NVIDIA today announced NVIDIA® DLSS 3, an AI-powered performance multiplier that kicks off a new era of NVIDIA RTX™ neural rendering for games and applications.
NVIDIA today unveiled the GeForce RTX® 40 Series of GPUs, designed to deliver revolutionary performance for gamers and creators, led by its new flagship, the RTX 4090 GPU, with up to 4x the performance of its predecessor.
Opening a new era of neural graphics that marries AI and simulation, NVIDIA today announced the NVIDIA RTX™ 6000 workstation GPU, based on its new NVIDIA Ada Lovelace architecture.
Telecoms began touting the benefits of 5G networks six years ago. Yet the race to deliver ultrafast wireless internet today resembles a contest between the tortoise and the hare, as some mobile network operators struggle with costly and complex network requirements. Advanced data analytics company HEAVY.AI today unveiled solutions to put carriers on more even Read article >
The post HEAVY.AI Delivers Digital Twin for Telco Network Planning and Operations Based on NVIDIA Omniverse appeared first on NVIDIA Blog.
With tens of millions of weekly transactions across its more than 2,000 stores, Lowe’s helps customers achieve their home-improvement goals. Now, the Fortune 50 retailer is experimenting with high-tech methods to elevate both the associate and customer experience. Using NVIDIA Omniverse Enterprise to visualize and interact with a store’s digital data, Lowe’s is testing digital Read article >
The post Reinventing Retail: Lowe’s Teams With NVIDIA and Magic Leap to Create Interactive Store Digital Twins appeared first on NVIDIA Blog.
Deutsche Bahn’s rail network consists of 5,700 stations and 33,000 kilometers of track, making it the largest in Western Europe. Digitale Schiene Deutschland (Digital Rail for Germany, or DSD), part of Germany’s national railway operator Deutsche Bahn, is working to increase the network’s capacity without building new tracks. It’s striving to create a powerful railway Read article >
The post On Track: Digitale Schiene Deutschland Building Digital Twin of Rail Network in NVIDIA Omniverse appeared first on NVIDIA Blog.
NVIDIA today announced its first software- and infrastructure-as-a-service offering — NVIDIA Omniverse™ Cloud — a comprehensive suite of cloud services for artists, developers and enterprise teams to design, publish, operate and experience metaverse applications anywhere.
NVIDIA today introduced NVIDIA DRIVE™ Thor, its next-generation centralized computer for safe and secure autonomous vehicles.
Canon, John Deere, Microsoft Azure, Teradyne, TK Elevator Join Over 1,000 Customers Adopting Jetson Orin Family Within Six Months of Launch SANTA CLARA, Calif., Sept. 20, 2022 (GLOBE NEWSWIRE) …
An AI model card is a document that details how machine learning (ML) models work. Model cards provide detailed information about the ML model’s metadata…
An AI model card is a document that details how machine learning (ML) models work. Model cards provide detailed information about the ML model’s metadata including the datasets that it is based on, performance measures that it was trained on, and the deep learning training methodology itself. This post walks you through the current practice for AI model cards and how NVIDIA is planning to advance them with Model Card++, the enhanced next-generation AI model card.
In their 2019 paper, Model Cards for Model Reporting, a group of data scientists, including Margaret Mitchell, Timnit Gebru, and Lucy Vasserman, sought to create a documentation standard for AI models. Their primary motivation was to promote transparency and accountability in the AI model development process by disclosing essential information about an AI model.
This information includes who developed the model, intended use cases and out-of-scope applications, expected users, how the model performs with different demographic groups, information about the data used to train and verify the model, limitations, and ethical considerations.
Until the development of the first AI model card, little information was shared about a particular AI model to help determine whether the model was suitable for a particular organization’s purpose.
This becomes problematic if the output of a model could have an adverse impact on a particular group of people. For example, the 2019 university-led study, Discrimination through Optimization: How Facebook’s Ad Delivery Can Lead to Skewed Outcomes revealed that algorithms for delivering ads on social media resulted in discriminatory ad delivery despite the use of neutral parameters for targeting the ads.
The adoption of model cards helps the development and improvement of models by allowing developers to compare their results to those of similar models. Model cards highlight performance problems for those who plan to deploy a model.
At the same time, model cards also educate policymakers who are drafting regulations and legislation governing AI models and systems. Although not required, developing model cards is a best practice that encourages developers to engage with the people who will ultimately be impacted by the model’s output.
The importance of model cards
While model cards are designed to encourage model transparency and trustworthiness, they are also used by stakeholders to improve developer understanding and standardize the decision-making processes.
Model cards are structured and organized in a schema. They concisely report information on different factors like demographics, environmental conditions, quantitative evaluation metrics, and, where provided, ethical considerations.
Model cards can also record model version, type, date, license restrictions, information about the publishing organization, and other qualitative information. Model cards are designed to educate and allow an informed comparison of measures and benchmarks. Figure 1 shows the NGC Model Card for StyleGAN3.
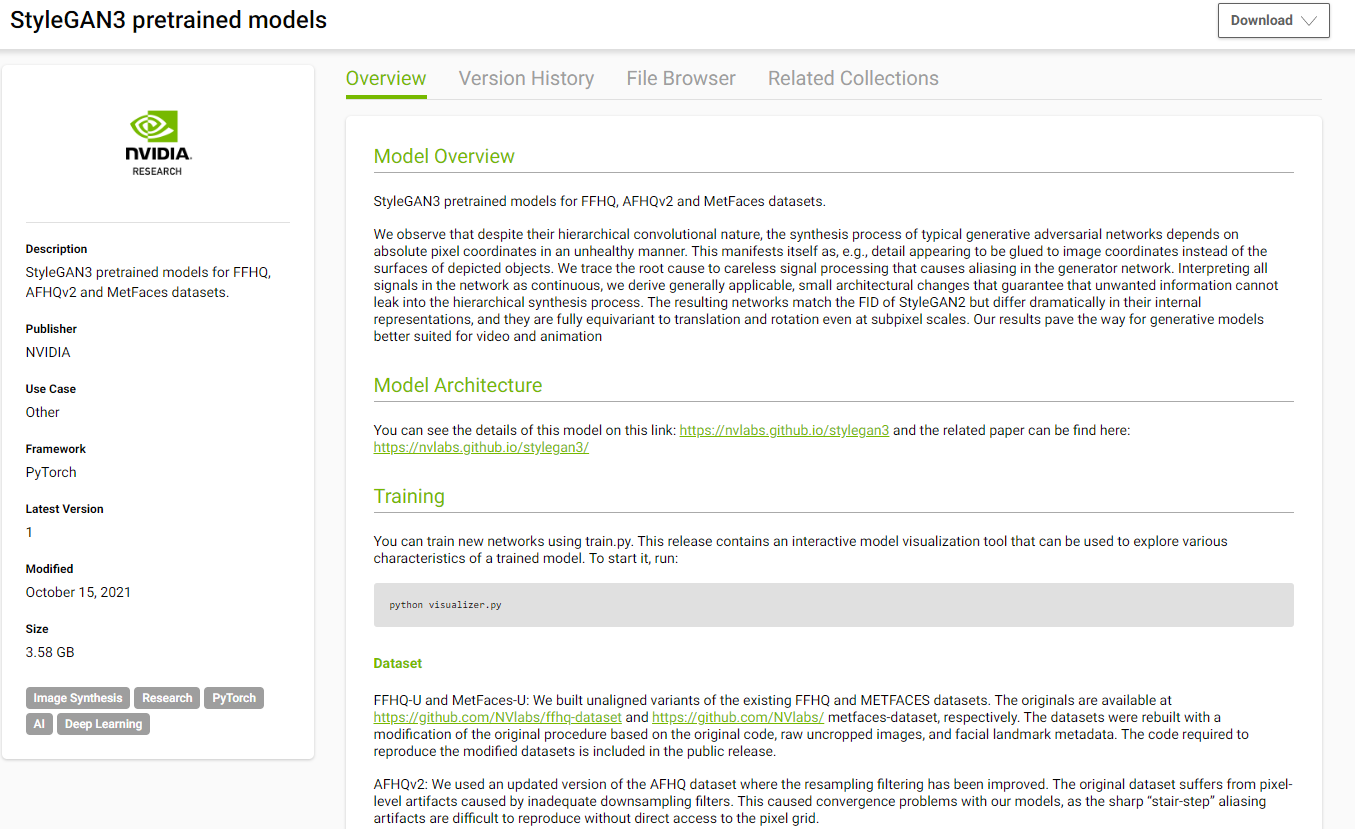
Model cards are like open-source fact sheets. Unless you are the developer of the model itself, you would likely not even know much about the AI model itself without model cards. Model cards provide the most comprehensive understanding of a model’s details and considerations individuals should take into account for its application.
For instance, a smartphone might have a face detection system that allows the user to unlock it based on recognition. Without model cards, model developers might not realize how a model will behave until it is deployed. This is what happened when Dr. Joy Buolamwini tried to use a face detection system as part of her graduate work at MIT.
AI model card accessibility
AI model cards should not just be built for developers; companies should also build model cards that are accessible to and readable by nontechnical individuals and technical experts alike.
Model cards are not restricted to a given industry or domain. They can be used for computer vision, speech, recommender systems, and other AI workflows. In addition to having active use in higher education and research and high performance computing spaces, model cards have utility across multiple industries including automotive, healthcare, and robotics applications. Model cards can:
- Teach students and help them understand real-world use cases
- Inform policymakers and clarify intended use for non-model developers
- Educate those interested in seeking the benefits of AI
Mobilizing AI through model cards is a decisive and transparent step that companies can take toward the advancement of trustworthy AI.
Improving and enhancing model cards
We conducted market research to inform the improvements to existing model cards. While 90% of respondents in the developer sample agree that model cards are important and 70% would recommend them as-is, there is room for improvement to drive their adoption, use, and impact.
Based on our research, existing model cards should be enhanced in two primary areas: accessibility and content quality. Model card users need model cards to be easily accessible and understandable.
Accessibility
Discovery is one element of model card accessibility that needs improvement. In releasing models, AI developers should be able to find and then promote model cards alongside their work. This is true of models introduced in research papers as well as models deployed for commercial use.
Secondly, model cards need to be located where interested individuals can reference them. One of the ways NVIDIA promotes model cards is through the NGC Catalog. Models and model cards are located side-by-side in this same repository.
Content quality
After a model card has been located, the next challenge for the user is understanding the information contained in it. This is particularly critical in the model evaluation stage before selection. Not understanding the information contained in the model card leads to the same outcome as not knowing that the information exists; either way, model users cannot make informed decisions.
To address this, NVIDIA encourages using a consistent organizational structure, simple format, and clear language for model cards. Adding filterable and searchable fields is also recommended. When individuals can find the information contained in model cards, they are more likely to understand the software. According to our research, respondents liked and relied on the information contained in the model card when it was easily accessible and understandable.
In fact, performance and licensing information were the two most important areas respondents wanted to see in model cards. Figure 2 shows how the StyleGAN3 model card devotes separate sections to performance and licensing.

After performance and licensing information, respondents felt that the section on ethical considerations was the most important category of information to include in model selection criteria. Within ethical considerations, respondents shared that they wanted more information about the datasets used to train and validate the model—particularly details regarding unwanted bias—as well as information about safety and security.
Overview of Model Card++
Model Card++ is the improved NGC Catalog Model Card prototype that NVIDIA has developed over the last 9 months. In addition to the typical information given in the Overview section of a model card in the NGC Catalog, Model Card++ incorporates:
- The Plus Plus Promise (also known as the ++ Promise or the Triple P), describing the NVIDIA software development approach and the standards we hold ourselves to in all model development
- Subsections detailing model-specific information concerning bias, explainability, privacy, safety, and security
Figure 4 shows the ++ Promise, which will be embedded in every Model Card++.
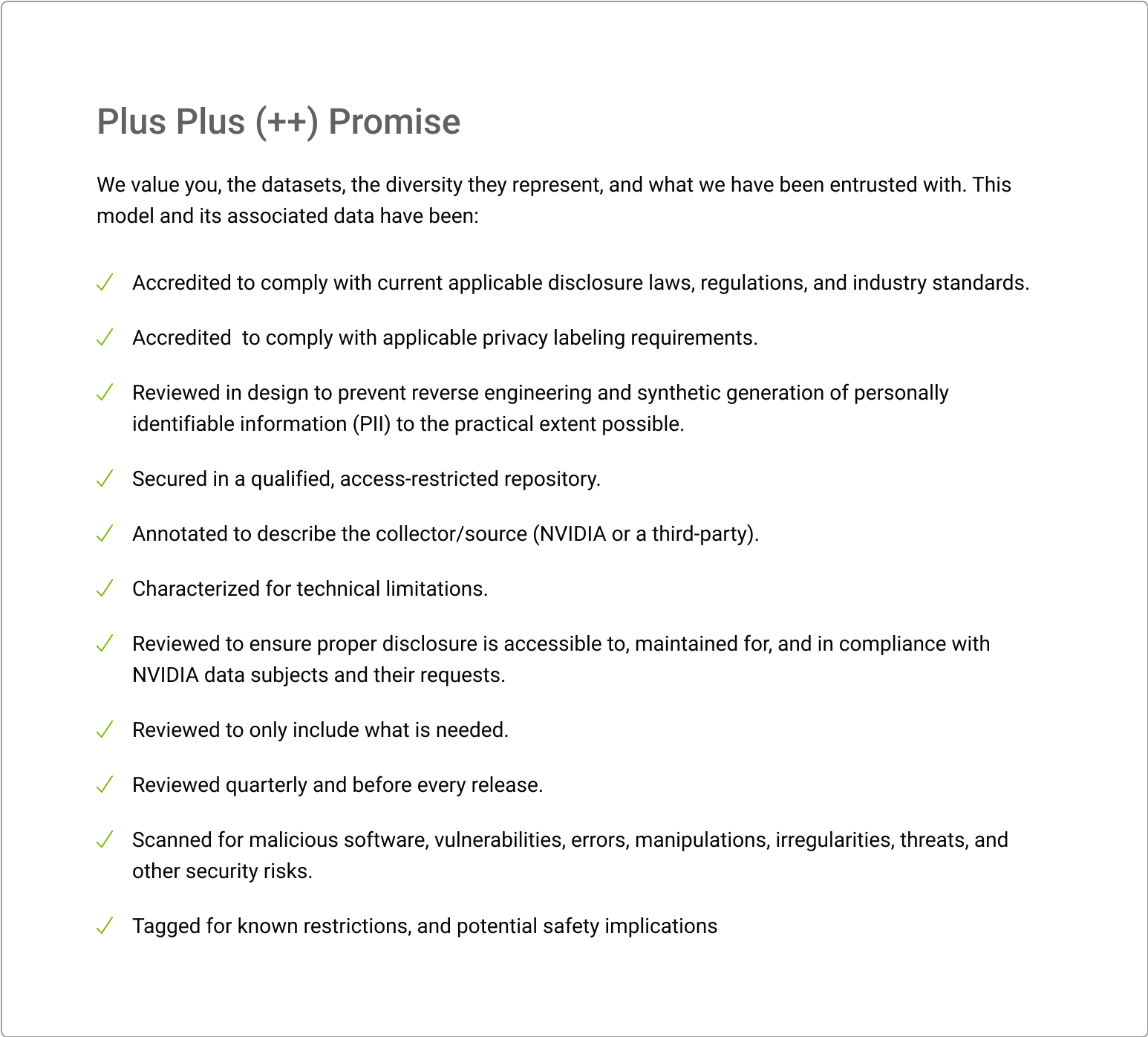
The ++ Promise describes the steps that NVIDIA is taking to demonstrate the trustworthiness of our work embedded in design. The subcards outline:
- Steps taken to mitigate unwanted bias
- Decision logic and example domains
- Provenance of training datasets and what type of data was collected and how
- Development controls used and known restrictions
This is not an exhaustive list but demonstrates the intent by design and commitment to standards and protections that value individuals, the data, and the NVIDIA contribution to AI. This applies to every model, across domains and use cases.
Figure 5 shows an example of the Explainability subcard. Each Model Card++ will include a dedicated section of fields and responses for each of the subsections. What is shown in the response section for each field is not meant to represent a real-world model, but to illustrate what will be provided based on current understanding and the latest research.
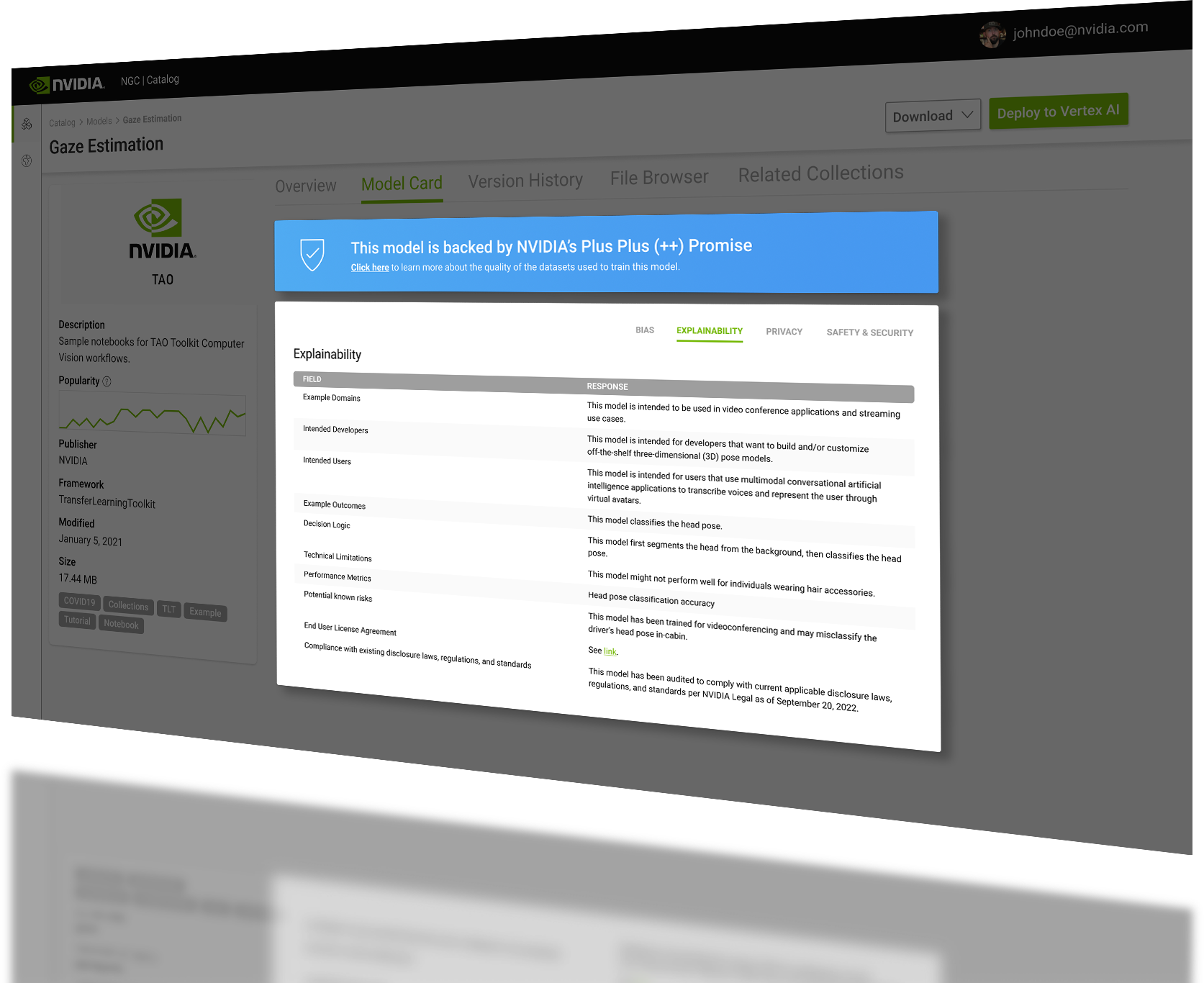
The Explainability subcard gives information about example domains for an AI model, intended users, decision logic, and compliance review. NVIDIA model cards aim to present AI models using clear, consistent, and concise language.
NVIDIA will start rolling out Model Card++ by the end of the year, with all commercial models using it by the end of 2023.
How we built Model Card++
Model Card++ is the next generation of AI model card. It is the result of a disciplined, cross-functional approach in partnership with engineering, product, research, product security, and legal teams. Building on existing NGC model cards, we reviewed model cards from other organizations and templates, including GitHub, to find out what other information could be provided consistently.
We worked with engineering to pilot what could be consistently provided in addition to information that is currently provided. We discovered that although our model cards have a section for ethical considerations, there is more that can be provided, like measures we took to mitigate unwanted bias. We also found we could describe dataset provenance and traceability, dataset storage, and quality validation.
We look to provide more details about dataset demographic make-up, performance metrics for different demographic groups, and specific mitigation efforts we have taken to address unwanted bias. We also worked with an algorithmic bias consultant to develop a process for assessing unwanted bias that is compliant with data privacy laws and coupled that with our latest market research.
As we built Model Card++, we also corroborated our work with market research by surveying developers who used our models and those from across the industry. We validated the desired information and structured it with our user design experience team to present it in a clear and organized format. We are excited about introducing Model Card++ to the world and hope to continue leading efforts that encourage inclusive AI for all.
Get the latest updates about Model Card++ at the September GTC 2022 session, Ingredients of Trust: Moving towards Model Card++.