NVIDIA today announced the second generation of NVIDIA OVX™, powered by the NVIDIA Ada Lovelace GPU architecture and enhanced networking technology, to deliver groundbreaking real-time graphics, AI and digital twin simulation capabilities.
NVIDIA and Deloitte today announced an expansion of their alliance to help enable enterprises around the world to develop, implement and deploy hybrid-cloud solutions using the NVIDIA AI and NVIDIA Omniverse™ Enterprise platforms.
The latest release of NVIDIA Maxine is paving the way for real-time audio and video communications. Whether for a video conference, a call made to a customer service center, or a live stream, Maxine enables clear communications to enhance virtual interactions. NVIDIA Maxine is a suite of GPU-accelerated AI software development kits (SDKs) and cloud-native Read article >
The post New NVIDIA Maxine Cloud-Native Architecture Delivers Breakthrough Audio and Video Quality at Scale appeared first on NVIDIA Blog.
Meet Violet, an AI-powered customer service assistant ready to take your order. Unveiled this week at GTC, Violet is a cloud-based avatar that represents the latest evolution in avatar development through NVIDIA Omniverse Avatar Cloud Engine (ACE), a suite of cloud-native AI microservices that make it easier to build and deploy intelligent virtual assistants and Read article >
The post NVIDIA Omniverse ACE Enables Easier, Faster Deployment of Interactive Avatars appeared first on NVIDIA Blog.
New cloud services to support AI workflows and the launch of a new generation of GeForce RTX GPUs featured today in NVIDIA CEO Jensen Huang’s GTC keynote, which was packed with new systems, silicon, and software. “Computing is advancing at incredible speeds, the engine propelling this rocket is accelerated computing, and its fuel is AI,” Read article >
The post Keynote Wrap-Up: NVIDIA CEO Unveils Next-Gen RTX GPUs, AI Workflows in the Cloud appeared first on NVIDIA Blog.
Today, NVIDIA announced the Jetson Orin Nano series of system-on-modules (SoMs). They deliver up to 80X the AI performance of NVIDIA Jetson Nano and set the new…
Today, NVIDIA announced the Jetson Orin Nano series of system-on-modules (SoMs). They deliver up to 80X the AI performance of NVIDIA Jetson Nano and set the new standard for entry-level edge AI and robotics applications.
For the first time, the Jetson family now includes NVIDIA Orin-based modules that span from the entry-level Jetson Orin Nano to the highest-performance Jetson AGX Orin. This gives customers the flexibility to scale their applications easily.
Jump-start your Jetson Orin Nano development today with full software emulation support provided by the Jetson AGX Orin Developer Kit.
The need for increased real-time processing capability continues to grow for everyday use cases across industries. Entry-level AI applications like smart cameras, handheld devices, service robots, intelligent drones, smart meters, and more all face similar challenges.
These applications require more low-latency processing on-device for the data flowing from their multimodal sensor pipelines while keeping within the constraints of a power-efficient, cost-optimized small form factor.
Jetson Orin Nano series
Jetson Orin Nano series production modules will be available in January starting at $199. The modules deliver up to 40 TOPS of AI performance in the smallest Jetson form-factor, with power options as little as 5W and up to 15W. The series comes with two different versions: Jetson Orin Nano 4GB and Jetson Orin Nano 8GB.
*NVIDIA Orin Architecture from Jetson Orin Nano 8GB, Jetson Orin Nano 4GB has 2 TPCs and 4 SMs.
As shown in Figure 1, Jetson Orin Nano showcases the NVIDIA Orin architecture with an NVIDIA Ampere Architecture GPU. It has up to eight streaming multiprocessors (SMs) composed of 1024 CUDA cores and up to 32 Tensor Cores for AI processing.
The NVIDIA Ampere Architecture third-generation Tensor Cores deliver better performance per watt than the previous generation and bring more performance with support for sparsity. With sparsity, you can take advantage of the fine-grained structured sparsity in deep learning networks to double the throughput for Tensor Core operations.
To accelerate all parts of your application pipeline, Jetson Orin Nano also includes a 6-core Arm Cortex-A78AE CPU, video decode engine, ISP, video image compositor, audio processing engine, and video input block.
Within its small, 70x45mm 260-pin SODIMM footprint, the Jetson Orin Nano modules include various high-speed interfaces:
- Up to seven lanes of PCIe Gen3
- Three high-speed 10-Gbps USB 3.2 Gen2 ports
- Eight lanes of MIPI CSI-2 camera ports
- Various sensor I/O
To reduce your engineering effort, we’ve made the Jetson Orin Nano and Jetson Orin NX modules completely pin– and form-factor–compatible. Table 1 shows the differences between the Jetson Orin Nano 4GB and the Jetson Orin Nano 8GB.
Jetson Orin Nano 4GB | Jetson Orin Nano 8GB | |
AI Performance | 20 Sparse TOPs | 10 Dense TOPs | 40 Sparse TOPs | 20 Dense TOPs |
GPU | 512-core NVIDIA Ampere Architecture GPU with 16 Tensor Cores | 1024-core NVIDIA Ampere Architecture GPU with 32 Tensor Cores |
GPU Max Frequency | 625 MHz | |
CPU | 6-core Arm Cortex-A78AE v8.2 64-bit CPU 1.5 MB L2 + 4 MB L3 | |
CPU Max Frequency | 1.5 GHz | |
Memory | 4GB 64-bit LPDDR5 34 GB/s | 8GB 128-bit LPDDR5 68 GB/s |
Storage | – (Supports external NVMe) |
|
Video Encode | 1080p30 supported by 1-2 CPU cores | |
Video Decode | 1x 4K60 (H.265) | 2x 4K30 (H.265) | 5x 1080p60 (H.265) | 11x 1080p30 (H.265) | |
Camera | Up to 4 cameras (8 through virtual channels*) 8 lanes MIPI CSI-2 D-PHY 2.1 (up to 20 Gbps) | |
PCIe | 1 x4 + 3 x1 (PCIe Gen3, Root Port, & Endpoint) | |
USB | 3x USB 3.2 Gen2 (10 Gbps) 3x USB 2.0 | |
Networking | 1x GbE | |
Display | 1x 4K30 multimode DisplayPort 1.2 (+MST)/e DisplayPort 1.4/HDMI 1.4* | |
Other I/O | 3x UART, 2x SPI, 2x I2S, 4x I2C, 1x CAN, DMIC and DSPK, PWM, GPIOs | |
Power | 5W – 10W | 7W – 15W |
Mechanical | 69.6 mm x 45 mm 260-pin SO-DIMM connector | |
Price | $199† | $299† |
* For more information about additional compatibility to DisplayPort 1.4a and HDMI 2.1 and virtual channels, see the Jetson Orin Nano series data sheet.
† 1KU Volume
For more information about supported features, see the Software Features section of the latest NVIDIA Jetson Linux Developer Guide.
Start your development today using the Jetson AGX Orin Developer Kit and emulation
The Jetson AGX Orin Developer Kit and all the Jetson Orin modules share one SoC architecture, enabling the developer kit to emulate any of the modules and make it easy for you to start developing your next product today.
You don’t have to wait for the Jetson Orin Nano hardware to be available before starting to port their applications to the new NVIDIA Orin architecture and latest NVIDIA JetPack. With the new overlay released today, you can emulate the Jetson Orin Nano modules with the developer kit, just as with the other Jetson Orin modules. With the developer kit configured to emulate Jetson Orin Nano 8GB or Jetson Orin Nano 4GB, you can develop and run your full application pipeline.
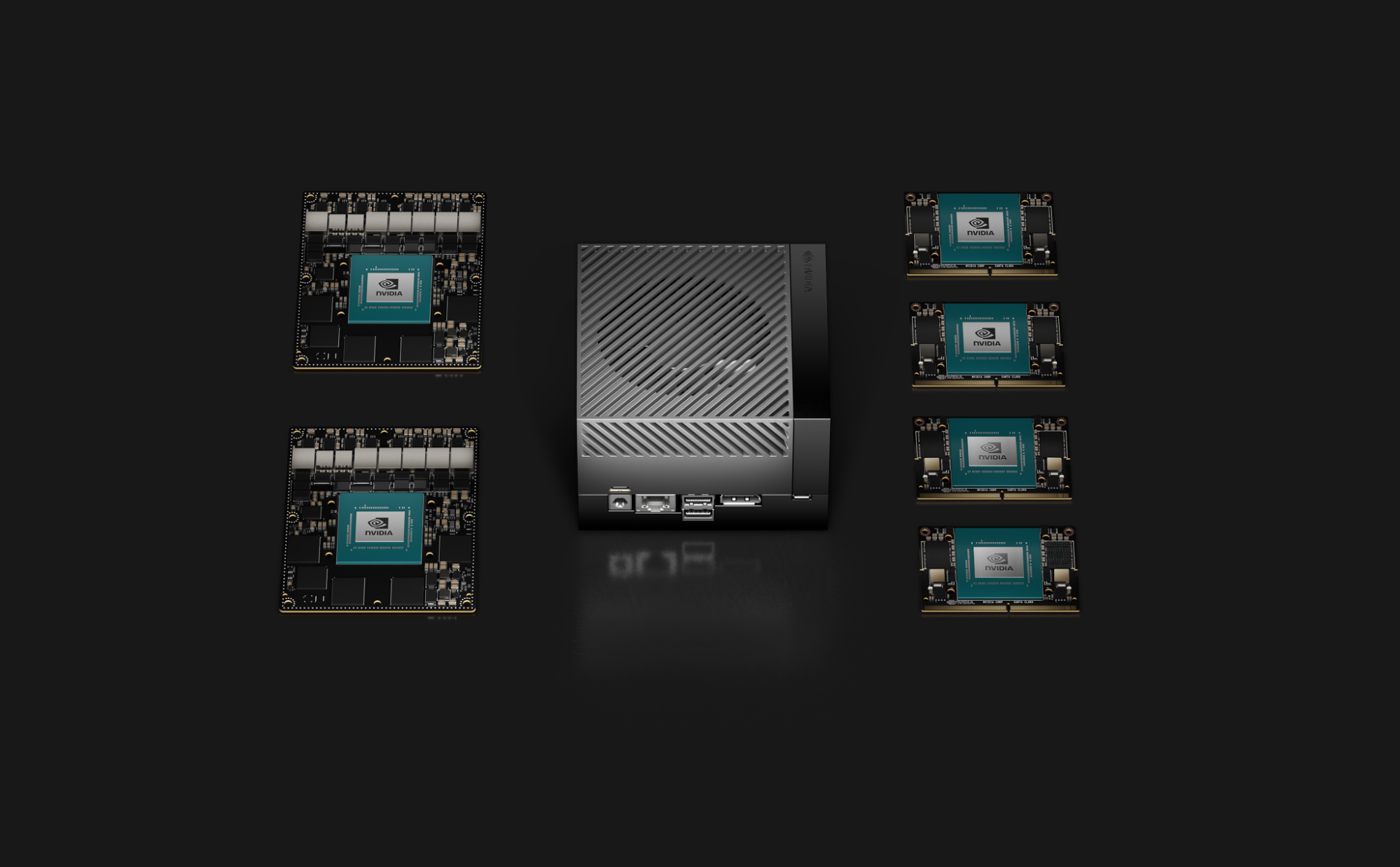
Performance benchmarks with Jetson Orin Nano
With Jetson AGX Orin, NVIDIA is leading the inference performance category of MLPerf. Jetson Orin modules provide a giant leap forward for your next-generation applications, and now the same NVIDIA Orin architecture is made accessible for entry-level AI devices.
We used emulation mode with NVIDIA JetPack 5.0.2 to run computer vision benchmarks with Jetson Orin Nano, and the results showcase how it sets the new standard. Testing included some of our dense INT8 and FP16 pretrained models from NGC, and a standard ResNet-50 model. We also ran the same models for comparison on Jetson Nano, TX2 NX, and Xavier NX.
Here is the full list of benchmarks:
- NVIDIA PeopleNet v2.3 for pruned people detection, and NVIDIA PeopleNet v2.5 for the highest accuracy people detection
- NVIDIA ActionRecognitionNet 2D and 3D models
- NVIDIA LPRNet for license plate recognition
- NVIDIA DashCamNet, BodyPoseNet for multiperson human pose estimation
- ResNet-50 (224×224) Model
Taking the geomean of these benchmarks, Jetson Orin Nano 8GB shows a 30x performance increase compared to Jetson Nano. With future software improvements, we expect this to approach a 45x performance increase. Other Jetson devices have increased performance 1.5x since their first supporting software release, and we expect the same with Jetson Orin Nano.
Jetson runs the NVIDIA AI software stack, and use-case-specific application frameworks are available, including NVIDIA Isaac for robotics, NVIDIA DeepStream for vision AI, and NVIDIA Riva for conversational AI. You can save significant time with the NVIDIA Omniverse Replicator for synthetic data generation (SDG), and with the NVIDIA TAO Toolkit for fine-tuning pretrained AI models from the NGC catalog.
Jetson compatibility with the overall NVIDIA AI accelerated computing platform makes for ease of development and seamless migration. For more information about the NVIDIA software technologies that we bring in Jetson Orin, join us for an upcoming webinar about NVIDIA JetPack 5.0.2.
Strengthen entry-level robots with NVIDIA Isaac ROS
The Jetson Orin platform is designed to solve the toughest robotics challenges and brings accelerated computing to over 700,000 ROS developers. Combined with the powerful hardware capabilities of Jetson Orin Nano, enhancements in the latest NVIDIA Isaac software for ROS deliver excellent performance and productivity in the hands of roboticists.
The new Isaac ROS DP release optimizes ROS2 node-processing pipelines that can be executed on the Jetson Orin platform and provides new DNN-based GEMS designed to increase throughput. The Jetson Orin Nano can take advantage of those highly optimized ROS2 packages for tasks such as localization, real-time 3D reconstruction, and depth estimation, which can be used for obstacle avoidance.
Unlike the original Jetson Nano, which can only process simple applications, the Jetson Orin Nano can run more complex applications. With a continuing commitment to improving NVIDIA Isaac ROS, you’ll see increased accuracy and throughput on the Jetson Orin Platform over time.
For roboticists developing the next generation of service robots, intelligent drones, and more, the Jetson Orin Nano is the ideal solution with up to 40 TOPS for modern AI inference pipelines in a power-efficient and small form factor.
Get started developing for all six Jetson Orin modules by placing an order for the Jetson AGX Orin Developer Kit and installing the latest NVIDIA JetPack.
For more information about the overlay for emulating Jetson Orin Nano modules, see Jetson Linux and read the NVIDIA Jetson Orin Nano documentation available at the Jetson download center. For more information and support, see the NVIDIA Embedded Developer page and the Jetson forums.
Supercomputers are used to model and simulate the most complex processes in scientific computing, often for insight into new discoveries that otherwise would be…
Supercomputers are used to model and simulate the most complex processes in scientific computing, often for insight into new discoveries that otherwise would be impractical or impossible to demonstrate physically.
The NVIDIA BlueField data processing unit (DPU) is transforming high-performance computing (HPC) resources into more efficient systems, while accelerating problem solving across a breadth of scientific research, from mathematical modeling and molecular dynamics to weather forecasting, climate research, and even renewable energy.
BlueField has already made a marked impact in the areas of cloud networking, security, telecommunications, and edge computing. In addition, there are several areas across high-performance computing where it is sparking innovations for application performance and system efficiency.
NVIDIA BlueField-3 provides powerful computing based on multiple Arm AArch64 cores, a multithreaded datapath accelerator, integrated NVIDIA ConnectX-7 400Gb/s networking, and a broad range of programmable acceleration engines in the I/O path. It’s equipped with dual DDR 6500MT/s DRAM controllers and comes standard with 64 GB onboard memory. BlueField-3 is the third-generation data center infrastructure-on-a-chip that enables incredibly efficient and powerful software-defined, hardware-accelerated infrastructures from cloud to core data center to edge.
So, what does all this mean for high-performance computing?
Boosting HPC application performance and scalability
HPC is all about increasing performance and scalability. For nearly two decades, InfiniBand networking has been the proven leader in terms of performance and application scalability for several reasons.
From a high-level view, InfiniBand is just the most efficient way to move data: direct data placement. There’s no need for the CPU or operating system to be involved and no need for making multiple copies of the data as it makes its way from the network interface, through the system to the actual application that needs it.
If InfiniBand is already so efficient, what benefit would BlueField provide?
One of the key challenges that InfiniBand has been addressing for years is moving network communication overhead away from the CPU, enabling it to spend its time focusing on what it does best: application computation and branching code.
The CPU in today’s mainstream servers is overly general-purpose, sharing its compute cycles, time, and resources across hundreds or thousands of processes that have little to nothing to do with actual computing.
BlueField is bringing unprecedented innovation and efficiency to supercomputing by offloading, accelerating, and isolating a broad range of advanced networking, storage, and security services.
Why the era of AI ushered in the need for the BlueField DPU
The field of artificial intelligence research was founded as an academic discipline in 1956. Even a decade before that, scientists began to discuss the possibility of creating an artificial brain. It was much later that the concepts became reality, with more modern computer hardware and software.
In 2006, NVIDIA introduced CUDA, the industry’s first C-compiler developer environment for the GPU, solving complex computing problems up to 100x faster than traditional approaches. Today, artificial intelligence is prolific and driving nearly every area of scientific research, changing our lives and shaping the industrial landscape.
Similarly, references to the first proposals for nonblocking collective operations were introduced mid-2006. The proposed nonblocking interfaces for the collective group communication functions of Message Passing Interface (MPI) was certainly prolific in theory. However, it was not implemented across many applications. Perhaps this was because, until the introduction of the DPU, the full benefits could not be realized.
Today, with BlueField-3, the technology has arrived—providing the fundamental elements needed for innovation, performance, and efficiency. There is a renewed interest in nonblocking collective operations for increased application performance and scalability, and counter the effects of operating system jitter.
There are also several areas across scientific computing, including early examples, where BlueField is demonstrating how it can be used to transform HPC into highly efficient and sustainable computing.
Saving CPU cycles with in-network computing
NVIDIA Scalable Hierarchical Aggregation and Reduction Protocol (SHARP) technology improves upon the performance of MPI operation, by offloading many blocking collective operations from the CPU to the switch network, and eliminating the need to send data multiple times between endpoints. This innovative approach decreases the amount of data traversing the network as aggregation nodes are reached, and dramatically reduces the MPI operations time.
BlueField extends additional in-network computing capabilities by leveraging its Arm cores to implement the nonblocking operations. This enables the system host CPU to perform computation with peak overlap.
Figure 2 shows an example of this using the MVAPICH2-DPU library, which is being optimized to take advantage of the full potential of BlueField. It shows the capability to extract peak overlap between computation happening at the host and MPI_Ialltoall communication.
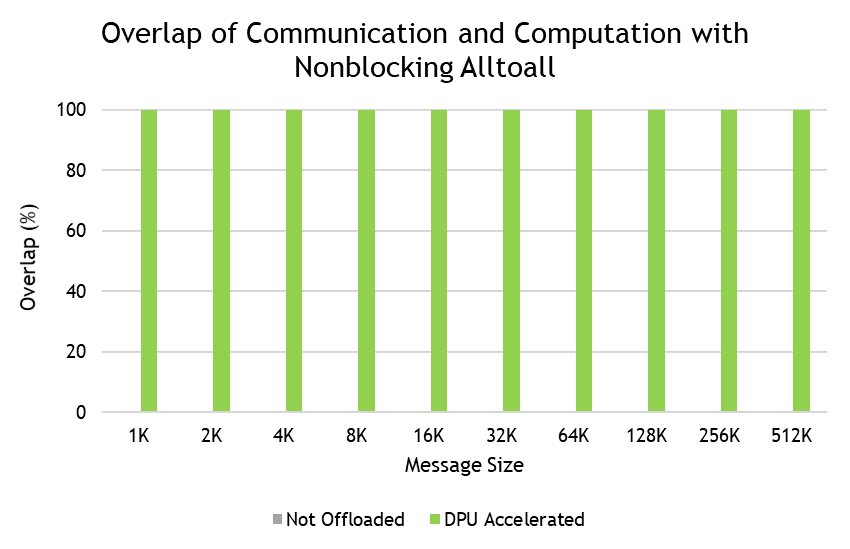
Computational storage for HPC workloads
Computational storage, or in-storage computing, brings HPC capabilities to traditional storage devices. In-storage computing enables you to perform selected computing tasks within or next to a storage device, offloading host processing and reducing data movement. BlueField provides the ability to combine in-storage and in-networking computing on a single card.
BlueField enables storage software stacks to be offloaded from compute nodes while also existing as a fabric-attached NVMe controller capable of accelerating critical storage functions, such as compression, checksum calculation, and parity generation. Such services are offered in parallel file systems.
The entire storage system stack is transparently offloaded within the Linux kernel while enabling simple NVIDIA DOCA implementations of standard storage functions on the NVMe target side.
The next-generation open storage architecture offers a new paradigm for accelerating, isolating, and securing high-performance storage systems. The system employs hardware and software co-design, making the DPU incredibly efficient and transparent to the user.
Acceleration of the file system means increasing the performance of critical functions within the storage system, with storage system performance being a key enabler of deep-learning-based scientific inquiry.
The ability to fully offload both the storage client and server onto DPUs leads to previously unrealizable levels of security and performance isolation. Critical data plane and control plane functions are moved to a separate domain on the DPU. This relieves the server CPU from the work and protects the functions in case the CPU or its software are compromised.
NVIDIA DOCA software framework
The NVIDIA DOCA SDK is the key to unlocking the potential of BlueField. Together, NVIDIA DOCA and BlueField enable the development of applications that deliver breakthrough networking, security, storage, and application performance with a comprehensive, open development platform.
NVIDIA DOCA supports a range of operating systems and distributions and includes drivers, libraries, tools, documentation, and example applications. The upcoming NVIDIA DOCA 1.5 and 2.0 releases introduce a broad range of networking, storage, security capabilities, and enhancements that deliver breakthrough performance and advanced programmability for HPC developers:
- A new communication channel library
- Fast access to host memory for UCX accelerations
- Storage emulation (SNAP) including storage encryption
- New NVIDIA DOCA services including UCC offload service and telemetry service
- NVIDIA DOCA security SDK
Transforming HPC today and tomorrow
There are many areas of innovation already on the horizon where BlueField, NVIDIA DOCA, and the community will continue to transform HPC.
Some ideas are already past the whiteboard, such as enhanced performance isolation at a data center scale or enhancing job schedulers for more intelligent job placement.
Because scientific applications are often highly synchronized, the negative effects of system noise on a large-scale HPC system can present a much greater impact on performance. Reducing system noise caused by other processes such as storage is critical.
Telemetry information is powerful. It’s not just about collecting information about routers, switches, and network traffic. Rather, it is possible to gather and share information by workload and I/O characterization.
AI frameworks precisely tune the performance isolation algorithms within the NVIDIA Quantum-2 InfiniBand platform. Multi-application environments sharing common data center resources, such as the network and storage, are ensured the best possible performance, as if the applications were running on bare metal as a single instance.
BlueField is perfectly positioned to address the challenges presented by large-scale computing. For more information on DPUs, add the following GTC session to your calendar:
For more information on other technologies discussed in this post, see the following resources:
Announced at GTC, technical artists, software developers, and ML engineers can now build custom, physically accurate, synthetic data generation pipelines in the…
Announced at GTC, technical artists, software developers, and ML engineers can now build custom, physically accurate, synthetic data generation pipelines in the cloud with NVIDIA Omniverse Replicator.
Omniverse Replicator is a highly extensible framework built on the NVIDIA Omniverse platform that enables physically accurate 3D synthetic data generation to accelerate the training and accuracy of perception networks.
Omniverse Replicator is now deployable in the cloud through containers hosted on NVIDIA NGC and SaaS available for early access by application. The Replicator suite of tools and content also now features a new Replicator Insight app for enhanced viewing and inspecting of generated data, plus new SimReady content and guides for plug-and-play synthetic data workflows.
Numerous partners are integrating Omniverse Replicator in their existing tools to extend their synthetic data workflows. Siemens with their SynthAI software, SmartCow, Mirage, and Lightning AI are among the first to use Omniverse Replicator to accelerate high-quality synthetic data generation.
Synthetic data: From local to cloud
For developers and enterprises who want the flexibility and scalability of cloud deployment, Omniverse Replicator is now available as container deployments on AWS. You can become a member of NGC to access containers and self-service deploy on Amazon EC2 G5 instances featuring A10G Tensor Core GPUs.
Enhanced inspecting and viewing
Generating synthetic data and improving AI models is an iterative process requiring the ability to view and analyze generated datasets along the way. This process can be quite cumbersome as data is not easily navigable and annotations are not easily inspected.
At GTC, we released Omniverse Replicator Insight early access, an app that enables you to view, inspect, and analyze generated datasets with a range of annotations efficiently and intuitively. Replicator Insight lets you browse generated datasets from different sensors on a frame-by-frame basis, select points of interest to view, and inspect specific annotations of specific objects.
Viewing, inspection, and analysis of generated datasets is efficient and intuitive on Replicator Insight with a range of annotations. It enables you to browse through the generated datasets from different sensors on a frame-by-frame basis. You can select objects of interest to view and inspect annotations for specific objects.
Replicator Insight lets developers and researchers take a leap towards data-centric AI training, integrating synthetic data more seamlessly into the model improvement process.
New SimReady assets
Omniverse Replicator SimReady Universal Scene Description (USD) assets help you get started generating synthetic data to narrow the gap between simulation and reality:
- High-fidelity 3D assets that jump-start synthetic data generation at the pixel level.
- Contextual content assets that help train data for diversity, context, and behaviors in a scene.
Conveyor belts, ramps, and cardboard boxes are just a few examples of SimReady assets available in the Omniverse Replicator library.
You can access the first collection of free SimReady assets by downloading Omniverse today. For more information about new assets and other resources, see New Cloud Applications, SimReady Assets, and Tools for Omniverse Developers Announced at GTC.
Replicator in action
Several partners are using Omniverse Replicator to accelerate the training and performance of AI perception networks. Their applications span every phase of end-to-end synthetic data generation workflows. With Omniverse Replicator as a foundational platform for their applications, these partners are helping customers strengthen datasets and improve the accuracy of AI models for a variety of industry use cases.
Mirage is helping ML engineers understand where their dataset is weak and integrate synthetic data that fixes these weaknesses. Replicator is the backbone from which Mirage’s customers generate high-fidelity data to improve their ML models.
Lightning AI lets you build models and use or create Lightning Apps: powerful, end-to-end machine learning systems that are fully customizable. The Omniverse Replicator Lightning App lets you quickly generate synthetic data to reduce the cost and effort associated with gathering and labeling real-world data.
With Lightning AI, researchers and developers can run parallel AutoML jobs, find the best-performing object detection model, and verify performance on real-world data for synthetic data generation.
SmartCow leverages Omniverse Replicator to generate synthetic data with variations simply and effectively. Adding those variations through Replicator enables SmartCow to continuously improve model accuracy with ease. SmartCow uses Replicator in its iterative process to generate additional variations from data drift detections and create improved models.
Siemens is collaborating with NVIDIA to bring the Omniverse Replicator high-fidelity rendering capabilities and SDK to SynthAI’s cloud. This will ensure a simple, streamlined workflow from product design and collaboration to synthetic data generation and model training and ending with successful deployment.
For more information about how Siemens’ SynthAI, SmartCow, and Mirage are building on Replicator, add the How to Build a Custom Synthetic Data Pipeline to Train AI Perception Models GTC session to your calendar.
Get started building 3D synthetic generation pipelines today
You can get started with Omniverse Replicator today by downloading Omniverse and installing the Omniverse Code app.
To get hands-on training with Replicator, join the Generate Synthetic Data Using Omniverse Replicator for Perception Models DLI training lab at GTC with NVIDIA Omniverse Replicator product manager, Nyla Worker.
For more information and the latest news, see the following resources:
- In the Omniverse Resource Center, you can learn how to build custom USD-based applications and extensions for the platform.
- Follow Omniverse on Instagram, Twitter, YouTube, and Medium for additional resources and inspiration.
- Check out the Omniverse forums and join our Discord Server and Twitch to chat with the community.
- Visit the NVIDIA-Omniverse GitHub repo to explore code samples and extensions built by the community.
Recent advances in large language models (LLMs) have fueled state-of-the-art performance for NLP applications such as virtual scribes in healthcare, interactive…
Recent advances in large language models (LLMs) have fueled state-of-the-art performance for NLP applications such as virtual scribes in healthcare, interactive virtual assistants, and many more.
To simplify access to LLMs, NVIDIA has announced two services: NeMo LLM for customizing and using LLMs, and BioNeMo, which expands scientific applications of LLMs for the pharmaceutical and biotechnology industries. NVIDIA NeMo Megatron, an end-to-end framework for training and deploying LLMs, is now available to developers around the world in open beta.
NeMo LLM service
The NVIDIA NeMo LLM service provides the fastest path to customize foundation LLMs and deploy them at scale leveraging the NVIDIA managed cloud API or through private and public clouds.
NVIDIA and community-built foundation models can be customized using prompt learning capabilities, which are compute-efficient techniques, embedding context in user queries to enable greater accuracy in specific use cases. These techniques require just a few hundred samples to achieve high accuracy. Now, the promise of LLMs serving several use cases with a single model is realized.
Developers can build applications ranging from text summarization, to paraphrasing, to story generation, and many others, for specific domains and use cases. Minimal compute and technical expertise are required.
The Megatron 530B model is one of the world’s largest LLMs, with 530 billion parameters based on the GPT-3 architecture. It will soon be available to developers through the early access program on the NVIDIA NeMo LLM service. Model checkpoints will soon be available through HuggingFace and NGC, or for use through the service, including:
- T5: 3B
- NV GPT-3: 5B/20B/530B
Apply now to use NeMo LLM in early access.
Join us for the GTC 2022 session, Enabling Fast-Path to Large Language Model Based AI Applications to learn more.
BioNeMo service
The BioNeMo service, built on NeMo Megatron, is a unified cloud environment for AI-based drug discovery workflows. Chemists, biologists, and AI drug discovery researchers can generate novel therapeutics; understand their properties, structure, and function; and ultimately predict binding to a drug target.
Today, the BioNeMo service supports state-of-the-art transformer-based models for both chemistry and proteomics. Support for DNA-based workflows is coming soon. The ESM-1 architecture provides equivalent capabilities for proteins, and OpenFold is supported for ease of use and scaling of workflows for predictions of protein structures. The platform enables an end-to-end modular drug discovery workflow to accelerate research and better understand proteins, genes, and other molecules.
Learn more about NVIDIA BioNeMo.
NeMo Megatron
NVIDIA has announced new updates to NVIDIA NeMo Megatron, an end-to-end framework for training and deploying LLM up to trillions of parameters. NeMo Megatron is now available to developers in open beta, on several cloud platforms including Microsoft Azure, Amazon Web Services, and Oracle Cloud Infrastructure, as well as NVIDIA DGX SuperPODs and NVIDIA DGX Foundry.
NeMo Megatron is available as a containerized framework on NGC, offering an easy, effective, and cost-efficient path to build and deploy LLMs. It consists of an end-to-end workflow for automated distributed data processing; training large-scale customized GPT-3, T5, and multilingual T5 (mT5) models; and deploying models for inference at scale.
Its hyperparameter tool enables custom model development, automatically searching for the best hyperparameter configurations for both training and inference, on any given distributed GPU cluster configuration.
Large-scale models are made practical, delivering high training efficiency, using techniques such as tensor, data, pipeline parallelism, and sequence parallelism, alongside selective activation recomputing. It is also equipped with prompt learning techniques that enable customization for different datasets with minimal data, vastly improving performance and few-shot tasks.
Apply now to use NeMo Megatron in open beta.
Join us for the GTC 2022 session, Efficient At-Scale Training and Deployment of Large Language Models (GPT-3 and T5) to learn more about the latest advancements.
Developers, creators, and enterprises around the world are using NVIDIA Omniverse to build virtual worlds and push the boundaries of the metaverse. Based on…
Developers, creators, and enterprises around the world are using NVIDIA Omniverse to build virtual worlds and push the boundaries of the metaverse. Based on Universal Scene Description (USD), an extensible, common language for virtual worlds, Omniverse is a scalable computing platform for full-design-fidelity 3D simulation workflows that developers across global industries are using to build out the 3D internet.
During the latest GTC keynote, NVIDIA announced the largest release of new features for Omniverse to date, with Omniverse Cloud managed services and container deployments, new developer toolkits, and an open publishing portal for developers.
With these latest releases and capabilities, developers can build, extend, and connect 3D tools and platforms to the Omniverse ecosystem with greater ease than ever.
Cloud services for building and operating metaverse applications
The first NVIDIA SaaS offering, Omniverse Cloud, is an infrastructure-as-a-service that connects Omniverse applications running in the cloud, on-premises, or on edge devices. Users can create and collaborate on any device with the Omniverse App Streaming feature, access and edit shared virtual worlds with Omniverse Nucleus Cloud, and scale 3D workloads across the cloud with Omniverse Farm.
Omniverse Cloud runs on the planetary-scale Omniverse Cloud Computer. It is powered by NVIDIA OVX for graphics-rich virtual world simulation, NVIDIA HGX for advanced AI workloads, and NVIDIA Graphics Delivery Network to enable low-latency delivery of interactive 3D experiences to edge devices.
Applications for industry workflows—like NVIDIA DRIVE Sim for testing and validating autonomous vehicles and NVIDIA Isaac Sim for training and testing robots—are packaged as containers for simple deployment. For synthetic data for industry use cases, Omniverse Replicator in the cloud enables synthetic 3D data generation for perception networks.
Ready to experience Omniverse in the cloud? Access cloud containers and deploy or apply for early access to managed services.
First-class developer experience with Omniverse Kit
Omniverse Kit is a powerful toolkit for building native Omniverse applications and microservices. It is designed to be the premier foundation for new Omniverse-connected tools and microservices.
All the building blocks that developers need for building applications, extensions, and connectors for Omniverse are available in Omniverse Kit. Its modularity enables you to assemble tools in a variety of ways, depending on your unique needs. The Kit team is continuously improving the toolkit with new tools and improved user experience.
Key updates
- Viewport 2.0 is now the default, along with many improvements to omni.ui.scene and general availability of the new Viewport menu, enabling you to create your own workflow with Viewport and build your own menu for tools.
- Kit Core now supports third-party extensions in C++ and has a number of new features, including Kit Actions for easier scripting and hotkeys, and Kit Activity Monitor for a full timeline of load activity. This means you can easily bring your own C++ library into Kit and build performance-critical code.
- Kit Runtime introduces many RTX performance and quality improvements. Action Graph has user interface and user experience improvements across the board, including specific nodes for creating user interfaces.
- To get started building quickly, you can take advantage of many documentation improvements, including interactive documentation building and new samples for omni.ui, Scene, and Viewport.

Publishing portal on Omniverse Exchange
With the upcoming release of a self-publishing experience for Omniverse Exchange, you as a developer will have a powerful channel to expand the user audience for your connectors, extensions, and asset libraries. The publishing portal will provide a workflow for partners and community members to publish applications, connectors, and extensions to be featured in the Exchange.
Through Omniverse Exchange, Omniverse customers can seamlessly access industry and purpose-built third-party solutions that will accelerate and optimize their workflows. All content undergoes security vetting and quality assurance before being published.
Developers can be among the first to upload extensions or connectors to the NVIDIA Omniverse Exchange Publishing Portal through the early-access program, available by application. Community members are already taking advantage of these developer tools.
Advances to USD
NVIDIA believes that USD is the best candidate to serve as the HTML of the metaverse. USD, an open and extensible ecosystem for describing, composing, simulating, and collaborating within 3D worlds, is now being used in a wide range of industries.
To accelerate the evolution of USD to meet the needs of the metaverse and become the standard language of virtual worlds, NVIDIA is continuing to contribute to the USD ecosystem in all areas. This ranges from education to building custom schemas for specific industry use cases, while providing free USD assets and resources to all audiences.
Key updates for the NVIDIA work in USD
- Asset Resolver 2.0 support, enabling Omniverse Connector interoperability with any build of USD
- New and improved USD code snippets with documentation for common workflows
- USD C++ extension examples for Omniverse Kit, including open-source USD schema examples and tutorials (coming soon)
- Support for UTF-8 identifiers for full interchange of content encoded with international character sets (coming soon)
- An open-source text render delegate enabling human-readable debugging and more efficient unit and A/B testing of scene delegate implementations (coming soon)
NVIDIA has developed Omniverse Connectors for a breadth of top industry applications. At GTC 2022, we introduced Connectors for Autodesk Alias, Siemens JT, and Open Geospatial Consortium (OGC) Web Map Service and APIs, now available in beta. Connectors are also in development for Unity, SimScale, and some of the Siemens Xcelerator portfolio.
In addition, new Omniverse Extensions extend the functionalities of Omniverse Apps, including Motionverse from Beijing DeepScience Technology Ltd, in3D, and SmartCow. Prevu3D and Move.AI will soon have extensions available, as well.
SimReady assets for simulation workflows
NVIDIA also released SimReady, a new category of 3D asset. 3D assets for digital twins and AI training workloads need specific, USD-based properties. NVIDIA is developing thousands of SimReady assets, as well as specifications for building them, tailored to specific simulation workloads like manipulator bot training and autonomous vehicle training.
SimReady goes beyond stunning, full-fidelity visuals for 3D art assets. It includes content with attached, robust metadata that can be inserted into any Omniverse simulation and behave as it would in the real world. For developers and technical artists, SimReady content can act as a standard baseline or starting point and evolve over time as simulation tools like Omniverse become more robust.
These assets provide full-fidelity visualization with the intent of photorealism. Core simulation metadata is always included in the assets and can be readily accessed on import of the art asset. SimReady assets also leverage the modular nature of USD to provide flexibility for technical artists generating synthetic data.
SimReady asset attributes for developers
- Semantic labels to help you train simulation algorithms by identifying the various components of a 3D model in a predictable and consistent way. These labels provide ground-truth arbitrary output variables for annotations within training.
- Physical materials that bring simulations closer to reality and enable you to fine-tune how computers see the world with non-visible sensor support like lidar and radar.
- Rigid body physics for accurate mass and defined center of gravity to help technical artists create simulations that reflect real-world behavior.
- Defined taxonomy with consistent tagging for search and discoverability, so that assets can be usable across many domains.
- Robust kinematics and constraints to help you define complex, multi-part relationships and behaviors.
- Advanced EM materials to unlock behaviors across the light spectrum.
Download Omniverse to access the first collection of free SimReady assets.
Watch the GTC session, How to Build Simulation-Ready USD 3D Assets, to learn more.
Generate synthetic datasets with Omniverse Replicator
NVIDIA Omniverse Replicator is now deployable in the cloud through containers hosted on NGC and through Omniverse Cloud early access. It features a new Replicator Insight app for enhanced viewing and inspecting of generated data.
Numerous partners, including Siemens SynthAI, SmartCow, Mirage, and Lightning AI, are integrating Omniverse Replicator into their existing tools to extend their synthetic data workflows. Learn more about how Omniverse Replicator is accelerating AI training faster than ever with custom, physically-accurate synthetic data generation pipelines.
Boost rendering performance with Ada Lovelace GPU
Announced at GTC, the new NVIDIA Ada Lovelace GPU delivers real-time rendering, graphics, and AI that will help technical designers and developers build larger virtual worlds. With a more than 2x boost in rendering performance from the previous-generation NVIDIA RTX A6000, NVIDIA Ada Lovelace enables real-time path tracing in 4K resolution. It also enables users to edit and operate large-scale worlds interactively and experience updates to virtual worlds in real time.
A new era of neural graphics
Neural graphics can change the way content is created and experienced. AI-powered tools on NVIDIA AI ToyBox now enable creators to experiment with the latest research in their 3D workflows. AI Animal Explorer is available now, with updates coming soon, including:
- GANverse3D with GAN-based and diffusion model-based experimental AI tools
- AI Car Explorer with meshed/textured car models by attributes like make and style
- Canvas360 with 360-degree landscapes and backgrounds for 3D design
Procedural behavior in Omniverse
Omniverse now enables proceduralism for behavior, movement, and motion in Omniverse worlds. Behavior and physics simulation technologies have improved ease of use, refinement, and functionality. With the new OmniGraph node-based physics systems, behavior, motion, and action can be entirely procedural without any manual animation.
Powerful, flexible simulation with PhysX
NVIDIA PhysX is an advanced real-time physics engine in Omniverse. Several key updates were announced at GTC, including:
- Automation improvements and a physics toolbar for object creation
- Support for multiple scenes with the ability to put individual assets and stages into their own physics scene
- Audio for physics collisions
- Ability to author vehicles and force fields in OmniGraph
- CPU bottleneck reduction and GPU improvements for soft body simulation
- Ability to simulate collisions for particle systems
Expanding ecosystem of extensions with Omniverse Code Contest
This summer, developers from around the world used Omniverse Code to build extensions for 3D worlds as part of the NVIDIA #ExtendOmniverse Contest. Exciting new tools were submitted for layout and scene authoring, Omni.ui, and scene modifier and manipulator tools.
Winners of the contest will be announced at GTC during the NVIDIA Omniverse User Group on September 20 at 3:00 PM (Pacific time).
Explore more at NVIDIA GTC
Join panels, talks, and hands-on labs for developers at GTC to learn more about Omniverse and other tools developers are using to build metaverse extensions and applications.
Watch the latest GTC keynote by NVIDIA founder and CEO Jensen Huang to hear about the latest advancements in AI and the metaverse.
Visit the Omniverse Resource Center to learn how you can build custom USD-based applications and extensions for the platform.
Follow Omniverse on Instagram, Twitter, YouTube, and Medium for additional resources and inspiration. Check out the Omniverse forums, Discord Server, and Twitch channel to chat with the community. Visit NVIDIA-Omniverse GitHub repo to explore code samples and extensions built by the community.