorial Brain Surgeon (CBS), for pruning 70% of the model weights. Overall, we see good improvements from CHITA and CHITA++.
![]() |
Post-pruning accuracy of various methods on ResNet20. Results are reported for pruning 70% of the model weights. |
![]() |
Post-pruning accuracy of various methods on MobileNet. Results are reported for pruning 70% of the model weights. |
Next, we report results for pruning a larger network: ResNet50 (on this network, some of the methods listed in the ResNet20 figure couldn’t scale). Here we compare with magnitude pruning and M-FAC. The figure below shows that CHITA achieves better test accuracy for a wide range of sparsity levels.
![]() |
Test accuracy of pruned networks, obtained using different methods. |
Conclusion, limitations, and future work
We presented CHITA, an optimization-based approach for pruning pre-trained neural networks. CHITA offers scalability and competitive performance by efficiently using second-order information and drawing on ideas from combinatorial optimization and high-dimensional statistics.
CHITA is designed for unstructured pruning in which any weight can be removed. In theory, unstructured pruning can significantly reduce computational requirements. However, realizing these reductions in practice requires special software (and possibly hardware) that support sparse computations. In contrast, structured pruning, which removes whole structures like neurons, may offer improvements that are easier to attain on general-purpose software and hardware. It would be interesting to extend CHITA to structured pruning.
Acknowledgements
This work is part of a research collaboration between Google and MIT. Thanks to Rahul Mazumder, Natalia Ponomareva, Wenyu Chen, Xiang Meng, Zhe Zhao, and Sergei Vassilvitskii for their help in preparing this post and the paper. Also thanks to John Guilyard for creating the graphics in this post.
Deep neural networks (DNNs) are the go-to model for learning functions from data, such as image classifiers or language models. In recent years, deep models…
Deep neural networks (DNNs) are the go-to model for learning functions from data, such as image classifiers or language models. In recent years, deep models have become popular for representing the data samples themselves. For example, a deep model can be trained to represent an image, a 3D object, or a scene, an approach called Implicit Neural Representations. (See also Neural Radiance Fields and Instant NGP). Read on for a few examples of performing operations on a pretrained deep model for both DNNs-that-are-functions and DNNs-that-are-data.
Suppose you have a dataset of 3D objects represented using Implicit Neural Representations (INRs) or Neural Radiance Fields (NeRFs). Very often, you may wish to “edit” the objects to change their geometry or fix errors and abnormalities. For example, to remove a handle of a cup or make all car wheels more symmetric than was reconstructed by the NeRF.
Unfortunately, a major challenge with using INRs and NeRFs is that they must be rendered before editing. Indeed, editing tools rely on rendering the objects and directly fine-tuning the INR or NeRF parameters. See, for example, 3D Neural Sculpting (3DNS): Editing Neural Signed Distance Functions. It would have been much more efficient to change the weights of the NeRF model directly without rendering it back to 3D space.
As a second example, consider a trained image classifier. In some cases, you may want to apply certain transformations to the classifier. For example, you may want to take a classifier trained in snowy weather and make it accurate for sunny images. This is an instance of a domain adaptation problem.
However, unlike traditional domain adaptation approaches, the setting focuses on learning the general operation of mapping a function (classifier) from one domain to another, rather than transferring a specific classifier from the source domain to the target domain.
Neural networks that process other neural networks
The key question our team raises is whether neural networks can learn to perform these operations. We seek a special type of neural network “processor” that can process the weights of other neural networks.
This, in turn, raises the important question of how to design neural networks that can process the weights of other neural networks. The answer to this question is not that simple.
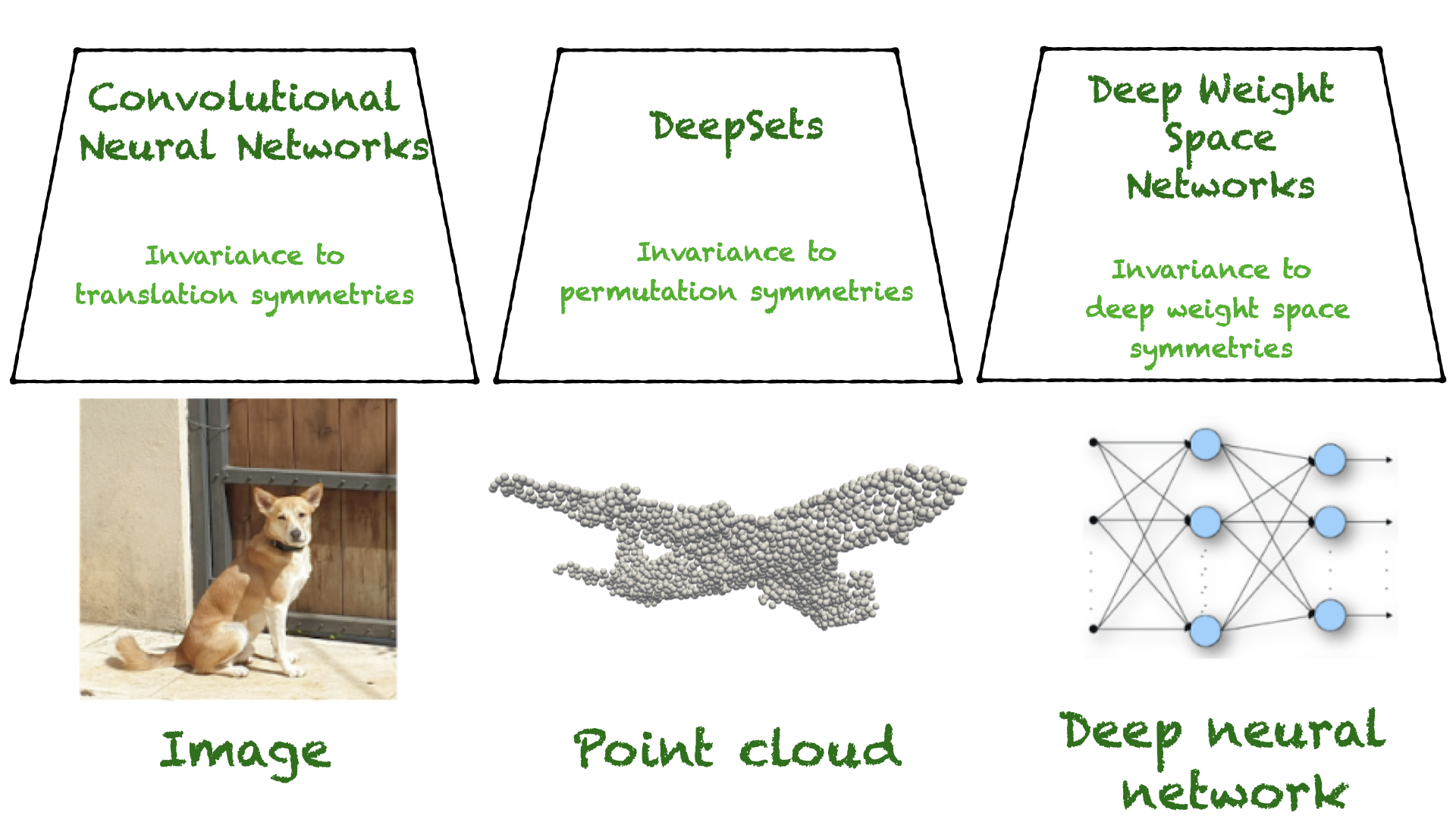
Previous work on processing deep weight spaces
The simplest way to represent the parameters of a deep network is to vectorize all weights (and biases) as a simple flat vector. Then, apply a fully connected network, also known as a multilayer perceptron (MLP).
Several studies have attempted this approach, showing that this method can predict the test performance of input neural networks. See Classifying the Classifier: Dissecting the Weight Space of Neural Networks, Hyper-Representations: Self-Supervised Representation Learning on Neural Network Weights for Model Characteristic Prediction, and Predicting Neural Network Accuracy from Weights.
Unfortunately, this approach has a major shortcoming because the space of neural network weights has a complex structure (explained more fully below). Applying an MLP to a vectorized version of all parameters ignores that structure and, as a result, hurts generalization. This effect is similar to other types of structured inputs, like images. This case works best with a deep network that is not sensitive to small shifts of an input image.
The solution is to use convolutional neural networks. They are designed in a way that is largely “blind” to the shifting of an image and, as a result, can generalize to new shifts that were not observed during training.
Here, we want to design deep architectures that follow the same idea, but instead of taking into account image shifts, we want to design architectures that are not sensitive to other transformations of model weights, as we describe below.
Specifically, a key structural property of neural networks is that their weights can be permuted while they still compute the same function. Figure 2 illustrates this phenomenon. This important property is overlooked when applying a fully connected network to vectorized weights.
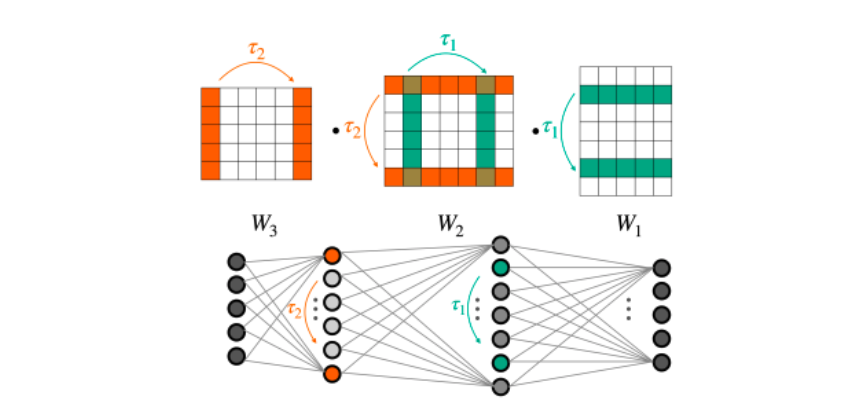
Unfortunately, a fully connected network that operates on flat vectors sees all these equivalent representations as different. This makes it much harder for the network to generalize across all such (equivalent) representations.
A brief introduction to symmetries and equivariant architectures
Fortunately, the preceding MLP limitations have been extensively studied in a subfield of machine learning called Geometric Deep Learning (GDL). GDL is about learning objects while being invariant to a group of transformations of these objects, like shifting images or permuting sets. This group of transformations is often called a symmetry group.
In many cases, learning tasks are invariant to these transformations. For example, finding the class of a point cloud should be independent of the order by which points are given the network because that order is irrelevant.
In other cases, like point cloud segmentation, every point in the cloud is assigned a class to which part of the object it belongs to. In these cases, the order of output points must change in the same way if the input is permuted. Such functions, whose output transforms according to the input transformation, are called equivariant functions.
More formally, for a group of transformations G, a function L: V → W is called G-equivariant if it commutes with the group action, namely L(gv) = gL(v) for all v ∈ V, g ∈ G. When L(gv) = L(v) for all g∈ G, L is called an invariant function.
In both cases, invariant and equivariant functions, restricting the hypothesis class is highly effective, and such symmetry-aware architectures offer several advantages due to their meaningful inductive bias. For example, they often have better sample complexity and fewer parameters. In practice, these factors result in significantly better generalization.
Symmetries of weight spaces
This section explains the symmetries of deep weight spaces. One might ask the question: Which transformations can be applied to the weights of MLPs, such that the underlying function represented by the MLP is not changed?
One specific type of transformation, called neuron permutations, is the focus here. Intuitively, when looking at a graph representation of an MLP (such as the one in Figure 2), changing the order of the neurons at a certain intermediate layer does not change the function. Moreover, the reordering procedure can be done independently for each internal layer.
In more formal terms, an MLP can be represented using the following set of equations:
The weight space of this architecture is defined as the (linear) space that contains all concatenations of vectorized weights and biases . Importantly, in this setup, the weight space is the input space to the (soon-to-be-defined) neural networks.
So, what are the symmetries of weight spaces? Reordering the neurons can be formally modeled as an application of a permutation matrix to the output of one layer and an application of the same permutation matrix to the next layer. Formally, a new set of parameters can be defined by the following equations:
The new set of parameters is different, but it is easy to see that such transformations do not change the function represented by the MLP. This is because the two permutation matrices and
cancel each other (assuming an elementwise activation function like ReLU).
More generally, and as stated earlier, a different permutation can be applied to each layer of the MLP independently. This means that the following more general set of transformations will not change the underlying function. Think about these as symmetries of weight spaces.
Here, represents permutation matrices. This observation was made more than 30 years ago by Hecht-Nielsen in On the Algebraic Structure of Feedforward Network Weight Spaces. A similar transformation can be applied to the biases of the MLP.
Building Deep Weight Space Networks
Most equivariant architectures in the literature follow the same recipe: a simple equivariant layer is defined, and the architecture is defined as a composition of such simple layers, possibly with pointwise nonlinearity between them.
A good example of such a construction is CNN architecture. In this case, the simple equivariant layer performs a convolution operation, and the CNN is defined as a composition of multiple convolutions. DeepSets and many GNN architectures follow a similar approach. For more information, see Weisfeiler and Leman Go Neural: Higher-Order Graph Neural Networks and Invariant and Equivariant Graph Networks.
When the task at hand is invariant, it is possible to add an invariant layer on top of the equivariant layers with an MLP, as illustrated in Figure 3.
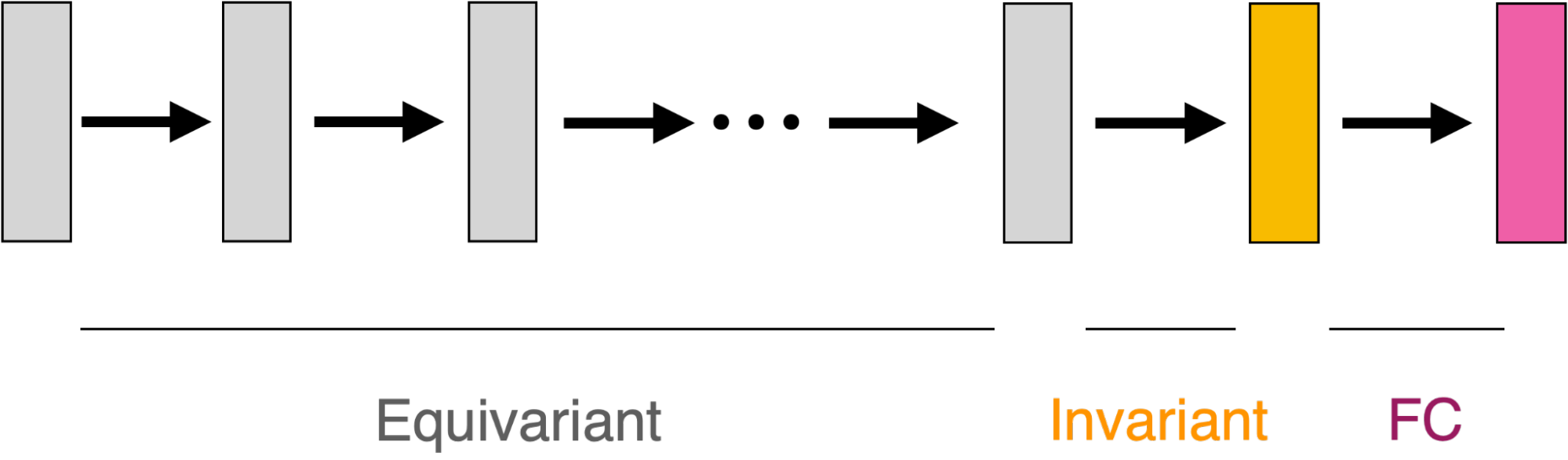
We follow this recipe in our paper, Equivariant Architectures for Learning in Deep Weight Spaces. Our main goal is to identify simple yet effective equivariant layers for the weight-space symmetries defined above. Unfortunately, characterizing spaces of general equivariant functions can be challenging. As with some previous studies (such as Deep Models of Interactions Across Sets), we aim to characterize the space of all linear equivariant layers.
We have developed a new method to characterize linear equivariant layers that is based on the following observation: the weight space V is a concatenation of simpler spaces that represent each weight matrix V=⊕Wi. (Bias terms are omitted for brevity).
This observation is important, as it enables writing any linear layer as a block matrix whose (i,j)-th block is a linear equivariant layer between
and
. This block structure is illustrated in Figure 4.
But how can we find all instances of ? Our paper lists all the possible cases and shows that some of these layers were already characterized in previous work. For example,
for internal layers was characterized in Deep Models of Interactions Across Sets.
Remarkably, the most general equivariant linear layer in this case is a generalization of the well-known deep sets layer that uses only four parameters. For other layers, we propose parameterizations based on simple equivariant operations such as pooling, broadcasting, and small fully connected layers, and show that they can represent all linear equivariant layers.
Figure 4 shows the structure of L, which is a block matrix between specific weight spaces. Each color represents a different type of layer. are in red. Each block maps a specific weight matrix to another weight matrix. This mapping is parameterized in a way that relies on the positions of the weight matrices in the network.

The layer is implemented by computing each block independently and then summing the results for each row. Our paper covers some additional technicalities, like processing the bias terms and supporting multiple input and output features.
We call these layers Deep Weight Space Layers (DWS Layers), and the networks constructed from them Deep Weight Space Networks (DWSNets). We focus here on DWSNets that take MLPs as input. For more details on extensions to CNNs and transformers, see Appendix H in Equivariant Architectures for Learning in Deep Weight Spaces.
The expressive power of Deep Weight Space Networks
Restricting our hypothesis class to a composition of simple equivariant functions may unintentionally impair the expressive power of equivariant networks. This has been widely studied in the graph neural networks literature cited above. Our paper shows that DWSNets can approximate feed-forward operations on input networks—a step toward understanding their expressive power. We then show that DWS networks can approximate certain “nicely behaving” functions defined in the MLP function space.
Experiments
DWSNets are evaluated in two families of tasks. First, taking input networks that represent data, like INRs. Second, taking input networks that represent standard I/O mappings such as image classification.
Experiment 1: INR classification
This setup classifies INRs based on the image they represent. Specifically, it involves training INRs to represent images from MNIST and Fashion-MNIST. The task is to have the DWSNet recognize the image content, like the digit in MNIST, using the weights of these INRs as input. The results show that our DWSNet architecture greatly outperforms the other baselines.
Method | MNIST INR | Fashion-MNIST INR |
MLP | 17.55% +- 0.01 | 19.91% +- 0.47 |
MLP + Perm. aug | 29.26% +- 0.18 | 22.76% +- 0.13 |
MLP + Alignment | 58.98% +- 0.52 | 47.79% +- 1.03 |
INR2Vec (Architecture) | 23.69% +- 0.10 | 22.33% +- 0.41 |
Transformer | 26.57% +- 0.18 | 26.97% +- 0.33 |
DWSNets (ours) | 85.71% +- 0.57 | 67.06% +- 0.29 |
Importantly, classifying INRs to the classes of images they represent is significantly more challenging than classifying the underlying images. An MLP trained on MNIST images can achieve near-perfect test accuracy. However, an MLP trained on MNIST INRs achieves poor results.
Experiment 2: Self-supervised learning on INRs
The goal here is to embed neural networks (specifically, INRs) into a semantic coherent low-dimensional space. This is an important task, as a good low-dimensional representation can be vital for many downstream tasks.
Our data consists of INRs fitted to sine waves of the form asin(bx), where a, b are sampled from a uniform distribution on the interval [0,10]. As the data is controlled by these two parameters, the dense representation should extract this underlying structure.

A SimCLR-like training procedure and objective are used to generate random views from each INR by adding Gaussian noise and random masking. Figure 4 presents a 2D TSNE plot of the resulting space. Our method, DWSNet, nicely captures the underlying characteristics of the data while competing approaches struggle.
Experiment 3: Adapting pretrained networks to new domains
This experiment shows how to adapt a pretrained MLP to a new data distribution without retraining (zero-shot domain adaptation). Given input weights for an image classifier, the task is to transform its weights into a new set of weights that performs well on a new image distribution (the target domain).
At test time, the DWSnet receives a classifier and adapts it to the new domain in a single forward pass. The CIFAR10 dataset is the source domain and a corrupted version of it is the target domain (Figure 6).
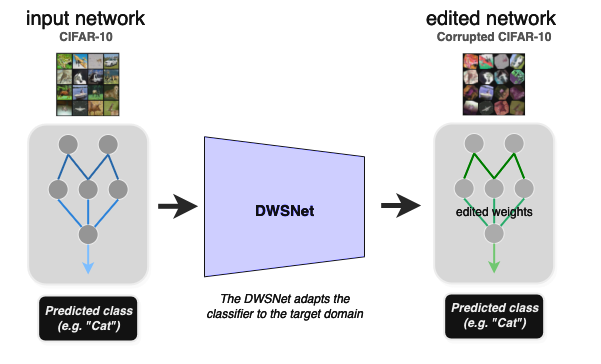
The results are presented in Table 2. Note that at test time the model should generalize to unseen image classifiers, as well as unseen images.
Method | CIFAR10->CIFAR10 corrupted |
No adaptation | 60.92% +- 0.41 |
MLP | 64.33% +- 0.36 |
MLP + permutation augmentation | 64.69% +- 0.56 |
MLP + alignment | 67.66% +- 0.90 |
INR2Vec (architecture) | 65.69% +- 0.41 |
Transformer | 61.37% +- 0.13 |
DWSNets (ours) | 71.36% +- 0.38 |
Future research directions
The ability to apply learning techniques to deep-weight spaces offers many new research directions. First, finding efficient data augmentation schemes for training functions over weight spaces has the potential to improve DWSNets generalization. Second, it is natural to study how to incorporate permutation symmetries for other types of input architectures and layers, like skip connections or normalization layers. Finally, it would be useful to extend DWSNets to real-world applications like shape deformation and morphing, NeRF editing, and model pruning. Read the full ICML 2023 paper, Equivariant Architectures for Learning in Deep Weight Spaces.
Several papers are closely related to the work presented here, and we encourage interested readers to check them. First, the paper Permutation Equivariant Neural Functionals provides a similar formulation to the problem discussed here but from a different view. A follow-up study, Neural Functional Transformers, suggests using attention mechanisms instead of simple sum/mean aggregations in linear equivariant layers. Finally, the paper Neural Networks Are Graphs! Graph Neural Networks for Equivariant Processing of Neural Networks proposes to model the input neural network as a weighted graph and applying GNNs to process the weight space.
NVIDIA interns around the globe wrapped up a week of “Our Founders Edition” celebrations — a nod to a special line of GeForce cards — which featured company lore and talks from founders Jensen Huang and Chris Malachowsky. Read article >
NVIDIA Jetson Orin is the best-in-class embedded AI platform. The Jetson Orin SoC module has the NVIDIA Ampere architecture GPU at its core but there is a lot…
NVIDIA Jetson Orin is the best-in-class embedded AI platform. The Jetson Orin SoC module has the NVIDIA Ampere architecture GPU at its core but there is a lot more compute on the SoC:
- A dedicated deep learning inference engine in the Deep Learning Accelerator (DLA) for deep learning workloads
- The Programmable Vision Accelerator (PVA) engine for image processing and computer vision algorithms
- The Multi-Standard Video Encoder (NVENC) and Multi-Standard Video Decoder (NVDEC)
The NVIDIA Orin SoC is powerful, with 275 peak AI TOPs, making it the best embedded and automotive AI platform. Did you know that almost 40% of these AI TOPs come from the two DLAs on NVIDIA Orin? While NVIDIA Ampere GPUs have the best-in-class throughput, the second-generation DLA has the best-in-class power efficiency. As applications of AI have rapidly grown in recent years, so has the demand for more efficient computing. This is especially true on the embedded side where power efficiency is always a key KPI.
That’s where DLA comes in. DLA is designed specifically for deep learning inference and can perform compute-intensive deep learning operations like convolutions much more efficiently than a CPU.
When integrated into an SoC as on Jetson AGX Orin or NVIDIA DRIVE Orin, the combination of GPU and DLA provides a complete solution for your embedded AI applications. In this post, we discuss the Deep Learning Accelerator to help you stop missing out. We cover a couple of case studies in automotive and robotics to demonstrate how DLA enables AI developers to add more functionality and performance to their applications. Finally, we look at how vision AI developers can use the DeepStream SDK to build application pipelines that use DLA and the entire Jetson SoC for optimal performance.
But first, here are some key performance indicators that DLA has a significant impact on.
Key performance indicators
When you are designing your application, you have a few key performance indicators or KPIs to meet. Often it’s a design tradeoff, for example, between max performance and power efficiency, and this requires the development team to carefully analyze and design their application to use the different IPs on the SoC.
If the key KPI for your application is latency, you must pipeline the tasks within your application under a certain latency budget. You can use DLA as an additional accelerator for tasks that are parallel to more compute-intensive tasks running on GPU. The DLA peak performance contributes between 38% and 74% to the NVIDIA Orin total deep learning (DL) performance, depending on the power mode.
Power mode: MAXN | Power mode: 50W | Power mode: 30W | Power mode: 15W | |
GPU sparse INT8 peak DL performance | 171 TOPs | 109 TOPs | 41 TOPs | 14 TOPs |
2x DLA sparse INT8 peak performance | 105 TOPs | 92 TOPs | 90 TOPs | 40 TOPs |
Total NVIDIA Orin peak INT8 DL performance | 275 TOPs | 200 TOPs | 131 TOPs | 54 TOPs |
Percentage: DLA peak INT8 performance of total NVIDIA Orin peak DL INT8 performance | 38% | 46% | 69% | 74% |
The DLA TOPs of the 30 W and 50 W power modes on Jetson AGX Orin 64GB are comparable to the maximum clocks on NVIDIA DRIVE Orin platforms for Automotive.
If power is one of your key KPIs, then you should consider DLA to take advantage of its power efficiency. DLA performance per watt is on average 3–5x more compared to the GPU, depending on the power mode and the workload. The following charts show performance per watt for three models representing common use cases.
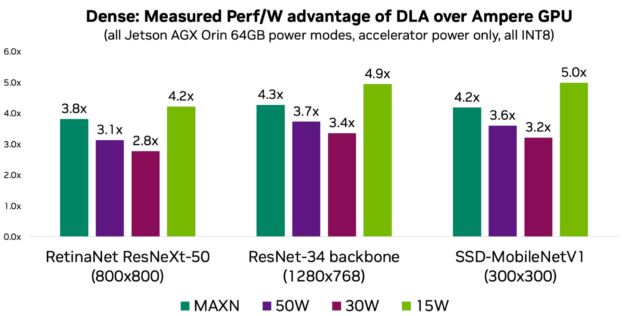
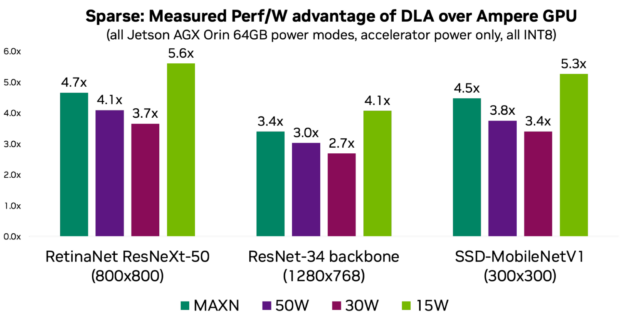
Put differently, without DLA’s power efficiency, it would not be possible to achieve up to 275 peak DL TOPs on NVIDIA Orin at a given platform power budget. For more information and measurements for more models, see the DLA-SW GitHub repo.
Here are some case studies within NVIDIA on how we used the AI compute offered by DLA: Automotive and Robotics
Case study: Automotive
NVIDIA DRIVE AV is the end-to-end autonomous driving solution stack for automotive OEMs to add autonomous driving and mapping features to their automotive product portfolio. It includes perception, mapping, and planning layers, as well as diverse DNNs trained on high-quality, real-world driving data.
Engineers from the NVIDIA DRIVE AV team work on designing and optimizing the perception, mapping, and planning pipelines by leveraging the entire NVIDIA Orin SoC platform. Given the large number of neural networks and other non-DNN tasks to process in the self-driving stack, they rely on DLA as the dedicated inference engine on the NVIDIA Orin SoC, to run DNN tasks. This is critical because the GPU compute is reserved to process non-DNN tasks. Without DLA compute, the team would not meet their KPIs.
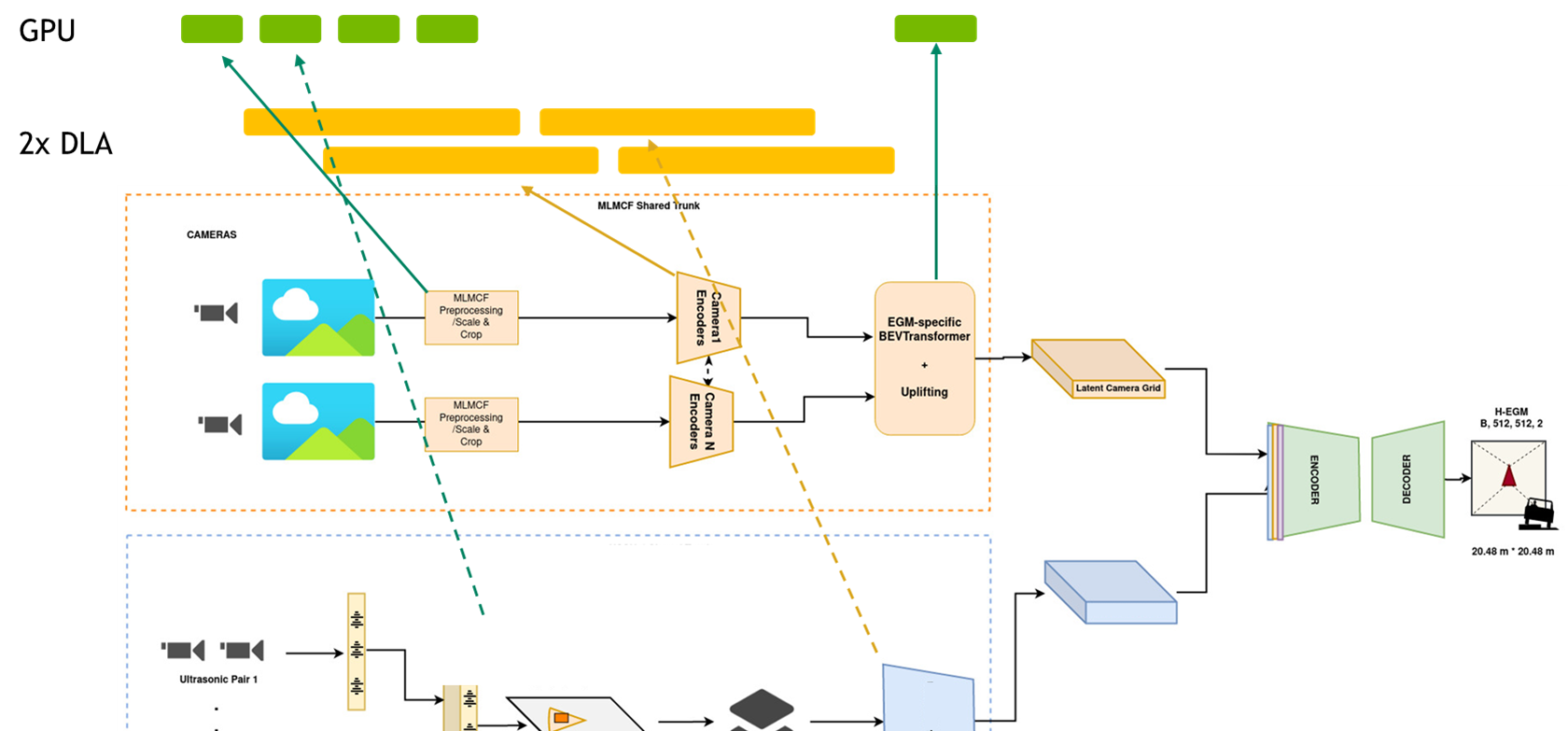
For more information, see Near-Range Obstacle Perception with Early Grid Fusion.
For instance, for the perception pipeline, they have inputs from eight different camera sensors and the latency of the entire pipeline must be lower than a certain threshold. The perception stack is DNN-heavy and accounts for more than 60% of all the compute.
To meet these KPIs, parallel pipeline tasks are mapped to GPU and DLA, where almost all the DNNs are running on DLAs and non-DNN tasks on the GPU to achieve the overall pipeline latency target. The outputs are then consumed sequentially or in parallel by other DNNs in other pipelines like mapping and planning. You may view the pipelines as a giant graph with tasks running in parallel on GPU and DLA. Using DLA, the team reduced their latency 2.5x.
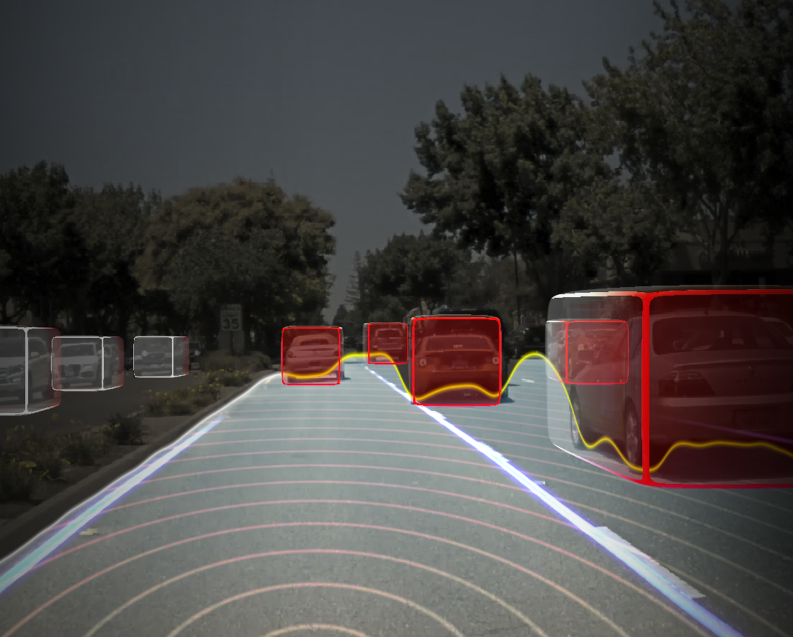
“Leveraging the entire SoC, especially the dedicated deep learning inference engine in DLA, is enabling us to add significant functionality to our software stack while still meeting latency requirements and KPI targets. This is only possible with DLA,” said Abhishek Bajpayee, engineering manager of the Autonomous Driving team at NVIDIA.
Case study: Robotics
NVIDIA Isaac is a powerful, end-to-end platform for the development, simulation, and deployment of AI-enabled robots used by robotics developers. For mobile robots in particular, the available DL compute, deterministic latencies, and battery endurance are important factors. This is why mapping DL inference to DLA is important.
A team of engineers from the NVIDIA Isaac team have developed a library for proximity segmentation using DNNs. Proximity segmentation can be used to determine whether an obstacle is within a proximity field and to avoid collisions with obstacles during navigation. They implemented the BI3D network on DLA that performs binary depth classification from a stereo camera.
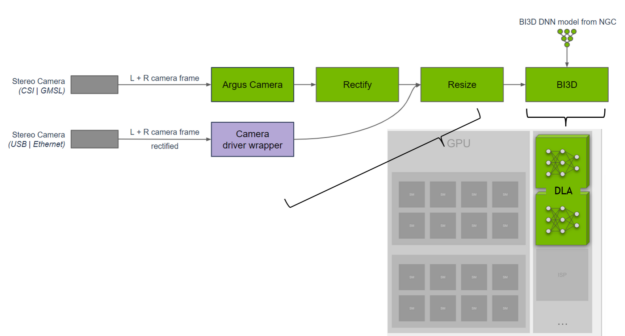
Figure 5. Proximity segmentation pipeline NEEDS ALT TEXT
A key KPI is ensuring real-time 30-fps detection from a stereo camera input. The NVIDIA Isaac team distributes the tasks across the SoC and uses DLA for the DNNs, while providing functional safety diversity in hardware and software from what is run on the GPU. For more information, see NVIDIA Isaac ROS Proximity Segmentation.
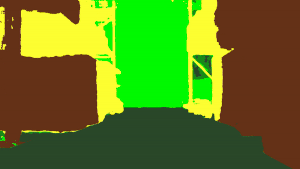
“We use TensorRT on DLA for DNN inference to provide hardware diversity from the GPU improving fault tolerance while offloading the GPU for other tasks. DLA delivers ~46 fps on Jetson AGX Orin for BI3D, which consists of three DNNs, providing low 30 ms of latency for our robotics applications,” said Gordon Grigor, vice president of Robotics Platform Software at NVIDIA.
NVIDIA DeepStream for DLA
The quickest way to explore DLA is through the NVIDIA DeepStream SDK, a complete streaming analytics toolkit.
If you are a vision AI developer building AI-powered applications to analyze video and sensor data, the DeepStream SDK enables you to build optimal end-to-end pipelines. For cloud or edge use cases such as retail analytics, parking management, managing logistics, optical inspection, robotics, and sports analytics, DeepStream enables the use of the entire SoC and specifically DLA with little effort.
For instance, you can use the pretrained models from the Model Zoo highlighted in the following table to run on DLA. Running these networks on DLA is as simple as setting a flag. For more information, see Using DLA for inference.
Model arch | Inference resolution | GPU FPS | DLA1 + DLA2 FPS | GPU + DLA1 + DLA2 FPS |
PeopleNet-ResNet18 | 960x544x3 | 218 | 128 | 346 |
PeopleNet-ResNet34 (v2.3) | 960x544x3 | 169 | 94 | 263 |
PeopleNet-ResNet34 (v2.5 unpruned) | 960x544x3 | 79 | 46 | 125 |
TrafficCamNet | 960x544x3 | 251 | 174 | 425 |
DashCamNet | 960x544x3 | 251 | 172 | 423 |
FaceDetect-IR | 384x240x3 | 1407 | 974 | 2381 |
VehicleMakeNet | 224x224x3 | 2434 | 1166 | 3600 |
VehicleTypeNet | 224x224x3 | 1781 | 1064 | 2845 |
FaceDetect (pruned) | 736x416x3 | 395 | 268 | 663 |
License Plate Detection | 640x480x3 | 784 | 388 | 1172 |
Get started with the Deep Learning Accelerator
Ready to dive in? For more information, see the following resources:
- Jetson DLA tutorial demonstrates a basic DLA workflow to help you in getting started with deploying a DNN to DLA.
- The DLA-SW GitHub repo has a collection of reference networks that you can use to explore running DNNs on your Jetson Orin DLA.
- The samples page has other examples and resources on how to use DLA to get the most out of your Jetson SoC.
- The DLA forum has ideas and feedback from other users.
Replit aims to empower the next billion software creators. In this week’s episode of NVIDIA’s AI Podcast, host Noah Kraviz dives into a conversation with Replit CEO Amjad Masad. Read article >