Expanding their longstanding alliance, Oracle and NVIDIA today announced a multi-year partnership to help customers solve business challenges with accelerated computing and AI. The collaboration aims to bring the full NVIDIA accelerated computing stack — from GPUs to systems to software — to Oracle Cloud Infrastructure (OCI).
Editor’s note: This post is part of our weekly In the NVIDIA Studio series, which celebrates featured artists, offers creative tips and tricks, and demonstrates how NVIDIA Studio technology improves creative workflows. In the coming weeks, we’ll be deep diving on new GeForce RTX 40 Series GPU features, technologies and resources, and how they dramatically Read article >
The post Adobe MAX Kicks Off With Creative App Updates and 3D Artist Anna Natter Impresses This Week ‘In the NVIDIA Studio’ appeared first on NVIDIA Blog.
Vehicle appraisals are getting souped up with a GPU-accelerated AI overhaul. ProovStation, a four-year-old startup based in Lyon, France, is taking on the ambitious computer-vision quest of automating vehicle inspection and repair estimates, aiming AI-driven super-high-resolution stations at businesses worldwide. It recently launched three of its state-of-the-art vehicle inspection scanners at French retail giant Carrefour’s Read article >
The post Souped-Up Auto Quotes: ProovStation Delivers GPU-Driven AI Appraisals appeared first on NVIDIA Blog.
The Metaverse is providing new opportunities for everyone—for artists building content across multiple 3D tools, for developers building AI trained in…
The Metaverse is providing new opportunities for everyone—for artists building content across multiple 3D tools, for developers building AI trained in virtual worlds, and for enterprises building digital twin simulations of their industrial processes. NVIDIA Omniverse is a computing platform for building and navigating virtual worlds. Based on Pixar’s Universal Scene Description (USD), Omniverse enables individuals and teams to build custom 3D pipelines and simulate large-scale virtual worlds faster than ever.
NVIDIA Deep Learning Institute (DLI) has launched three new self-paced, hands-on, 90-minute courses for developers and technical artists who build tools to create 3D worlds. In these courses, you will learn how to build advanced tools connected to the Omniverse platform that extend and enhance the 3D tools you already know and love. Click the links below to enroll, and read on for more details.
- Build Beautiful, Custom UI for 3D Tools on NVIDIA Omniverse
- Easily Develop Advanced 3D Layout Tools on NVIDIA Omniverse
- How to Build Custom 3D Scene Manipulator Tools on NVIDIA Omniverse
To learn more about these courses, we caught up with Paul Cutsinger, director of Omniverse Exchange at NVIDIA, whose team designed the courses.
Why did you create these training courses?
People have been building virtual worlds for the entertainment industry for decades, but we are at a time in history that’s especially exciting because everything that is around us, everything that is physically real in the world, is going to first be created digitally. In fact, people talk about digital twins, but really, the physical things are actually the twins of the digital world.
Virtual worlds are essential for the next era of industries. Building virtual worlds is a complex team sport. Making true simulations of the world requires scalable, physically accurate 3D simulations of supply chains, factories, warehouses, physical stores, distribution centers, and so on. Simulating the associated products and processes is an enormous task. And today’s 3D workflows are tedious and way too slow for designers, artists, and engineers.
For the Metaverse and virtual worlds to come about, we need to have tools, lots and lots of tools. Tools built for specific tasks. Tools that are small and easily composable in workflows. These three new DLI courses are the basis for Metaverse tool makers. They provide a foundation for building Omniverse tools.
Tell us a bit about each course.
In the first course, Build Beautiful, Custom UI for 3D Tools on NVIDIA Omniverse, you will learn the basic user interface (UI) elements of Omniverse and use them to build custom interfaces for a typical tool. We will show you how to build a UI that integrates stylistically with Omniverse while being tailored to your use case for a seamless user experience. In addition, you will learn how to use Omniverse Kit to produce extensions.
The second course, Easily Develop Advanced 3D Layout Tools on NVIDIA Omniverse, enables you to modify a scene. You’ll learn how to programmatically work with the USD API to find, create, move, and arrange items in the scene that you’re building. The course provides a good practical, hands-on orientation to USD.
The third course, How to Build Custom 3D Manipulator Tools on NVIDIA Omniverse, teaches you how to build manipulator tools that enable you to add UI affordances directly on objects inside a scene or in the viewport. For example, if I want to resize an object, I can go over to some property panel somewhere, find the scale slider, and move it. But if that task is an integral part of my solution, I can create a widget to manipulate the object directly. So the third course teaches you how to build scene manipulators so artists can more easily create and modify scenes.
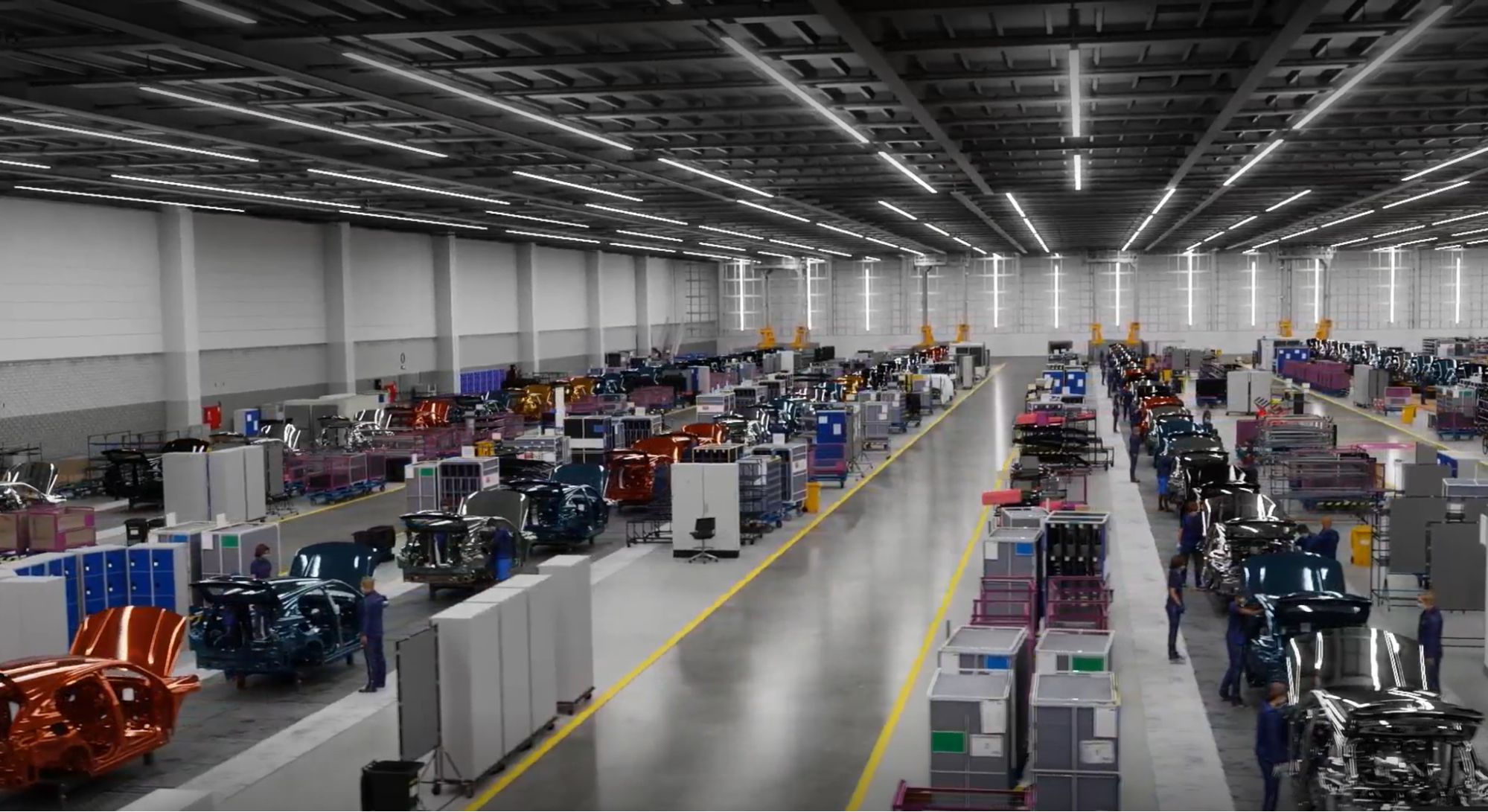
Who should take this training?
These courses are for developers, engineers, technical artists, tool-building companies, hobbyists, and researchers who want to develop Python-based tools for the Metaverse.
With Omniverse, I believe, we are going to see a whole new wave of creativity and levels of collaboration we’ve never seen before. But for that to happen, we need more tools to enable content creators. This training is designed for those who want to build tools for digital twins, virtual worlds, and 3D workflows. There is a tremendous opportunity for tool builders right now and Omniverse enables the building of plug-ins in an agnostic way. You won’t have to build the tool for each and every connected app. Just build once and use in Omniverse.
I’d also recommend this training for folks who are transitioning from artist to developer or developer to artist. This is a really great way to grow from one to the other. If you’re an artist, become a technical artist. If you’re a technical person, become a technical artist. Level up your skill set.
What are some examples of the kinds of tools being built?
We launched these courses at SIGGRAPH and held a contest to see what kind of extensions students would come up with. The #ExtendOmniverse contestants could submit entries to one of three categories that aligned with the courses:
- Omni.ui with Omniverse Kit
- Layout and scene authoring tools
- Scene modifier and manipulator tools
The results were phenomenal. We had extensions submitted from developers all over the world. The grand prize went to Yizhou Zhao who built an IndoorKit extension for robotics to reduce the effort required to set up tasks, such as picking up an object. Visit yizhouzhao/VRKitchen2.0-IndoorKit on GitHub for details. With this extension, and the help of Omniverse, it is easier to set up tasks for robots in a photorealistic and physics-reliable manner. You can read more about the Omniverse contest results in the article, To the Metaverse and Beyond: Meet the Omniverse Contest Finalists Building the Tools for 3D Worlds.

What I love the most about Omniverse is the reaction from people who first experience it. To quote Yizhou, “The moment I opened Omniverse, my knowledge and skills in math, physics, computational geometry, 3D design, animation, and deep learning came alive.”
As I mentioned earlier, I’m excited because I think we are unlocking a whole new wave of creativity in the world with Omniverse.
Visit NVIDIA Deep Learning Institute for a complete list of courses and workshops.
Efficient processing of string data is vital for many data science applications. To extract valuable information from string data, RAPIDS libcudf provides…
Efficient processing of string data is vital for many data science applications. To extract valuable information from string data, RAPIDS libcudf provides powerful tools for accelerating string data transformations. libcudf is a C++ GPU DataFrame library used for loading, joining, aggregating, and filtering data.
In data science, string data represents speech, text, genetic sequences, logging, and many other types of information. When working with string data for machine learning and feature engineering, the data must frequently be normalized and transformed before it can be applied to specific use cases. libcudf provides both general purpose APIs as well as device-side utilities to enable a wide range of custom string operations.
This post demonstrates how to skillfully transform strings columns with the libcudf general purpose API. You’ll gain new knowledge on how to unlock peak performance using custom kernels and libcudf device-side utilities. This post also walks you through examples of how to best manage GPU memory and efficiently construct libcudf columns to speed up your string transformations.
Introducing Arrow format for strings columns
libcudf stores string data in device memory using Arrow format, which represents strings columns as two child columns: chars and offsets
(Figure 1).
The chars
column holds the string data as UTF-8 encoded character bytes that are stored contiguously in memory.
The offsets
column contains an increasing sequence of integers which are byte positions identifying the start of each individual string within the chars data array. The final offset element is the total number of bytes in the chars column. This means the size of an individual string at row i
is defined as (offsets[i+1]-offsets[i])
.
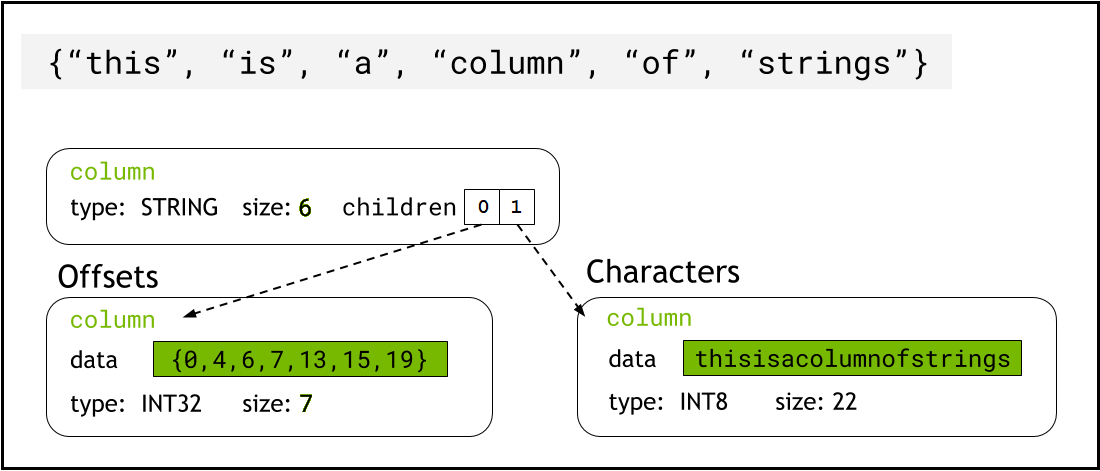
chars
and offsets
child columnsExample of string redaction function
To illustrate an example string transformation, consider a function that receives two input strings columns and produces one redacted output strings column.
The input data has the following form: a “names” column containing first and last names separated by a space and a “visibilities” column containing the status of “public” or “private.”
We propose the “redact” function that operates on the input data to produce output data consisting of the first initial of the last name followed by a space and the entire first name. However, if the corresponding visibility column is “private” then the output string should be fully redacted as “X X.”
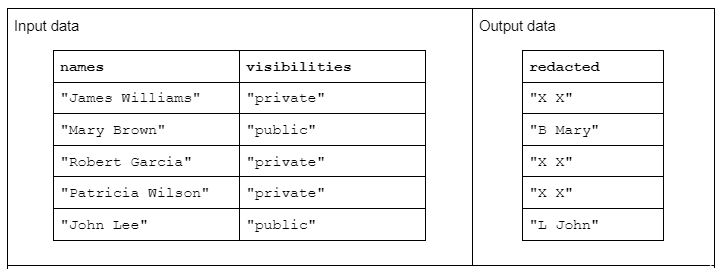
Transforming strings with the libcudf API
First, string transformation can be accomplished using the libcudf strings API. The general purpose API is an excellent starting point and a good baseline for comparing performance.
The API functions operate on an entire strings column, launching at least one kernel per function and assigning one thread per string. Each thread handles a single row of data in parallel across the GPU and outputs a single row as part of a new output column.
To complete the redact example function using the general purpose API, follow these steps:
- Convert the “visibilities” strings column into a Boolean column using
contains
- Create a new strings column from the names column by copying “X X” whenever the corresponding row entry in the boolean column is “false”
- Split the “redacted” column into first name and last name columns
- Slice the first character of the last names as the last name initials
- Build the output column by concatenating the last initials column and the first names column with space (” “) separator.
// convert the visibility label into a boolean
auto const visible = cudf::string_scalar(std::string("public"));
auto const allowed = cudf::strings::contains(visibilities, visible);
// redact names
auto const redaction = cudf::string_scalar(std::string("X X"));
auto const redacted = cudf::copy_if_else(names, redaction, allowed->view());
// split the first name and last initial into two columns
auto const sv = cudf::strings_column_view(redacted->view())
auto const first_last = cudf::strings::split(sv);
auto const first = first_last->view().column(0);
auto const last = first_last->view().column(1);
auto const last_initial = cudf::strings::slice_strings(last, 0, 1);
// assemble a result column
auto const tv = cudf::table_view({last_initial->view(), first});
auto result = cudf::strings::concatenate(tv, std::string(" "));
This approach takes about 3.5 ms on an A6000 with 600K rows of data. This example uses contains
, copy_if_else, split, slice_strings
and concatenate
to accomplish a custom string transformation. A profiling analysis with Nsight Systems shows that the split
function takes the longest amount of time, followed by slice_strings
and concatenate
.
Figure 2 shows profiling data from Nsight Systems of the redact example, showing end-to-end string processing at up to ~600 million elements per second. The regions correspond to NVTX ranges associated with each function. Light blue ranges correspond to periods where CUDA kernels are running.
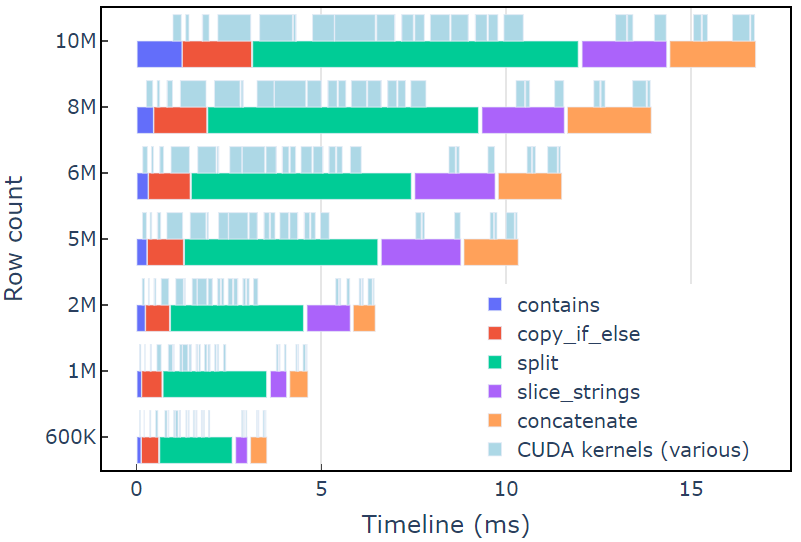
Transforming strings with a custom kernel
The libcudf strings API is a fast and efficient toolkit for transforming strings, but sometimes performance-critical functions need to run even faster. A key source of extra work in the libcudf strings API is the creation of at least one new strings column in global device memory for each API call, opening up the opportunity to combine multiple API calls into a custom kernel.
Performance limitations in kernel malloc calls
First, we’ll build a custom kernel to implement the redact example transformation. When designing this kernel, we must keep in mind that libcudf strings columns are immutable.
Strings columns cannot be changed in place because the character bytes are stored contiguously, and any changes to the length of a string would invalidate the offsets data. Therefore the redact_kernel
custom kernel generates a new strings column by using a libcudf column factory to build both offsets
and chars
child columns.
In this first approach, the output string for each row is created in dynamic device memory using a malloc call inside the kernel. The custom kernel output is a vector of device pointers to each row output, and this vector serves as input to a strings column factory.
The custom kernel accepts a cudf::column_device_view
to access the strings column data and uses the element
method to return a cudf::string_view
representing the string data at the specified row index. The kernel output is a vector of type cudf::string_view
that holds pointers to the device memory containing the output string and the size of that string in bytes.
The cudf::string_view
class is similar to the std::string_view class but is implemented specifically for libcudf and wraps a fixed length of character data in device memory encoded as UTF-8. It has many of the same features (find
and substr
functions, for example) and limitations (no null terminator) as the std
counterpart. A cudf::string_view
represents a character sequence stored in device memory and so we can use it here to record the malloc’d memory for an output vector.
Malloc kernel
// note the column_device_view inputs to the kernel
__global__ void redact_kernel(cudf::column_device_view const d_names,
cudf::column_device_view const d_visibilities,
cudf::string_view redaction,
cudf::string_view* d_output)
{
// get index for this thread
auto index = threadIdx.x + blockIdx.x * blockDim.x;
if (index >= d_names.size()) return;
auto const visible = cudf::string_view("public", 6);
auto const name = d_names.element(index);
auto const vis = d_visibilities.element(index);
if (vis == visible) {
auto const space_idx = name.find(' ');
auto const first = name.substr(0, space_idx);
auto const last_initial = name.substr(space_idx + 1, 1);
auto const output_size = first.size_bytes() + last_initial.size_bytes() + 1;
char* output_ptr = static_cast(malloc(output_size));
// build output string
d_output[index] = cudf::string_view{output_ptr, output_size};
memcpy(output_ptr, last_initial.data(), last_initial.size_bytes());
output_ptr += last_initial.size_bytes();
*output_ptr++ = ' ';
memcpy(output_ptr, first.data(), first.size_bytes());
} else {
d_output[index] = cudf::string_view{redaction.data(), redaction.size_bytes()};
}
}
__global__ void free_kernel(cudf::string_view redaction, cudf::string_view* d_output, int count)
{
auto index = threadIdx.x + blockIdx.x * blockDim.x;
if (index >= count) return;
auto ptr = const_cast(d_output[index].data());
if (ptr != redaction.data()) free(ptr); // free everything that does match the redaction string
}
This might seem like a reasonable approach, until the kernel performance is measured. This approach takes about 108 ms on an A6000 with 600K rows of data—more than 30x slower than the solution provided above using the libcudf strings API.
redact_kernel 60.3ms
free_kernel 45.5ms
make_strings_column 0.5ms
The main bottleneck is the malloc/free
calls inside the two kernels here. The CUDA dynamic device memory requires malloc/free
calls in a kernel to be synchronized, causing parallel execution to degenerate into sequential execution.
Pre-allocating working memory to eliminate bottlenecks
Eliminate the malloc/free
bottleneck by replacing the malloc/free
calls in the kernel with pre-allocated working memory before launching the kernel.
For the redact example, the output size of each string in this example should be no larger than the input string itself, since the logic only removes characters. Therefore, a single device memory buffer can be used with the same size as the input buffer. Use the input offsets to locate each row position.
Accessing the strings column’s offsets involves wrapping the cudf::column_view
with a cudf::strings_column_view
and calling its offsets_begin
method. The size of the chars
child column can also be accessed using the chars_size
method. Then a rmm::device_uvector
is pre-allocated before calling the kernel to store the character output data.
auto const scv = cudf::strings_column_view(names);
auto const offsets = scv.offsets_begin();
auto working_memory = rmm::device_uvector(scv.chars_size(), stream);
Pre-allocated kernel
__global__ void redact_kernel(cudf::column_device_view const d_names,
cudf::column_device_view const d_visibilities,
cudf::string_view redaction,
char* working_memory,
cudf::offset_type const* d_offsets,
cudf::string_view* d_output)
{
auto index = threadIdx.x + blockIdx.x * blockDim.x;
if (index >= d_names.size()) return;
auto const visible = cudf::string_view("public", 6);
auto const name = d_names.element(index);
auto const vis = d_visibilities.element(index);
if (vis == visible) {
auto const space_idx = name.find(' ');
auto const first = name.substr(0, space_idx);
auto const last_initial = name.substr(space_idx + 1, 1);
auto const output_size = first.size_bytes() + last_initial.size_bytes() + 1;
// resolve output string location
char* output_ptr = working_memory + d_offsets[index];
d_output[index] = cudf::string_view{output_ptr, output_size};
// build output string into output_ptr
memcpy(output_ptr, last_initial.data(), last_initial.size_bytes());
output_ptr += last_initial.size_bytes();
*output_ptr++ = ' ';
memcpy(output_ptr, first.data(), first.size_bytes());
} else {
d_output[index] = cudf::string_view{redaction.data(), redaction.size_bytes()};
}
}
The kernel outputs a vector of cudf::string_view
objects which is passed to the cudf::make_strings_column
factory function. The second parameter to this function is used for identifying null entries in the output column. The examples in this post do not have null entries, so a nullptr placeholder cudf::string_view{nullptr,0}
is used.
auto str_ptrs = rmm::device_uvector(names.size(), stream);
redact_kernel>>(*d_names,
*d_visibilities,
d_redaction.value(),
working_memory.data(),
offsets,
str_ptrs.data());
auto result = cudf::make_strings_column(str_ptrs, cudf::string_view{nullptr,0}, stream);
This approach takes about 1.1 ms on an A6000 with 600K rows of data and therefore beats the baseline by more than 2x. The approximate breakdown is shown below:
redact_kernel 66us
make_strings_column 400us
The remaining time is spent in cudaMalloc, cudaFree, cudaMemcpy,
which is typical of the overhead for managing temporary instances of rmm::device_uvector
. This method works well if all of the output strings are guaranteed to be the same size or smaller as the input strings.
Overall, switching to a bulk working memory allocation with RAPIDS RMM is a significant improvement and a good solution for a custom strings function.
Optimizing column creation for faster compute times
Is there a way to improve this even further? The bottleneck is now the cudf::make_strings_column
factory function which builds the two strings column components, offsets
and chars
, from the vector of cudf::string_view
objects.
In libcudf, many factory functions are included for building strings columns. The factory function used in the previous examples takes a cudf::device_span
of cudf::string_view
objects and then constructs the column by performing a gather
on the underlying character data to build the offsets and character child columns. A rmm::device_uvector
is automatically convertible to a cudf::device_span
without copying any data.
However, if the vector of characters and the vector of offsets are built directly, then a different factory function can be used, which simply creates the strings column without requiring a gather to copy the data.
The sizes_kernel
makes a first pass over the input data to compute the exact output size of each output row:
Optimized kernel: Part 1
__global__ void sizes_kernel(cudf::column_device_view const d_names,
cudf::column_device_view const d_visibilities,
cudf::size_type* d_sizes)
{
auto index = threadIdx.x + blockIdx.x * blockDim.x;
if (index >= d_names.size()) return;
auto const visible = cudf::string_view("public", 6);
auto const redaction = cudf::string_view("X X", 3);
auto const name = d_names.element(index);
auto const vis = d_visibilities.element(index);
cudf::size_type result = redaction.size_bytes(); // init to redaction size
if (vis == visible) {
auto const space_idx = name.find(' ');
auto const first = name.substr(0, space_idx);
auto const last_initial = name.substr(space_idx + 1, 1);
result = first.size_bytes() + last_initial.size_bytes() + 1;
}
d_sizes[index] = result;
}
The output sizes are then converted to offsets by performing an in-place exclusive_scan
. Note that the offsets
vector was created with names.size()+1
elements. The last entry will be the total number of bytes (all the sizes added together) while the first entry will be 0. These are both handled by the exclusive_scan
call. The size of the chars
column is retrieved from the last entry of the offsets
column to build the chars vector.
// create offsets vector
auto offsets = rmm::device_uvector(names.size() + 1, stream);
// compute output sizes
sizes_kernel>>(
*d_names, *d_visibilities, offsets.data());
thrust::exclusive_scan(rmm::exec_policy(stream), offsets.begin(), offsets.end(), offsets.begin());
The redact_kernel
logic is still very much the same except that it accepts the output d_offsets
vector to resolve each row’s output location:
Optimized kernel: Part 2
__global__ void redact_kernel(cudf::column_device_view const d_names,
cudf::column_device_view const d_visibilities,
cudf::size_type const* d_offsets,
char* d_chars)
{
auto index = threadIdx.x + blockIdx.x * blockDim.x;
if (index >= d_names.size()) return;
auto const visible = cudf::string_view("public", 6);
auto const redaction = cudf::string_view("X X", 3);
// resolve output_ptr using the offsets vector
char* output_ptr = d_chars + d_offsets[index];
auto const name = d_names.element(index);
auto const vis = d_visibilities.element(index);
if (vis == visible) {
auto const space_idx = name.find(' ');
auto const first = name.substr(0, space_idx);
auto const last_initial = name.substr(space_idx + 1, 1);
auto const output_size = first.size_bytes() + last_initial.size_bytes() + 1;
// build output string
memcpy(output_ptr, last_initial.data(), last_initial.size_bytes());
output_ptr += last_initial.size_bytes();
*output_ptr++ = ' ';
memcpy(output_ptr, first.data(), first.size_bytes());
} else {
memcpy(output_ptr, redaction.data(), redaction.size_bytes());
}
}
The size of the output d_chars
column is retrieved from the last entry of the d_offsets
column to allocate the chars vector. The kernel launches with the pre-computed offsets vector and returns the populated chars vector. Finally, the libcudf strings column factory creates the output strings columns.
This cudf::make_strings_column
factory function builds the strings column without making a copy of the data. The offsets
data and chars
data are already in the correct, expected format and this factory simply moves the data from each vector and creates the column structure around it. Once completed, the rmm::device_uvectors
for offsets
and chars
are empty, their data having been moved into the output column.
cudf::size_type output_size = offsets.back_element(stream);
auto chars = rmm::device_uvector(output_size, stream);
redact_kernel>>(
*d_names, *d_visibilities, offsets.data(), chars.data());
// from pre-assembled offsets and character buffers
auto result = cudf::make_strings_column(names.size(), std::move(offsets), std::move(chars));
This approach takes about 300 us (0.3 ms) on an A6000 with 600K rows of data and improves over the previous approach by more than 2x. You might notice that sizes_kernel
and redact_kernel
share much of the same logic: once to measure the size of the output and then again to populate the output.
From a code quality perspective, it is beneficial to refactor the transformation as a device function called by both the sizes and redact kernels. From a performance perspective, you might be surprised to see the computational cost of the transformation being paid twice.
The benefits for memory management and more efficient column creation often outweigh the computation cost of performing the transformation twice.
Table 2 shows the compute time, kernel count, and bytes processed for the four solutions discussed in this post. “Total kernel launches” reflects the total number of kernels launched, including both compute and helper kernels. “Total bytes processed” is the cumulative DRAM read plus write throughput and “minimum bytes processed” is an average of 37.9 bytes per row for our test inputs and outputs. The ideal “memory bandwidth limited” case assumes 768 GB/s bandwidth, the theoretical peak throughput of the A6000.
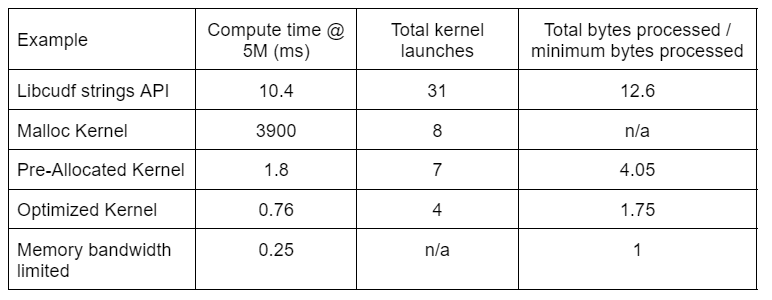
“Optimized Kernel” provides the highest throughput due to the reduced number of kernel launches and the fewer total bytes processed. With efficient custom kernels, the total kernel launches drop from 31 to 4 and the total bytes processed from 12.6x to 1.75x of the input plus output size.
As a result, the custom kernel achieves >10x higher throughput than the general purpose strings API for the redact transformation.
Peak performance analysis
The pool memory resource in RAPIDS Memory Manager (RMM) is another tool you can use to increase performance. The examples above use the default “CUDA memory resource” for allocating and freeing global device memory. However, the time needed to allocate working memory adds significant latency in between steps of the string transformations. The “pool memory resource” in RMM reduces latency by allocating a large pool of memory up front, and assigning suballocations as needed during processing.
With the CUDA memory resource, “Optimized Kernel” shows a 10x-15x speedup that begins to drop off at higher row counts due to the increasing allocation size (Figure 3). Using the pool memory resource mitigates this effect and maintains 15x-25x speedups over the libcudf strings API approach.
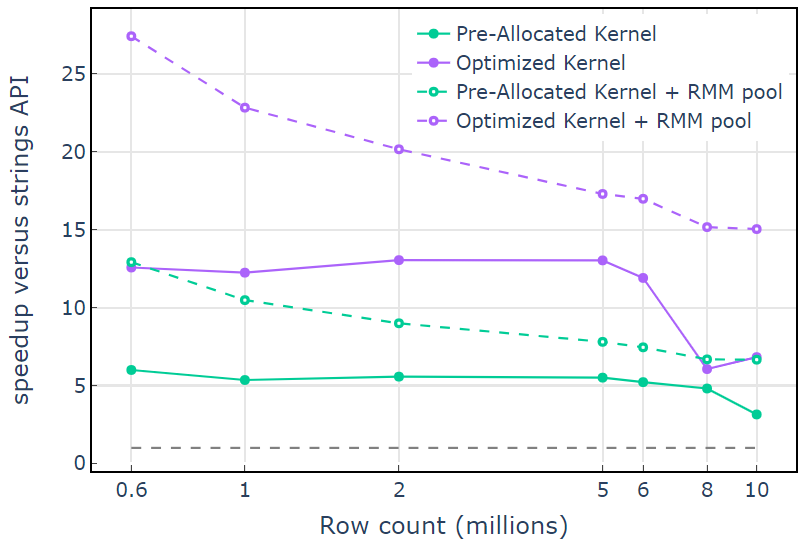
With the pool memory resource, an end-to-end memory throughput approaching the theoretical limit for a two-pass algorithm is demonstrated. “Optimized Kernel” reaches 320-340 GB/s throughput, measured using the size of inputs plus the size of outputs and the compute time (Figure 4).
The two-pass approach first measures the sizes of the output elements, allocates memory, and then sets the memory with the outputs. Given a two-pass processing algorithm, the implementation in “Optimized Kernel” performs close to the memory bandwidth limit. “End-to-end memory throughput” is defined as the input plus output size in GB divided by the compute time. *RTX A6000 memory bandwidth (768 GB/s).
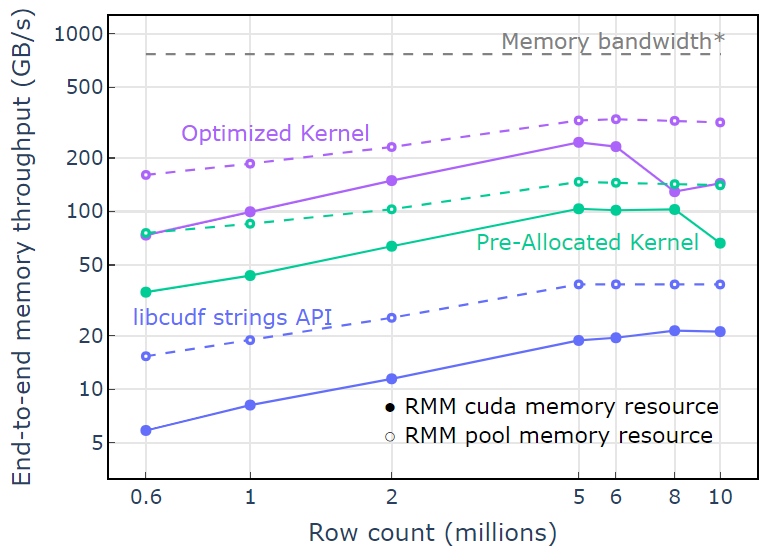
Key takeaways
This post demonstrates two approaches for writing efficient string data transformations in libcudf. The libcudf general purpose API is fast and straightforward for developers, and delivers good performance. libcudf also provides device-side utilities designed for use with custom kernels, in this example unlocking >10x faster performance.
Apply your knowledge
To get started with RAPIDS cuDF, visit the rapidsai/cudf GitHub repo. If you have not yet tried cuDF and libcudf for your string processing workloads, we encourage you to test the latest release. Docker containers are provided for releases as well as nightly builds. Conda packages are also available to make testing and deployment easier. If you’re already using cuDF, we encourage you to run the new strings transformation example by visiting rapidsai/cudf/tree/HEAD/cpp/examples/strings on GitHub.
Image captioning is the machine learning task of automatically generating a fluent natural language description for a given image. This task is important for improving accessibility for visually impaired users and is a core task in multimodal research encompassing both vision and language modeling.
However, datasets for image captioning are primarily available in English. Beyond that, there are only a few datasets covering a limited number of languages that represent just a small fraction of the world’s population. Further, these datasets feature images that severely under-represent the richness and diversity of cultures from across the globe. These aspects have hindered research on image captioning for a wide variety of languages, and directly hamper the deployment of accessibility solutions for a large potential audience around the world.
Today we present and make publicly available the Crossmodal 3600 (XM3600) image captioning evaluation dataset as a robust benchmark for multilingual image captioning that enables researchers to reliably compare research contributions in this emerging field. XM3600 provides 261,375 human-generated reference captions in 36 languages for a geographically diverse set of 3600 images. We show that the captions are of high quality and the style is consistent across languages.
The Crossmodal 3600 dataset includes reference captions in 36 languages for each of a geographically diverse set of 3600 images. All images used with permission under the CC-BY 2.0 license. |
Overview of the Crossmodal 3600 Dataset
Creating large training and evaluation datasets in multiple languages is a resource-intensive endeavor. Recent work has shown that it is feasible to build multilingual image captioning models trained on machine-translated data with English captions as the starting point. However, some of the most reliable automatic metrics for image captioning are much less effective when applied to evaluation sets with translated image captions, resulting in poorer agreement with human evaluations compared to the English case. As such, trustworthy model evaluation at present can only be based on extensive human evaluation. Unfortunately, such evaluations usually cannot be replicated across different research efforts, and therefore do not offer a fast and reliable mechanism to automatically evaluate multiple model parameters and configurations (e.g., model hill climbing) or to compare multiple lines of research.
XM3600 provides 261,375 human-generated reference captions in 36 languages for a geographically diverse set of 3600 images from the Open Images dataset. We measure the quality of generated captions by comparing them to the manually provided captions using the CIDEr metric, which ranges from 0 (unrelated to the reference captions) to 10 (perfectly matching the reference captions). When comparing pairs of models, we observed strong correlations between the differences in the CIDEr scores of the model outputs, and side-by-side human evaluations comparing the model outputs. , making XM3600 is a reliable tool for high-quality automatic comparisons between image captioning models on a wide variety of languages beyond English.
Language Selection
We chose 30 languages beyond English, roughly based on their percentage of web content. In addition, we chose an additional five languages that include under-resourced languages that have many native speakers or major native languages from continents that would not be covered otherwise. Finally, we also included English as a baseline, thus resulting in a total of 36 languages, as listed in the table below.
Arabic | Bengali* | Chinese | Croatian | Cusco Quechua* |
Czech | |||||
Danish | Dutch | English | Filipino | Finnish | French | |||||
German | Greek | Hebrew | Hindi | Hungarian | Indonesian | |||||
Italian | Japanese | Korean | Maori* | Norwegian | Persian | |||||
Polish | Portuguese | Romanian | Russian | Spanish | Swahili* | |||||
Swedish | Telugu* | Thai | Turkish | Ukrainian | Vietnamese |
List of languages used in XM3600. *Low-resource languages with many native speakers, or major native languages from continents that would not be covered otherwise. |
Image Selection
The images were selected from among those in the Open Images dataset that have location metadata. Since there are many regions where more than one language is spoken, and some areas are not well covered by these images, we designed an algorithm to maximize the correspondence between selected images and the regions where the targeted languages are spoken. The algorithm starts with the selection of images with geo-data corresponding to the languages for which we have the smallest pool (e.g., Persian) and processes them in increasing order of their candidate image pool size. If there aren’t enough images in an area where a language is spoken, then we gradually expand the geographic selection radius to: (i) a country where the language is spoken; (ii) a continent where the language is spoken; and, as last resort, (iii) from anywhere in the world. This strategy succeeded in providing our target number of 100 images from an appropriate region for most of the 36 languages, except for Persian (where 14 continent-level images are used) and Hindi (where all 100 images are at the global level, because the in-region images were assigned to Bengali and Telugu).
English![]() Photo by Chris Sampson |
Swahili![]() Photo by Henrik Palm |
Telugu![]() Photo by rojypala |
||
Cusco Quechua![]() Photo by McKay Savage |
Filipino![]() Photo by Simon Schoeters |
Chinese![]() Photo by Stefan Krasowski |
Sample images showcasing the geographical diversity of the annotated images. Images used under CC BY 2.0 license. |
Caption Generation
In total, all 3600 images (100 images per language) are annotated in all 36 languages, each with an average of two annotations per language, yielding a total of 261,375 captions.
Annotators work in batches of 15 images. The first screen shows all 15 images with their captions in English as generated by a captioning model trained to output a consistent style of the form “<main salient objects> doing <activities> in the <environment>”, often with object attributes, such as a “smiling” person, “red” car, etc. The annotators are asked to rate the caption quality given guidelines for a 4-point scale from “excellent” to “bad”, plus an option for “not_enough_information”. This step forces the annotators to carefully assess caption quality and it primes them to internalize the style of the captions. The following screens show the images again but individually and without the English captions, and the annotators are asked to produce descriptive captions in the target language for each image.
The image batch size of 15 was chosen so that the annotators would internalize the style without remembering the exact captions. Thus, we expect the raters to generate captions based on the image content only and lacking translation artifacts. For example in the example shown below, the Spanish caption mentions “number 42” and the Thai caption mentions “convertibles”, none of which are mentioned in the English captions. The annotators were also provided with a protocol to use when creating the captions, thus achieving style consistency across languages.
![]() Photo by Brian Solis |
English | • A vintage sports car in a showroom with many other vintage sports cars | ||
• The branded classic cars in a row at display | ||||
Spanish | • Automóvil clásico deportivo en exhibición de automóviles de galería — (Classic sports car in gallery car show) | |||
• Coche pequeño de carreras color plateado con el número 42 en una exhibición de coches — (Small silver racing car with the number 42 at a car show) | ||||
Thai | • รถเปิดประทุนหลายสีจอดเรียงกันในที่จัดแสดง — (Multicolored convertibles line up in the exhibit) | |||
• รถแข่งวินเทจจอดเรียงกันหลายคันในงานจัดแสดง — (Several vintage racing cars line up at the show.) |
Sample captions in three different languages (out of 36 — see full list of captions in Appendix A of the Crossmodal-3600 paper), showcasing the creation of annotations that are consistent in style across languages, while being free of direct-translation artifacts (e.g., the Spanish “number 42” or the Thai “convertibles” would not be possible when directly translating from the English versions). Image used under CC BY 2.0 license. |
<!–
>>>>> gd2md-html alert: inline image link here (to images/image7.jpg). Store image on your image server and adjust path/filename/extension if necessary. Photo by Brian Solis |
English |
|
Spanish |
|
|
Thai |
|
–>
Caption Quality and Statistics
We ran two to five pilot studies per language to troubleshoot the caption generation process and to ensure high quality captions. We then manually evaluated a random subset of captions. First we randomly selected a sample of 600 images. Then, to measure the quality of captions in a particular language, for each image, we selected for evaluation one of the manually generated captions. We found that:
- For 25 out of 36 languages, the percentage of captions rated as “Good” or “Excellent” is above 90%, and the rest are all above 70%.
- For 26 out of 36 languages, the percentage of captions rated as “Bad” is below 2%, and the rest are all below 5%.
For languages that use spaces to separate words, the number of words per caption can be as low as 5 or 6 for some agglutinative languages like Cusco Quechua and Czech, and as high as 18 for an analytic language like Vietnamese. The number of characters per caption also varies drastically — from mid-20s for Korean to mid-90s for Indonesian — depending on the alphabet and the script of the language.
Empirical Evaluation and Results
We empirically measured the ability of the XM3600 annotations to rank image captioning model variations by training four variations of a multilingual image captioning model and comparing the CIDEr differences of the models’ outputs over the XM3600 dataset for 30+ languages, to side-by-side human evaluations. We observed strong correlations between the CIDEr differences and the human evaluations. These results support the use of the XM3600 references as a means to achieve high-quality automatic comparisons between image captioning models on a wide variety of languages beyond English.
Recent Uses
Recently PaLI used XM3600 to evaluate model performance beyond English for image captioning, image-to-text retrieval and text-to-image retrieval. The key takeaways they found when evaluating on XM3600 were that multilingual captioning greatly benefits from scaling the PaLI models, especially for low-resource languages.
Acknowledgements
We would like to acknowledge the coauthors of this work: Xi Chen and Radu Soricut.
Building models that understand and generate natural language well is one the grand goals of machine learning (ML) research and has a direct impact on building smart systems for everyday applications. Improving the quality of language models is a key target for researchers to make progress toward such a goal.
Most common paradigms to build and train language models use either autoregressive decoder-only architectures (e.g., PaLM or GPT-3), where the model is trained to predict the next word for a given prefix phrase, or span corruption-based encoder-decoder architectures (e.g., T5, ST-MoE), where the training objective is to recover the subset of words masked out of the input. On the one hand, T5-like models perform well on supervised fine-tuning tasks, but struggle with few-shot in-context learning. On the other hand, autoregressive language models are great for open-ended generation (e.g., dialog generation with LaMDA) and prompt-based learning (e.g., in-context learning with PaLM), but may perform suboptimally on fine-tuning tasks. Thus, there remains an opportunity to create an effective unified framework for pre-training models.
In “Unifying Language Learning Paradigms”, we present a novel language pre-training paradigm called Unified Language Learner (UL2) that improves the performance of language models universally across datasets and setups. UL2 frames different objective functions for training language models as denoising tasks, where the model has to recover missing sub-sequences of a given input. During pre-training it uses a novel mixture-of-denoisers that samples from a varied set of such objectives, each with different configurations. We demonstrate that models trained using the UL2 framework perform well in a variety of language domains, including prompt-based few-shot learning and models fine-tuned for down-stream tasks. Additionally, we show that UL2 excels in generation, language understanding, retrieval, long-text understanding and question answering tasks. Finally, we are excited to publicly release the checkpoints for our best performing UL2 20 billion parameter model.
Background: Language Modeling Objectives and Architectures
Common objective functions for training language models can mostly be framed as learning data transformations that map inputs to targets. The model is conditioned on different forms of input to predict target tokens. To this end, different objectives utilize different properties of the inputs.
The standard Causal Language modeling objective (CausalLM) is trained to predict full sequence lengths and so, only recognizes tokens in the target output. The prefix language modeling objective (PrefixLM) modifies this process by randomly sampling a contiguous span of k tokens from the given tokenized text to form the input of the model, referred to as the “prefix”. The span corruption objective masks contiguous spans from the inputs and trains the model to predict these masked spans.
In the table below, we list the common objectives on which state-of-the-art language models are trained along with different characteristics of the input, i.e., how it is presented to the model. Moreover, we characterize the example efficiency of each objective in terms of the ability of the model for exploiting supervision signals from a single input, e.g., how much of the input tokens contribute to the calculation of the loss.
Objective Function |
Inputs (Bi-directional) |
Targets (Causal) |
Input Properties |
Example Efficiency |
CausalLM | none | text | N/A | full seq_len |
PrefixLM | text (up to position k) | text (after position k) | contiguous | seq_len – k |
Span corruption | masked text | masked_tokens | non-contiguous, may be bi-directional | typically lower than others |
Common objectives used in today’s language models. Throughout, “text” indicates tokenized text. |
UL2 leverages the strengths of each of these objective functions through a framework that generalizes over each of them, which enables the ability to reason and unify common pre-training objectives. Based on this framework, the main task for training a language model is to learn the transformation of a sequence of input tokens to a sequence of target tokens. Then all the objective functions introduced above can be simply reduced to different ways of generating input and target tokens. For instance, the PrefixLM objective can be viewed as a transformation that moves a segment of k contiguous tokens from the inputs to the targets. Meanwhile, the span corruption objective is a data transformation that corrupts spans (a subsequence of tokens in the input), replacing them with mask tokens that are shifted to the targets.
It is worth noting that one can decouple the model architecture and the objective function with which it’s trained. Thus, it is possible to train different architectures, such as the common single stack decoder-only and two-stack encoder-decoder models, with any of these objectives.
Mixture of Denoisers
The UL2 framework can be used to train a model on a mixture of pre-training objectives and supply it with capabilities and inductive bias benefits from different pre-training tasks. Training on the mixture helps the model leverage the strengths of different tasks and mitigates the weaknesses of others. For instance, the mixture-of-denoisers objective can strongly improve the prompt-based learning capability of the model as opposed to a span corruption-only T5 model.
UL2 is trained using a mixture of three denoising tasks: (1) R-denoising (or regular span corruption), which emulates the standard T5 span corruption objective; (2) X-denoising (or extreme span corruption); and (3) S-denoising (or sequential PrefixLM). During pre-training, we sample from the available denoising tasks based on user-specified ratios (i.e., different combinations of the R, X, and S-denoisers) and prepare the input and target appropriately. Then, a paradigm token is appended to the input (one of [R]
, [X]
, or [S]
) indicating the denoising task at hand.
![]() |
An overview of the denoising objectives used in UL2’s mixture-of-denoisers. |
Improving Trade-Offs Across Learning Paradigms
Many existing commonly used language learning paradigms typically excel at one type of task or application, such as fine-tuning performance or prompt-based in-context learning. In the plot below, we show baseline objective functions on different tasks compared to UL2: CausalLM (referred to as GPT-like), PrefixLM, Span Corrupt (also referred to as T5 in the plot), and a baseline objective function proposed by UniLM. We use these objectives for training decoder only architectures (green) and encoder-decoder architectures (blue) and evaluate different combinations of objective functions and architectures on two main sets of tasks:
- Fine-tuning, by measuring performance on SuperGLUE (y-axis of the plot below)
- In-context learning, by measuring performance of the model on a suite of 1-shot GEM tasks (e.g., XSUM, SGD or Schema guided dialog and TOTTO) (x-axis of the plot below).
For most of the existing language learning paradigms, there is a trade-off between the quality of the model on these two sets of tasks. We show that UL2 bridges this trade-off across in-context learning and fine-tuning.
UL2 for Few-Shot Prompting and Chain-of-Thought Reasoning
We scale up UL2 and train a 20 billion parameter encoder-decoder model on the public C4 corpus and demonstrate some impressive capabilities of the UL2 20B model.
UL2 is a powerful in-context learner that excels at both few-shot and chain-of-thought (CoT) prompting. In the table below, we compare UL2 with other state-of-the-art models (e.g, T5 XXL and PaLM) for few-shot prompting on the XSUM summarization dataset. Our results show that UL2 20B outperforms PaLM and T5, both of which are in the same ballpark of compute cost.
Model | ROUGE-1 | ROUGE-2 | ROUGE-L |
LaMDA 137B | – | 5.4 | – |
PaLM 62B | – | 11.2 | – |
PaLM 540B | – | 12.2 | – |
PaLM 8B | – | 4.5 | – |
T5 XXL 11B | 0.6 | 0.1 | 0.6 |
T5 XXL 11B + LM | 13.3 | 2.3 | 10.7 |
UL2 20B | 25.5 | 8.6 | 19.8 |
Comparison of UL2 with T5 XXL, PaLM and LamDA 137B on 1-shot summarization (XSUM) in terms of ROUGE-1/2/L (higher is better), which captures the quality by comparing the generated summaries with the gold summaries as reference. |
Most CoT prompting results have been obtained using much larger language models, such as GPT-3 175B, PaLM 540B, or LaMDA 137B. We show that reasoning via CoT prompting can be achieved with UL2 20B, which is both publicly available and several times smaller than prior models that leverage chain-of-thought prompting. This enables an open avenue for researchers to conduct research on CoT prompting and reasoning at an accessible scale. In the table below, we show that for UL2, CoT prompting outperforms standard prompting on math word problems with a range of difficulties (GSM8K, SVAMP, ASDiv, AQuA, and MAWPS). We also show that self-consistency further improves performance.
![]() |
Chain-of-thought (CoT) prompting and self-consistency (SC) results on five arithmetic reasoning benchmarks. |
Conclusion and Future Directions
UL2 demonstrates superior performance on a plethora of fine-tuning and few-shot tasks. We publicly release checkpoints of our best performing UL2 model with 20 billion parameters, which we hope will inspire faster progress in developing better language models in the machine learning community as a whole.
Acknowledgements
It was an honor and privilege to work on this with Vinh Q. Tran, Xavier Garcia, Jason Wei, Xuezhi Wang, Hyung Won Chung, Dara Bahri, Tal Schuster, Huaixiu Steven Zheng, Denny Zhou, Neil Houlsby and Donald Metzler. We further acknowledge Alexey Gritsenko, Andrew M. Dai, Jacob Devlin, Jai Gupta, William Fedus, Orhan Firat, Sebastian Gerhmann, Nan Du, Dave Uthus, Siamak Shakeri, Slav Petrov and Quoc Le for support and discussions. We thank the Jax and T5X team for building such wonderful infrastructure that made this research possible.
As a civil engineer, Scott Ashford used explosives to make the ground under Japan’s Sendai airport safer in an earthquake. Now, as the dean of the engineering college at Oregon State University, he’s at ground zero of another seismic event. In its biggest fundraising celebration in nearly a decade, Oregon State announced plans today for Read article >
The post AI Supercomputer to Power $200 Million Oregon State University Innovation Complex appeared first on NVIDIA Blog.
Career-related questions are common during NVIDIA cybersecurity webinars and GTC sessions. How do you break into the profession? What experience do you need?…
Career-related questions are common during NVIDIA cybersecurity webinars and GTC sessions. How do you break into the profession? What experience do you need? And how do AI skills intersect with cybersecurity skills?
The truth is, that while the barrier to entry may seem high, there is no single path into a career that focuses on or incorporates cybersecurity and AI. With many disciplines in the field, there is a massive need to grow the cybersecurity workforce. According to the (ISC)² 2021 Cyber Workforce Report, the global cybersecurity workforce needs to grow 65% to effectively defend organizations’ critical assets.
Whether you’re just starting your career or are looking to make a mid-career change, read on for five tips to help you break into the field.
1. Evaluate your raw skills
Organizations of all sizes are facing a cybersecurity skills gap. Fortunately, the skills needed to work in cybersecurity are not strictly defined. Everyone has a different skill set and can bring something unique to the table. Evaluate your skills with the following questions:
- Are you curious? Cybersecurity professionals investigate, ask questions, and figure out how to optimize processes and tools.
- Are you good at explaining concepts? Communicating with both technical and non-technical audiences, including customers, is a highly valued skill.
- Do you like building (or breaking) things? Cybersecurity practitioners are constantly optimizing and building.
- Do you like analyzing data? Identifying patterns and behaviors is often required when you work in cybersecurity.
- Are you calm under pressure? Responding to reports of security vulnerabilities and active security incidents helps protect customers.
Taking a close look at your raw skills and strengths helps narrow down the roles that might be right for you.
2. Determine which areas and roles interest you
Cybersecurity touches every aspect of business, which means you can contribute in a variety of specific areas and roles, including:
- IT Operations – Are you technical? Are you a builder? Do you like to analyze data? Cybersecurity practitioners and teams are typically part of IT Operations.
- Marketing and Communications – Communicating in a way that a variety of audiences can understand is essential in cybersecurity. While they may not be traditional practitioners, marketers and PR managers are critical in the cybersecurity industry.
- Training – Building training programs around cyber hygiene and company policies may be right for you if you enjoy teaching.
- Risk Management and Compliance – Evaluating cyber risk is on every leader’s mind. Risk managers who specialize in cyber risk and compliance are valuable.
- Architect or Engineer – Designing and building secure products and services are imperative to protecting any organization’s assets.
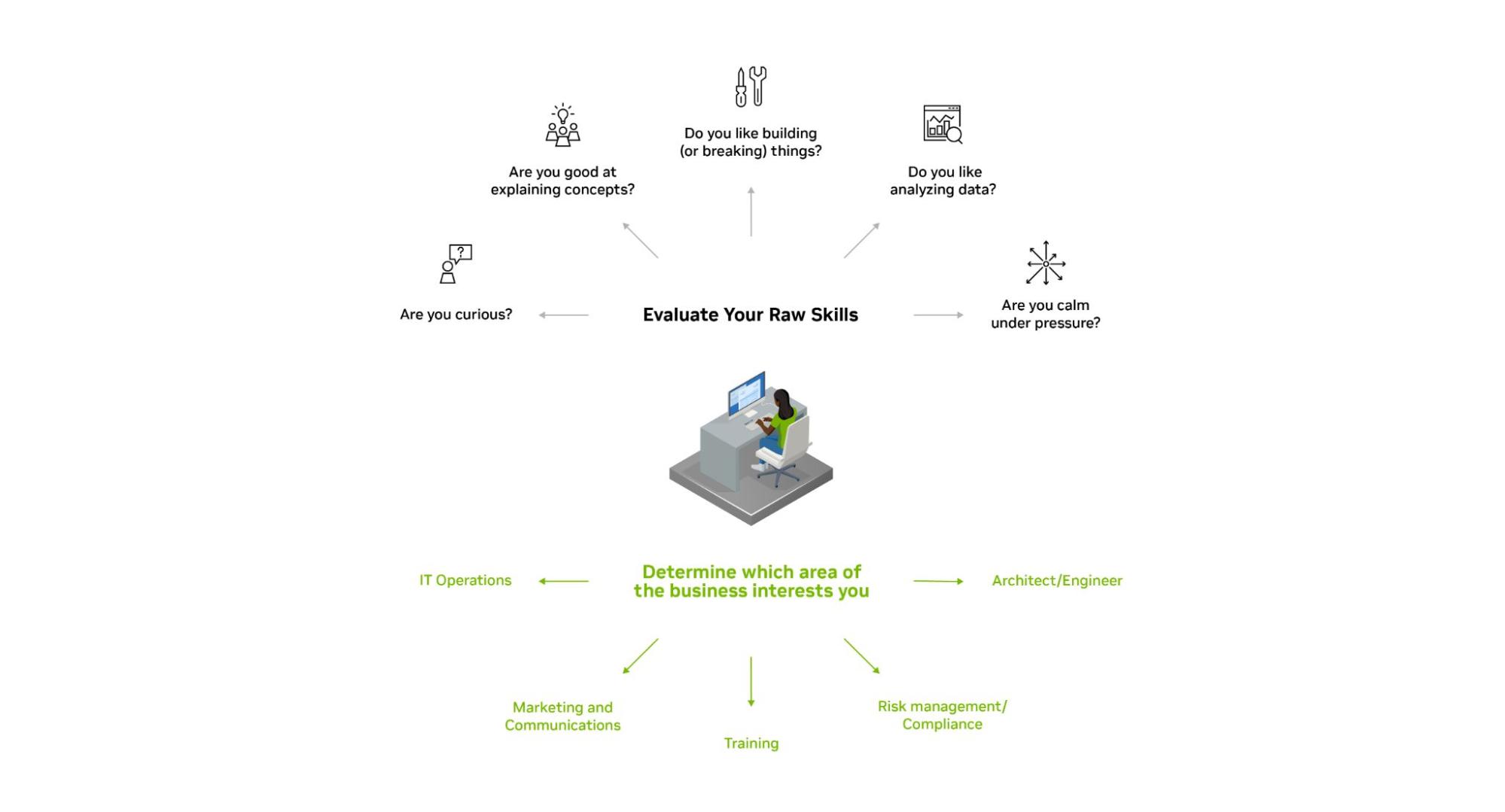
3. Build connections with subject matter experts
Connecting with experts in the areas you don’t know well can be a tremendous help as you begin your career. This is particularly true if you are interested in a career that incorporates cybersecurity and AI.
If you have a background in data science or AI, and cybersecurity is now piquing your interest, reach out to cybersecurity professionals within your organization. Shadow them, learn from them, and exchange information. Become each other’s counterparts and figure out how combining your skills can have a positive impact on the security of your organization.
4. Identify cybersecurity issues that AI can solve
Cybersecurity and AI are becoming increasingly intertwined. Individuals who understand both are in demand in the current workforce. More specifically, the industry needs those who understand cybersecurity and AI deeply enough to identify when, where, and how to apply AI techniques to cybersecurity workflows.
If a career that combines cybersecurity and AI interests you, start defining use cases for applying AI to cybersecurity. What challenges do you think AI can solve? Simply identifying these use cases is beneficial to security teams and can help you hit the ground running.
5. Invest in your professional development
Learn from experts and invest in your professional development. Take time to research courses and events. NVIDIA offers a range of cybersecurity sessions twice a year at our GTC conferences. Many of these sessions are available year-round through NVIDIA On-Demand. You can also take courses year-round through the NVIDIA Deep Learning Institute. Once you’re hired, on-the-job training is common in the field of cybersecurity.
Check out other industry conferences like RSA, Blackhat, DefCon (AI Village), regional BSides, and InfoSec. The Camlis Conference is an annual event that gathers researchers and practitioners to discuss machine learning in cybersecurity. USENIX Security Conference brings together researchers, practitioners, system administrators, system programmers, and others interested in the latest advances in the security and privacy of computer systems and networks.
If you’re interested in a career in cybersecurity, evaluating your skills is a good place to start. Then research opportunities in the field and be proactive with your professional relationships and development.
Learn to detect data abnormalities before they impact your business by using XGBoost, autoencoders, and GANs.
Learn to detect data abnormalities before they impact your business by using XGBoost, autoencoders, and GANs.