NVIDIA DGX Cloud — which delivers tools that can turn nearly any company into an AI company — is now broadly available, with thousands of NVIDIA GPUs online on Oracle Cloud Infrastructure, as well as NVIDIA infrastructure located in the U.S. and U.K. Unveiled at NVIDIA’s GTC conference in March, DGX Cloud is an AI Read article >
SANTA CLARA, Calif., July 24, 2023 (GLOBE NEWSWIRE) — NVIDIA today announced that it has named to its board of directors Melissa Lora, who spent three decades as an executive at Taco Bell …
Real-time processing of network traffic can be leveraged by the high degree of parallelism GPUs offer. Optimizing packet acquisition or transmission in these…
Real-time processing of network traffic can be leveraged by the high degree of parallelism GPUs offer. Optimizing packet acquisition or transmission in these types of applications avoids bottlenecks and enables the overall execution to keep up with high-speed networks. In this context, DOCA GPUNetIO promotes the GPU as an independent component that can exercise network and compute tasks without the intervention of the CPU.
This post provides a list of GPU packet processing applications focusing on different and unrelated contexts where NVIDIA DOCA GPUNetIO has been integrated to lower latency and maximize performance.
NVIDIA DOCA GPUNetIO API
NVIDIA DOCA GPUNetIO is one of the new libraries released with the NVIDIA DOCA software framework. The DOCA GPUNetIO library enables direct communication between the NIC and the GPU through one or more CUDA kernels. This removes the CPU from the critical path.
Using the CUDA device functions in the DOCA GPUNetIO library, a CUDA kernel can send and receive packets directly from and to the GPU without the need of CPU cores or memory. Key features of this library include:
- GPUDirect Async Kernel-Initiated Network (GDAKIN): Communications over Ethernet; GPU (CUDA kernel) can directly interact with the network card to send or receive packets in GPU memory (GPUDirect RDMA) without the intervention of the CPU.
- GPU memory exposure: Combine within a single function the basic CUDA memory allocation feature with the GDRCopy library to expose a GPU memory buffer to the direct access (read or write) from the CPU without using CUDA API.
- Accurate Send Scheduling: From the GPU, it’s possible to schedule the transmission of a burst of packets in the future, associate a timestamp to it, and provide this information to the network card, which will in turn take care of sending packets at the right time.
- Semaphores: Useful message passing object to share information and synchronize across different CUDA kernels or between a CUDA kernel and a CPU thread.
For a deep dive into the principles and benefits of DOCA GPUNetIO, see Inline GPU Packet Processing with NVIDIA DOCA GPUNetIO. For more details about DOCA GPUNetIO API, refer to the DOCA GPUNetIO SDK Programming Guide.
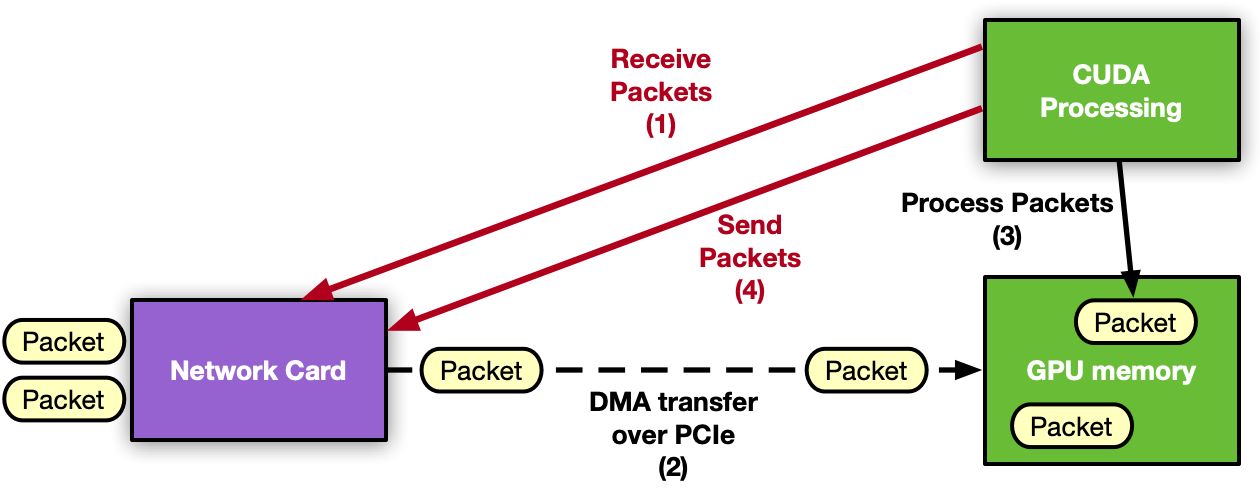
Along with the library, the following NVIDIA DOCA application and NVIDIA DOCA sample show how to use functions and features offered by the library.
- NVIDIA DOCA application: A GPU packet processing application that can detect, manage, filter, and analyze UDP, TCP, and ICMP traffic. The application also implements an HTTP over TCP server. With a simple HTTP client (curl or wget, for example), it’s possible to establish a TCP three-way handshake connection and ask for simple HTML pages through HTTP GET requests to the GPU.
- NVIDIA DOCA sample: GPU send-only example that shows how to use the Accurate Send Scheduling feature (system configuration, functions to use).
DOCA GPUNetIO in real-world applications
DOCA GPUNetIO has been used to empower the NVIDIA Aerial SDK to send and receive using the GPU, getting rid of the CPU. For more details, see Inline GPU Packet Processing with NVIDIA DOCA GPUNetIO. The sections below provide new examples that successfully use DOCA GPUNetIO to leverage the GPU packet acquisition with the GDAKIN technique.
NVIDIA Morpheus AI
NVIDIA Morpheus is a performance-oriented, application framework that enables cybersecurity developers to create fully optimized AI pipelines for filtering, processing, and classifying large volumes of real-time data. The framework abstracts GPU and CPU parallelism and concurrency through an accessible programming model consisting of Python and C++ APIs.
Leveraging this framework, developers can quickly construct arbitrary data pipelines composed of stages that acquire, mutate, or publish data for a downstream consumer. You can apply Morpheus in different contexts including malware detection, phishing/spear phishing detection, ransomware detection, and many others. Its flexibility and high performance are ideal for real-time network traffic analysis.
For the network monitoring use case, the NVIDIA Morpheus team recently integrated the DOCA framework to implement a high-speed, low-latency GPU packet acquisition source stage to feed real-time packets to an AI-pipeline responsible for analyzing packets’ contents. For more details, visit Morpheus on GitHub.

As shown in Figure 2, GPU packet acquisition happens in real time. Through DOCA Flow, flow steering rules are applied to the Ethernet receive queues, meaning queues can only receive specific types of packets (TCP, for example). Morpheus launches a CUDA kernel, which performs the following steps in a loop:
- Receive packets using DOCA GPUNetIO receive function
- Filter and analyze in parallel packets in GPU memory
- Copy relevant packets info in a list of GPU memory buffers
- The related DOCA GPUNetIO semaphore item is set to READY when a buffer has accumulated enough packets of information
- The CUDA kernel in front of the AI pipeline is polling the semaphore item
- When the item is READY, AI is unblocked as packet information is ready in the buffer
The GTC session Defensive Cyber Operations (DCO) on Edge Networks presents a concrete example in which this architecture is leveraged to deploy a high-performance, AI-enabled SPAN/Network TAP solution. This solution was motivated by the daunting data rates in information technology (IT) and operational technology (OT) networks, heterogeneity of Layer 7 application data, and edge computing size, weight, and power (SWaP) constraints.
In the case of edge computing, many organizations are unable to “burst to cloud” when the compute demand increases, especially on disconnected edge networks. This scenario requires designing an architecture for I/O and compute challenges that deliver performance across the SWaP spectrum.
This DCO example addresses these constraints through the lens of a common cybersecurity problem, identifying leaked data (leaked passwords, secret keys, and PII, for example) in unencrypted TCP traffic, and represents an extension of the Morpheus SID demo. Identifying and remediating these vulnerabilities reduces the attack surface and increases the security posture of organizations.
In this example, the DCO solution receives packets into a heterogeneous Morpheus pipeline (GPU and concurrent CPU stages written in a mix of Python and C++) that applies a transformer model to detect leaked sensitive data in Layer 7 application data. It integrates outputs with the ELK stack, including an intuitive visualization for Security Operations Center (SOC) analysts to exploit (Figures 3 and 4).

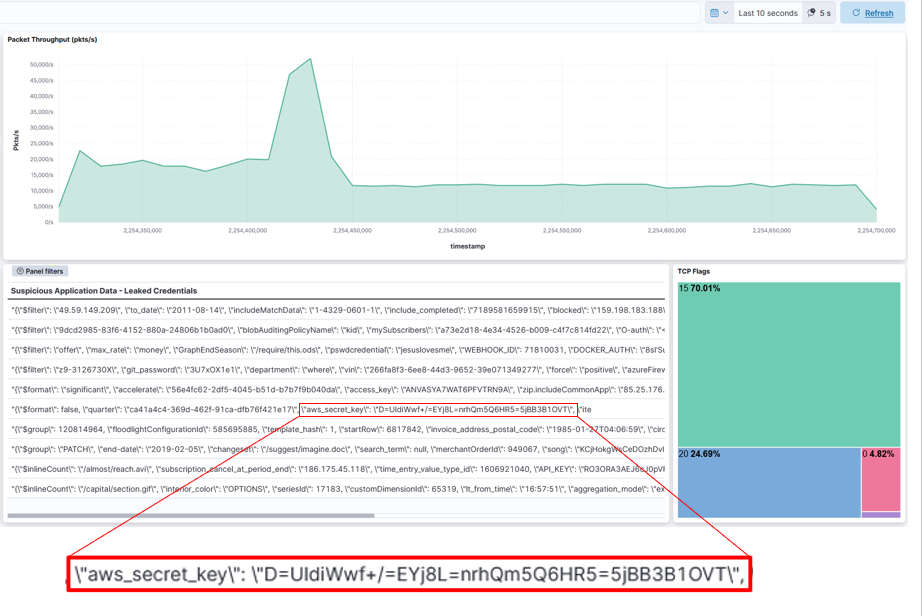
The experimental setup included cloud-native UDP multicast and REST applications running on VMs with a 100 Gbps NVIDIA BlueField-2 DPU. These applications communicated through a SWaP-efficient NVIDIA Spectrum SN2100 Ethernet switch. Packet generators injected sensitive data into packets transmitted by these applications. Network packets were aggregated and mirrored off a SPAN port on the NVIDIA Spectrum SN2100 and sent to an NVIDIA A30X converged accelerator powering the Morpheus packet inspection pipeline achieving impressive throughput results.
- This pipeline includes several components from I/O, packet filtering, packet processing, and indexing in a third-party SIEM platform (Elasticsearch). Focusing only on the I/O aspects, DOCA GPUNetIO enables Morpheus to receive packets into GPU memory at up to 100 Gbps with a single receive queue, removing a critical bottleneck in cyber packet processing applications.
- Leveraging stage level concurrency, the pipeline demonstrated a 60% boost in Elasticsearch indexing throughput.
- Running the end-to-end data pipeline on the NVIDIA A30X converged accelerator generated enriched packets at ~50% capacity of the elasticsearch indexer. Using twice as many A30Xs would fully saturate the indexer, providing a convenient scaling heuristic.
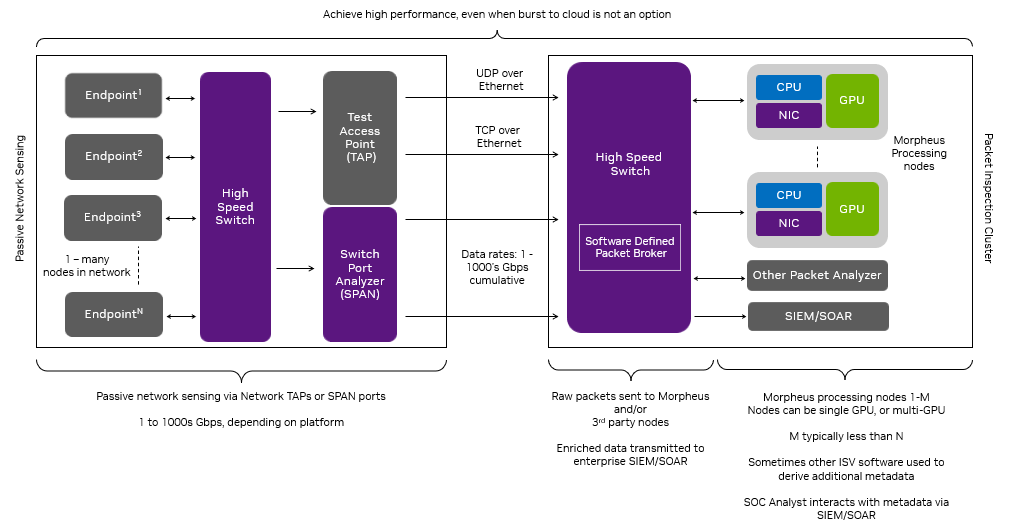
While this use case demonstrates a specific application of Morpheus, it represents the foundational components for cyber packet processing applications. Morpheus plus DOCA GPUNetIO together provide the performance and extensibility for a huge number of latency-sensitive and compute-intensive packet processing applications.
Line-rate radar signal processing
This section walks through an example in which the radar detection application ingests downconverted I/Q samples from a simulated range-only radar system at line rate of 100 Gbps, performing all required signal processing needed to convert the received I/Q RF samples into object detections in real time.
Remote sensing applications such as radar, lidar, and optical platforms rely on signal processing algorithms to turn the raw data collected from the environment they are measuring into actionable information. These algorithms are often highly parallelizable and require a high computational load, making them ideal for GPU-based processing.
Additionally, input sensors generate enormous quantities of raw data, meaning the ingress/egress capability of the processing solution must be able to handle very high bandwidths at low latencies.
Further complicating the problem, many edge-based sensor systems have strict SWaP constraints, restricting the number and power of available CPU cores that might be used in other high-throughput networking approaches, such as DPDK-based GPUDirect RDMA.
DOCA GPUNetIO enables the GPU to directly handle the networking load as well as the signal processing required to make a real-time sensor streaming application successful.
Commonly used signal processing algorithms were used in the radar detection application. The flowchart in Figure 6 shows a graphical representation of the signal processing pipeline being used to convert the I/Q samples into detections.

MTI filtering is a common technique used to eliminate stationary background clutter, such as the ground or buildings, from the reflected RF waveform in radar systems. The approach used here is known as the Three-Pulse Canceler, which is simply a convolution of the I/Q data in the pulse dimension with the filter coefficients ‘[+1, -2, +1].’
Pulse Compression maximizes the signal-to-noise ratio (SNR) of the received waveform with respect to the presence of targets. It is performed by computing the cross-correlation of the received RF data with the transmitted waveform.
Constant False Alarm Rate (CFAR) detectors compute an empirical estimate of the noise, localized to each range bin of the filtered data. The power of each bin is then compared to the noise and declared a detection if it is statistically likely given the noise estimate and distribution.
A 3D buffer of size (# Waveforms) x (# Channels) x (# Samples) is used to hold the organized RF data being received (note that applying the MTI filter upon packet receipt reduces the size of the pulse dimension to 1). No ordering is assumed to the UDP data streaming in, except that it is streamed roughly in order of the packets’ ascending waveform ID. Around 500 complex samples are transmitted per-packet, and the samples’ location in the 3D buffer is dependent on the waveform ID, channel ID, and sample index.
This application runs two CUDA kernels and one CPU core persistently. The first CUDA kernel is responsible for using the DOCA GPUNetIO API to read packets from the NIC onto the GPU. The second CUDA kernel places packet data into the correct memory location based on metadata in the packets header and applies the MTI filter, and the CPU core is responsible for launching the CUDA kernels that handle Pulse Compression and CFAR. The FFTs were performed using the cuFFT library.
Figure 7 shows a graphical representation of the application.

The throughput of the radar detection pipeline is >100 Gbps. Running at the line rate of 100 Gbps for 1 million 16-channel waveforms, no packets were dropped and the signal processing never fell behind the throughput of the data stream. The latency, measured from when the last data packet for an independent waveform ID was received, was on the order of 3 milliseconds. An NVIDIA ConnectX-6 Dx SmartNIC and an NVIDIA A100 80 GB GPU were used. Data was sent through UDP packets over Ethernet.
Future work will evaluate the performance of this architecture when running exclusively on a BlueField DPU with an integrated GPU.
Real-time DSP services over GPU
Analog signals are everywhere, both artificial (Wi-Fi radio, for example) and natural (solar radiation and earthquakes, for example). To capture analog data digitally, sound waves must be converted using a D-A converter, controlled by parameters such as sample rate and sample bit depth. Digital audio and video can be processed with FFT, allowing sound designers to use tools such as an equalizer (EQ) to alter general characteristics of the signal.
This example explains how NVIDIA products and SDK were used to perform real-time audio DSP with GPU over the network. To do so, the team built a client that parses a WAV file, frames data into multiple Ethernet packets, and sends them over the network to a server application. This application is responsible for receiving packets, applying FFT, manipulating the audio signal, and finally sending back the modified data.
The client’s responsibility is recognizing which portion should be sent to the “server” for the signal processing chain and how to treat the processed samples when they are received back from the server. This approach supports multiple DSP algorithms, such as overlap-add, and various sample window selections.
The server application uses DOCA GPUNetIO to receive packets in GPU memory from a CUDA kernel. When a subset of packets has been received, the CUDA kernel in parallel applies the FFT through the cuFFTDx library to each packet’s payload. In parallel, to each packet, a different CUDA thread applies a frequency filter reducing the amplitude of low or high frequencies. Basically, it applies a low-pass or high-pass filter.

An inverse FFT is applied to each packet. Through DOCA GPUNetIO, the CUDA kernel sends back to the client the modified packets. The client reorders packets and rebuilds them to recreate an audible and reproducible WAV audio file with sound effects applied.
Using the client, the team could tweak parameters to optimize performance and the quality of the audio output. It is possible to separate the flows and multiplex streams into their processing chains, thus offloading many complex computations into the GPU. This is just scratching the potential of this solution, which could open new market opportunities for cloud DSP service providers.
Summary
DOCA GPUNetIO library promotes a generic GPU-centric approach for both packets’ acquisition and transmission in network applications exercising real-time traffic analysis. This post demonstrates how this library can be adopted in a wide range of applications from different contexts, providing huge improvements for latency, throughput, and system resource utilization.
To learn more about GPU packet processing and GPUNetIO, see the following resources:
On Aug. 8, Jensen Huang features new NVIDIA technologies and award-winning research for content creation.
On Aug. 8, Jensen Huang features new NVIDIA technologies and award-winning research for content creation.
Google at ICML 2023
Groups across Google actively pursue research in the field of machine learning (ML), ranging from theory and application. We build ML systems to solve deep scientific and engineering challenges in areas of language, music, visual processing, algorithm development, and more. We aim to build a more collaborative ecosystem with the broader ML research community through open-sourcing tools and datasets, publishing our work, and actively participating in conferences.
Google is proud to be a Diamond Sponsor of the 40th International Conference on Machine Learning (ICML 2023), a premier annual conference, which is being held this week in Honolulu, Hawaii. As a leader in ML research, Google has a strong presence at this year’s conference with over 120 accepted papers and active involvement in a number of workshops and tutorials. Google is also proud to be a Platinum Sponsor for both the LatinX in AI and Women in Machine Learning workshops. We look forward to sharing some of our extensive ML research and expanding our partnership with the broader ML research community.
Registered for ICML 2023? We hope you’ll visit the Google booth to learn more about the exciting work, creativity, and fun that goes into solving a portion of the field’s most interesting challenges. Visit the @GoogleAI Twitter account to find out about Google booth activities (e.g., demos and Q&A sessions). See Google DeepMind’s blog to learn about their technical participation at ICML 2023.
Take a look below to learn more about the Google research being presented at ICML 2023 (Google affiliations in bold).
Board and Organizing Committee
Board Members include: Corinna Cortes, Hugo Larochelle
Tutorial Chairs include: Hanie Sedghi
Google Research booth activities
Presenters: Bryan Perozzi, Anton Tsitsulin, Brandon Mayer
Title: Unsupervised Graph Embedding @ Google (paper, EXPO workshop)
Tuesday, July 25th at 10:30 AM HST
Presenters: Zheng Xu
Title: Federated Learning of Gboard Language Models with Differential Privacy (paper 1, paper 2, blog post)
Tuesday, July 25th at 3:30 PM HST
Presenters: Thomas Kipf
Title: Self-supervised scene understanding (paper 1, paper 2)
Wednesday, July 26th at 10:30 AM HST
Presenters: Johannes von Oswald, Max Vladymyrov
Title: Transformers learn in-context by gradient descent (paper)
Wednesday, July 26th at 3:30 PM HST
Accepted papers
Scaling Vision Transformers to 22 Billion Parameters (see blog post)
Mostafa Dehghani, Josip Djolonga, Basil Mustafa, Piotr Padlewski, Jonathan Heek, Justin Gilmer, Andreas Steiner, Mathilde Caron, Robert Geirhos, Ibrahim Alabdulmohsin, Rodolphe Jenatton, Lucas Beyer, Michael Tschannen, Anurag Arnab, Xiao Wang, Carlos Riquelme, Matthias Minderer, Joan Puigcerver, Utku Evci, Manoj Kumar, Sjoerd van Steenkiste, Gamaleldin F. Elsayed, Aravindh Mahendran, Fisher Yu, Avital Oliver, Fantine Huot, Jasmijn Bastings, Mark Patrick Collier, Alexey Gritsenko, Vighnesh Birodkar, Cristina Vasconcelos, Yi Tay, Thomas Mensink, Alexander Kolesnikov, Filip Pavetić, Dustin Tran, Thomas Kipf, Mario Lučić, Xiaohua Zhai, Daniel Keysers, Jeremiah Harmsen, Neil Houlsby
Fast Inference from Transformers via Speculative Decoding
Yaniv Leviathan, Matan Kalman, Yossi Matias
Best of Both Worlds Policy Optimization
Christoph Dann, Chen-Yu Wei, Julian Zimmert
Inflow, Outflow, and Reciprocity in Machine Learning
Mukund Sundararajan, Walid Krichene
Transformers Learn In-Context by Gradient Descent
Johannes von Oswald, Eyvind Niklasson, Ettore Randazzo, João Sacramento, Alexander Mordvintsev, Andrey Zhmoginov, Max Vladymyrov
Arithmetic Sampling: Parallel Diverse Decoding for Large Language Models
Luke Vilnis, Yury Zemlyanskiy, Patrick Murray*, Alexandre Passos*, Sumit Sanghai
Differentially Private Hierarchical Clustering with Provable Approximation Guarantees (see blog post)
Jacob Imola*, Alessandro Epasto, Mohammad Mahdian, Vincent Cohen-Addad, Vahab Mirrokni
Multi-Epoch Matrix Factorization Mechanisms for Private Machine Learning
Christopher A. Choquette-Choo, H. Brendan McMahan, Keith Rush, Abhradeep Thakurta
Random Classification Noise Does Not Defeat All Convex Potential Boosters Irrespective of Model Choice
Yishay Mansour, Richard Nock, Robert Williamson
Simplex Random Features
Isaac Reid, Krzysztof Choromanski, Valerii Likhosherstov, Adrian Weller
Pix2Struct: Screenshot Parsing as Pretraining for Visual Language Understanding
Kenton Lee, Mandar Joshi, Iulia Turc, Hexiang Hu, Fangyu Liu, Julian Eisenschlos, Urvashi Khandelwal, Peter Shaw, Ming-Wei Chang, Kristina Toutanova
Mu2SLAM: Multitask, Multilingual Speech and Language Models
Yong Cheng, Yu Zhang, Melvin Johnson, Wolfgang Macherey, Ankur Bapna
Robust Budget Pacing with a Single Sample
Santiago Balseiro, Rachitesh Kumar*, Vahab Mirrokni, Balasubramanian Sivan, Di Wang
A Statistical Perspective on Retrieval-Based Models
Soumya Basu, Ankit Singh Rawat, Manzil Zaheer
Approximately Optimal Core Shapes for Tensor Decompositions
Mehrdad Ghadiri, Matthew Fahrbach, Gang Fu, Vahab Mirrokni
Efficient List-Decodable Regression Using Batches
Abhimanyu Das, Ayush Jain*, Weihao Kong, Rajat Sen
Efficient Training of Language Models Using Few-Shot Learning
Sashank J. Reddi, Sobhan Miryoosefi, Stefani Karp, Shankar Krishnan, Satyen Kale, Seungyeon Kim, Sanjiv Kumar
Fully Dynamic Submodular Maximization Over Matroids
Paul Duetting, Federico Fusco, Silvio Lattanzi, Ashkan Norouzi-Fard, Morteza Zadimoghaddam
GFlowNet-EM for Learning Compositional Latent Variable Models
Edward J Hu, Nikolay Malkin, Moksh Jain, Katie Everett, Alexandros Graikos, Yoshua Bengio
Improved Online Learning Algorithms for CTR Prediction in Ad Auctions
Zhe Feng, Christopher Liaw, Zixin Zhou
Large Language Models Struggle to Learn Long-Tail Knowledge
Nikhil Kandpal, Haikang Deng, Adam Roberts, Eric Wallace, Colin Raffel
Multi-channel Autobidding with Budget and ROI Constraints
Yuan Deng, Negin Golrezaei, Patrick Jaillet, Jason Cheuk Nam Liang, Vahab Mirrokni
Multi-layer Neural Networks as Trainable Ladders of Hilbert Spaces
Zhengdao Chen
On User-Level Private Convex Optimization
Badih Ghazi, Pritish Kamath, Ravi Kumar, Raghu Meka, Pasin Manurangsi, Chiyuan Zhang
PAC Generalization via Invariant Representations
Advait U Parulekar, Karthikeyan Shanmugam, Sanjay Shakkottai
Regularization and Variance-Weighted Regression Achieves Minimax Optimality in Linear MDPs: Theory and Practice
Toshinori Kitamura, Tadashi Kozuno, Yunhao Tang, Nino Vieillard, Michal Valko, Wenhao Yang, Jincheng Mei, Pierre Menard, Mohammad Gheshlaghi Azar, Remi Munos, Olivier Pietquin, Matthieu Geist,Csaba Szepesvari, Wataru Kumagai, Yutaka Matsuo
Speeding Up Bellman Ford via Minimum Violation Permutations
Silvio Lattanzi, Ola Svensson, Sergei Vassilvitskii
Statistical Indistinguishability of Learning Algorithms
Alkis Kalavasis, Amin Karbasi, Shay Moran, Grigoris Velegkas
Test-Time Adaptation with Slot-Centric Models
Mihir Prabhudesai, Anirudh Goyal, Sujoy Paul, Sjoerd van Steenkiste, Mehdi S. M. Sajjadi, Gaurav Aggarwal, Thomas Kipf, Deepak Pathak, Katerina Fragkiadaki>
Algorithms for Bounding Contribution for Histogram Estimation Under User-Level Privacy
Yuhan Liu*, Ananda Theertha Suresh, Wennan Zhu, Peter Kairouz, Marco Gruteser
Bandit Online Linear Optimization with Hints and Queries
Aditya Bhaskara, Ashok Cutkosky, Ravi Kumar, Manish Purohit
CLUTR: Curriculum Learning via Unsupervised Task Representation Learning
Abdus Salam Azad, Izzeddin Gur, Jasper Emhoff, Nathaniel Alexis, Aleksandra Faust, Pieter Abbeel, Ion Stoica
CSP: Self-Supervised Contrastive Spatial Pre-training for Geospatial-Visual Representations
Gengchen Mai, Ni Lao, Yutong He, Jiaming Song, Stefano Ermon
Ewald-Based Long-Range Message Passing for Molecular Graphs
Arthur Kosmala, Johannes Gasteiger, Nicholas Gao, Stephan Günnemann
Fast (1+ε)-Approximation Algorithms for Binary Matrix Factorization
Ameya Velingker, Maximilian Vötsch, David Woodruff, Samson Zhou
Federated Linear Contextual Bandits with User-Level Differential Privacy
Ruiquan Huang, Huanyu Zhang, Luca Melis, Milan Shen, Meisam Hejazinia, Jing Yang
Investigating the Role of Model-Based Learning in Exploration and Transfer
Jacob C Walker, Eszter Vértes, Yazhe Li, Gabriel Dulac-Arnold, Ankesh Anand, Theophane Weber, Jessica B Hamrick
Label Differential Privacy and Private Training Data Release
Robert Busa-Fekete, Andres Munoz, Umar Syed, Sergei Vassilvitskii
Lifelong Language Pretraining with Distribution-Specialized Experts
Wuyang Chen*, Yanqi Zhou, Nan Du, Yanping Huang, James Laudon, Zhifeng Chen, Claire Cui
Multi-User Reinforcement Learning with Low Rank Rewards
Dheeraj Mysore Nagaraj, Suhas S Kowshik, Naman Agarwal, Praneeth Netrapalli, Prateek Jain
Multi-View Masked World Models for Visual Robotic Manipulation
Younggyo Seo, Junsu Kim, Stephen James, Kimin Lee, Jinwoo Shin, Pieter Abbeel
PaLM-E: An Embodied Multimodal Language Model (see blog post)
Danny Driess, Fei Xia, Mehdi S. M. Sajjadi, Corey Lynch, Aakanksha Chowdhery, Brian Ichter,Ayzaan Wahid, Jonathan Tompson, Quan Vuong, Tianhe Yu, Wenlong Huang, Yevgen Chebotar, Pierre Sermanet, Daniel Duckworth, Sergey Levine, Vincent Vanhoucke, Karol Hausman, Marc Toussaint, Klaus Greff, Andy Zeng, Igor Mordatch, Pete Florence
Private Federated Learning with Autotuned Compression
Enayat Ullah*, Christopher A. Choquette-Choo, Peter Kairouz, Sewoong Oh
Refined Regret for Adversarial MDPs with Linear Function Approximation
Yan Dai, Haipeng Luo, Chen-Yu Wei, Julian Zimmert
Scaling Up Dataset Distillation to ImageNet-1K with Constant Memory
Justin Cui, Ruoche Wan, Si Si, Cho-Jui Hsieh
SGD with AdaGrad Stepsizes: Full Adaptivity with High Probability to Unknown Parameters, Unbounded Gradients and Affine Variance
Amit Attia, Tomer Koren
The Statistical Benefits of Quantile Temporal-Difference Learning for Value Estimation
Mark Rowland, Yunhao Tang, Clare Lyle, Rémi Munos, Marc G. Bellemare, Will Dabney
Unveiling The Mask of Position-Information Pattern Through the Mist of Image Features
Chieh Hubert Lin, Hung-Yu Tseng, Hsin-Ying Lee, Maneesh Kumar Singh, Ming-Hsuan Yang
User-Level Private Stochastic Convex Optimization with Optimal Rates
Raef Bassily, Ziteng Sun
A Simple Zero-Shot Prompt Weighting Technique to Improve Prompt Ensembling in Text-Image Models
James Urquhart Allingham*, Jie Ren, Michael W Dusenberry, Xiuye Gu, Yin Cui, Dustin Tran, Jeremiah Zhe Liu, Balaji Lakshminarayanan
Can Large Language Models Reason About Program Invariants?
Kexin Pei, David Bieber, Kensen Shi, Charles Sutton, Pengcheng Yin
Concurrent Shuffle Differential Privacy Under Continual Observation
Jay Tenenbaum, Haim Kaplan, Yishay Mansour, Uri Stemmer
Constant Matters: Fine-Grained Error Bound on Differentially Private Continual Observation
Hendrik Fichtenberger, Monika Henzinger, Jalaj Upadhyay
Cross-Entropy Loss Functions: Theoretical Analysis and Applications
Anqi Mao, Mehryar Mohri, Yutao Zhong
Efficient Rate Optimal Regret for Adversarial Contextual MDPs Using Online Function Approximation
Orin Levy, Alon Cohen, Asaf Cassel, Yishay Mansour
Fairness in Streaming Submodular Maximization Over a Matroid Constraint
Marwa El Halabi, Federico Fusco, Ashkan Norouzi-Fard, Jakab Tardos, Jakub Tarnawski
The Flan Collection: Designing Data and Methods for Effective Instruction Tuning (see blog post)
Shayne Longpre, Le Hou, Tu Vu, Albert Webson, Hyung Won Chung, Yi Tay, Denny Zhou, Quoc V Le, Barret Zoph, Jason Wei, Adam Roberts
Graph Reinforcement Learning for Network Control via Bi-level Optimization
Daniele Gammelli, James Harrison, Kaidi Yang, Marco Pavone, Filipe Rodrigues, Francisco C. Pereira
Learning-Augmented Private Algorithms for Multiple Quantile Release
Mikhail Khodak*, Kareem Amin, Travis Dick, Sergei Vassilvitskii
LegendreTron: Uprising Proper Multiclass Loss Learning
Kevin H Lam, Christian Walder, Spiridon Penev, Richard Nock
Measuring the Impact of Programming Language Distribution
Gabriel Orlanski*, Kefan Xiao, Xavier Garcia, Jeffrey Hui, Joshua Howland, Jonathan Malmaud, Jacob Austin, Rishabh Singh, Michele Catasta*
Multi-task Differential Privacy Under Distribution Skew
Walid Krichene, Prateek Jain, Shuang Song, Mukund Sundararajan, Abhradeep Thakurta, Li Zhang
Muse: Text-to-Image Generation via Masked Generative Transformers
Huiwen Chang, Han Zhang, Jarred Barber, AJ Maschinot, José Lezama, Lu Jiang, Ming-Hsuan Yang, Kevin Murphy, William T. Freeman, Michael Rubinstein, Yuanzhen Li, Dilip Krishnan
On the Convergence of Federated Averaging with Cyclic Client Participation
Yae Jee Cho, Pranay Sharma, Gauri Joshi, Zheng Xu, Satyen Kale, Tong Zhang
Optimal Stochastic Non-smooth Non-convex Optimization Through Online-to-Non-convex Conversion
Ashok Cutkosky, Harsh Mehta, Francesco Orabona
Out-of-Domain Robustness via Targeted Augmentations
Irena Gao, Shiori Sagawa, Pang Wei Koh, Tatsunori Hashimoto, Percy Liang
Polynomial Time and Private Learning of Unbounded Gaussian Mixture Models
Jamil Arbas, Hassan Ashtiani, Christopher Liaw
Pre-computed Memory or On-the-Fly Encoding? A Hybrid Approach to Retrieval Augmentation Makes the Most of Your Compute
Michiel de Jong, Yury Zemlyanskiy, Nicholas FitzGerald, Joshua Ainslie, Sumit Sanghai, Fei Sha, William W. Cohen
Scalable Adaptive Computation for Iterative Generation
Allan Jabri*, David J. Fleet, Ting Chen
Scaling Spherical CNNs
Carlos Esteves, Jean-Jacques Slotine, Ameesh Makadia
STEP: Learning N:M Structured Sparsity Masks from Scratch with Precondition
Yucheng Lu, Shivani Agrawal, Suvinay Subramanian, Oleg Rybakov, Christopher De Sa, Amir Yazdanbakhsh
Stratified Adversarial Robustness with Rejection
Jiefeng Chen, Jayaram Raghuram, Jihye Choi, Xi Wu, Yingyu Liang, Somesh Jha
When Does Privileged information Explain Away Label Noise?
Guillermo Ortiz-Jimenez*, Mark Collier, Anant Nawalgaria, Alexander D’Amour, Jesse Berent, Rodolphe Jenatton, Effrosyni Kokiopoulou
Adaptive Computation with Elastic Input Sequence
Fuzhao Xue*, Valerii Likhosherstov, Anurag Arnab, Neil Houlsby, Mostafa Dehghani, Yang You
Can Neural Network Memorization Be Localized?
Pratyush Maini, Michael C. Mozer, Hanie Sedghi, Zachary C. Lipton, J. Zico Kolter, Chiyuan Zhang
Controllability-Aware Unsupervised Skill Discovery
Seohong Park, Kimin Lee, Youngwoon Lee, Pieter Abbeel
Efficient Learning of Mesh-Based Physical Simulation with Bi-Stride Multi-Scale Graph Neural Network
Yadi Cao, Menglei Chai, Minchen Li, Chenfanfu Jiang
Federated Heavy Hitter Recovery Under Linear Sketching
Adria Gascon, Peter Kairouz, Ziteng Sun, Ananda Theertha Suresh
Graph Generative Model for Benchmarking Graph Neural Networks
Minji Yoon, Yue Wu, John Palowitch, Bryan Perozzi, Russ Salakhutdinov
H-Consistency Bounds for Pairwise Misranking Loss Surrogates
Anqi Mao, Mehryar Mohri, Yutao Zhong
Improved Regret for Efficient Online Reinforcement Learning with Linear Function Approximation
Uri Sherman, Tomer Koren, Yishay Mansour
Invariant Slot Attention: Object Discovery with Slot-Centric Reference Frames
Ondrej Biza*, Sjoerd van Steenkiste, Mehdi S. M. Sajjadi, Gamaleldin Fathy Elsayed, Aravindh Mahendran, Thomas Kipf
Multi-task Off-Policy Learning from Bandit Feedback
Joey Hong, Branislav Kveton, Manzil Zaheer, Sumeet Katariya, Mohammad Ghavamzadeh
Optimal No-Regret Learning for One-Sided Lipschitz Functions
Paul Duetting, Guru Guruganesh, Jon Schneider, Joshua Ruizhi Wang
Policy Mirror Ascent for Efficient and Independent Learning in Mean Field Games
Batuhan Yardim, Semih Cayci, Matthieu Geist, Niao He
Regret Minimization and Convergence to Equilibria in General-Sum Markov Games
Liad Erez, Tal Lancewicki, Uri Sherman, Tomer Koren, Yishay Mansour
Reinforcement Learning Can Be More Efficient with Multiple Rewards
Christoph Dann, Yishay Mansour, Mehryar Mohri
Reinforcement Learning with History-Dependent Dynamic Contexts
Guy Tennenholtz, Nadav Merlis, Lior Shani, Martin Mladenov, Craig Boutlier
User-Defined Event Sampling and Uncertainty Quantification in Diffusion Models for Physical Dynamical Systems
Marc Anton Finzi*, Anudhyan Boral, Andrew Gordon Wilson, Fei Sha, Leonardo Zepeda-Nunez
Discrete Key-Value Bottleneck
Frederik Träuble, Anirudh Goyal, Nasim Rahaman, Michael Curtis Mozer, Kenji Kawaguchi, Yoshua Bengio, Bernhard Schölkopf
DSGD-CECA: Decentralized SGD with Communication-Optimal Exact Consensus Algorithm
Lisang Ding, Kexin Jin, Bicheng Ying, Kun Yuan, Wotao Yin
Exphormer: Sparse Transformers for Graphs
Hamed Shirzad, Ameya Velingker, Balaji Venkatachalam, Danica J. Sutherland, Ali Kemal Sinop
Fast, Differentiable and Sparse Top-k: A Convex Analysis Perspective
Michael Eli Sander*, Joan Puigcerver, Josip Djolonga, Gabriel Peyré, Mathieu Blondel
Improved Policy Evaluation for Randomized Trials of Algorithmic Resource Allocation
Aditya Mate, Bryan Wilder, Aparna Taneja, Milind Tambe
In Search for a Generalizable Method for Source Free Domain Adaptation
Malik Boudiaf*, Tom Denton, Bart van Merrienboer, Vincent Dumoulin, Eleni Triantafillou
Learning Rate Schedules in the Presence of Distribution Shift
Matthew Fahrbach, Adel Javanmard, Vahab Mirrokni, Pratik Worah
Not All Semantics Are Created Equal: Contrastive Self-Supervised Learning with Automatic Temperature Individualization
Zi-Hao Qiu, Quanqi Hu, Zhuoning Yuan, Denny Zhou, Lijun Zhang, Tianbao Yang
On the Relationship Between Explanation and Prediction: A Causal View
Amir-Hossein Karimi*, Krikamol Muandet, Simon Kornblith, Bernhard Schölkopf, Been Kim
On the Role of Attention in Prompt-Tuning
Samet Oymak, Ankit Singh Rawat, Mahdi Soltanolkotabi, Christos Thrampoulidis
PLay: Parametrically Conditioned Layout Generation Using Latent Diffusion
Chin-Yi Cheng, Forrest Huang, Gang Li, Yang Li
The Power of Learned Locally Linear Models for Nonlinear Policy Optimization
Daniel Pfrommer, Max Simchowitz, Tyler Westenbroek, Nikolai Matni, Stephen Tu
Relevant Walk Search for Explaining Graph Neural Networks
Ping Xiong, Thomas Schnake, Michael Gastegger, Grégoire Montavon, Klaus Robert Muller,Shinichi Nakajima
Repository-Level Prompt Generation for Large Language Models of Code
Disha Shrivastava, Hugo Larochelle, Daniel Tarlow
Robust and Private Stochastic Linear Bandits
Vasileios Charisopoulos*, Hossein Esfandiari, Vahab Mirrokni
Simple Diffusion: End-to-End Diffusion for High Resolution Images
Emiel Hoogeboom, Jonathan Heek, Tim Salimans
Tied-Augment: Controlling Representation Similarity Improves Data Augmentation
Emirhan Kurtulus, Zichao Li, Yann Dauphin, Ekin D. Cubuk
Why Is Public Pre-Training Necessary for Private Model Training?
Arun Ganesh, Mahdi Haghifam*, Milad Nasr, Sewoong Oh, Thomas Steinke, Om Thakkar, Abhradeep Guha Thakurta, Lun Wang
A Connection Between One-Step RL and Critic Regularization in Reinforcement Learning
Benjamin Eysenbach, Matthieu Geist, Sergey Levine, Ruslan Salakhutdinov
Beyond Uniform Lipschitz Condition in Differentially Private Optimization
Rudrajit Das*, Satyen Kale, Zheng Xu, Tong Zhang, Sujay Sanghavi
Efficient Graph Field Integrators Meet Point Clouds
Krzysztof Choromanski, Arijit Sehanobish, Han Lin, Yunfan Zhao, Eli Berger, Tetiana Parshakova, Alvin Pan, David Watkins, Tianyi Zhang, Valerii Likhosherstov, Somnath Basu Roy Chowdhury, Avinava Dubey, Deepali Jain, Tamas Sarlos, Snigdha Chaturvedi, Adrian Weller
Fast as CHITA: Neural Network Pruning with Combinatorial Optimization
Riade Benbaki, Wenyu Chen, Xiang Meng, Hussein Hazimeh, Natalia Ponomareva, Zhe Zhao, Rahul Mazumder
Jump-Start Reinforcement Learning (see blog post)
Ikechukwu Uchendu*, Ted Xiao, Yao Lu, Banghua Zhu, Mengyuan Yan, Joséphine Simon, Matthew Bennice, Chuyuan Fu, Cong Ma, Jiantao Jiao, Sergey Levine, Karol Hausman
Learning in POMDPs is Sample-Efficient with Hindsight Observability
Jonathan Lee, Alekh Agarwal, Christoph Dann, Tong Zhang
Low-Variance Gradient Estimation in Unrolled Computation Graphs with ES-Single
Paul Vicol
Masked Trajectory Models for Prediction, Representation, and Control
Philipp Wu, Arjun Majumdar, Kevin Stone, Yixin Lin, Igor Mordatch, Pieter Abbeel, Aravind Rajeswaran
Overcoming Simplicity Bias in Deep Networks Using a Feature Sieve
Rishabh Tiwari, Pradeep Shenoy
Pairwise Ranking Losses of Click-Through Rates Prediction for Welfare Maximization in Ad Auctions
Boxiang Lyu, Zhe Feng, Zachary Robertson, Sanmi Koyejo
Predictive Flows for Faster Ford-Fulkerson
Sami Davies, Benjamin Moseley, Sergei Vassilvitskii, Yuyan Wang
Scaling Laws for Multilingual Neural Machine Translation
Patrick Fernandes, Behrooz Ghorbani, Xavier Garcia, Markus Freitag, Orhan Firat
Sequential Monte Carlo Learning for Time Series Structure Discovery
Feras Saad, Brian Patton, Matthew Douglas Hoffman, Rif A. Saurous, Vikash Mansinghka
Stochastic Gradient Succeeds for Bandits
Jincheng Mei, Zixin Zhong, Bo Dai, Alekh Agarwal, Csaba Szepesvari, Dale Schuurmans
Subset-Based Instance Optimality in Private Estimation
Travis Dick, Alex Kulesza, Ziteng Sun, Ananda Theertha Suresh
The Unreasonable Effectiveness of Few-Shot Learning for Machine Translation
Xavier Garcia, Yamini Bansal, Colin Cherry, George Foster, Maxim Krikun, Melvin Johnson, Orhan Firat
Tutorials
Self-Supervised Learning in Vision: from Research Advances to Best Practices
Xinlei Chen, Ishan Misra, Randall Balestriero, Mathilde Caron, Christoph Feichtenhofer, Mark Ibrahim
How to DP-fy ML: A Practical Tutorial to Machine Learning with Differential Privacy (see blog post)
Sergei Vassilvitskii, Natalia Ponomareva, Zheng Xu
Recent Advances in the Generalization Theory of Neural Networks
Tengyu Ma, Alex Damian
EXPO Day workshops
Graph Neural Networks in Tensorflow: A Practical Guide
Workshop Organizers include: Bryan Perozzi, Anton Tsitsulin, Brandon Mayer, Jonathan Halcrow
Google sponsored affinity workshops
LatinX in AI (LAXAI)
Platinum Sponsor
Keynote Speaker: Monica Ribero
Panelist: Yao Qin
Women in Machine Learning (WiML)
Platinum Sponsor
Panelists: Yao Qin
Workshops
Federated Learning and Analytics in Practice: Algorithms, Systems, Applications, and Opportunities
Organizer: Peter Kairouz, Zheng Xu
Speaker: Brendan McMahan
Interpretable Machine Learning in Healthcare (IMLH)
Organizer: Ramin Zabih
Knowledge and Logical Reasoning in the Era of Data-Driven Learning
Organizer: Beliz Günel
The Many Facets of Preference-Based Learning (MFPL)
Organizer: Robert Busa-Fekete, Mohammad Ghavamzadeh
The Synergy of Scientific and Machine Learning Modelling (SynS & ML)
Speaker: Sercan Arik
Theory of Mind in Communicating Agents
Organizer: Pei Zhou
Artificial Intelligence & Human Computer Interaction
Organizer: Yang Li, Forrest Huang
Data-Centric Machine Learning Research (DMLR)
Organizer: Alicia Parrish, Najoung Kim
Speaker: Peter Mattson
Neural Compression: from Information Theory to Applications
Speaker: Johannes Ballé
Panelist: George Toderici
Organizer: Ahmad Beirami
Spurious Correlations, Invariance and Stability (SCIS)
Organizer: Amir Feder
* Work done while at Google
AI-related products and technologies are constructed and deployed in a societal context: that is, a dynamic and complex collection of social, cultural, historical, political and economic circumstances. Because societal contexts by nature are dynamic, complex, non-linear, contested, subjective, and highly qualitative, they are challenging to translate into the quantitative representations, methods, and practices that dominate standard machine learning (ML) approaches and responsible AI product development practices.
The first phase of AI product development is problem understanding, and this phase has tremendous influence over how problems (e.g., increasing cancer screening availability and accuracy) are formulated for ML systems to solve as well many other downstream decisions, such as dataset and ML architecture choice. When the societal context in which a product will operate is not articulated well enough to result in robust problem understanding, the resulting ML solutions can be fragile and even propagate unfair biases.
When AI product developers lack access to the knowledge and tools necessary to effectively understand and consider societal context during development, they tend to abstract it away. This abstraction leaves them with a shallow, quantitative understanding of the problems they seek to solve, while product users and society stakeholders — who are proximate to these problems and embedded in related societal contexts — tend to have a deep qualitative understanding of those same problems. This qualitative–quantitative divergence in ways of understanding complex problems that separates product users and society from developers is what we call the problem understanding chasm.
This chasm has repercussions in the real world: for example, it was the root cause of racial bias discovered by a widely used healthcare algorithm intended to solve the problem of choosing patients with the most complex healthcare needs for special programs. Incomplete understanding of the societal context in which the algorithm would operate led system designers to form incorrect and oversimplified causal theories about what the key problem factors were. Critical socio-structural factors, including lack of access to healthcare, lack of trust in the health care system, and underdiagnosis due to human bias, were left out while spending on healthcare was highlighted as a predictor of complex health need.
To bridge the problem understanding chasm responsibly, AI product developers need tools that put community-validated and structured knowledge of societal context about complex societal problems at their fingertips — starting with problem understanding, but also throughout the product development lifecycle. To that end, Societal Context Understanding Tools and Solutions (SCOUTS) — part of the Responsible AI and Human-Centered Technology (RAI-HCT) team within Google Research — is a dedicated research team focused on the mission to “empower people with the scalable, trustworthy societal context knowledge required to realize responsible, robust AI and solve the world’s most complex societal problems.” SCOUTS is motivated by the significant challenge of articulating societal context, and it conducts innovative foundational and applied research to produce structured societal context knowledge and to integrate it into all phases of the AI-related product development lifecycle. Last year we announced that Jigsaw, Google’s incubator for building technology that explores solutions to threats to open societies, leveraged our structured societal context knowledge approach during the data preparation and evaluation phases of model development to scale bias mitigation for their widely used Perspective API toxicity classifier. Going forward SCOUTS’ research agenda focuses on the problem understanding phase of AI-related product development with the goal of bridging the problem understanding chasm.
Bridging the AI problem understanding chasm
Bridging the AI problem understanding chasm requires two key ingredients: 1) a reference frame for organizing structured societal context knowledge and 2) participatory, non-extractive methods to elicit community expertise about complex problems and represent it as structured knowledge. SCOUTS has published innovative research in both areas.
An illustration of the problem understanding chasm. |
A societal context reference frame
An essential ingredient for producing structured knowledge is a taxonomy for creating the structure to organize it. SCOUTS collaborated with other RAI-HCT teams (TasC, Impact Lab), Google DeepMind, and external system dynamics experts to develop a taxonomic reference frame for societal context. To contend with the complex, dynamic, and adaptive nature of societal context, we leverage complex adaptive systems (CAS) theory to propose a high-level taxonomic model for organizing societal context knowledge. The model pinpoints three key elements of societal context and the dynamic feedback loops that bind them together: agents, precepts, and artifacts.
- Agents: These can be individuals or institutions.
- Precepts: The preconceptions — including beliefs, values, stereotypes and biases — that constrain and drive the behavior of agents. An example of a basic precept is that “all basketball players are over 6 feet tall.” That limiting assumption can lead to failures in identifying basketball players of smaller stature.
- Artifacts: Agent behaviors produce many kinds of artifacts, including language, data, technologies, societal problems and products.
The relationships between these entities are dynamic and complex. Our work hypothesizes that precepts are the most critical element of societal context and we highlight the problems people perceive and the causal theories they hold about why those problems exist as particularly influential precepts that are core to understanding societal context. For example, in the case of racial bias in a medical algorithm described earlier, the causal theory precept held by designers was that complex health problems would cause healthcare expenditures to go up for all populations. That incorrect precept directly led to the choice of healthcare spending as the proxy variable for the model to predict complex healthcare need, which in turn led to the model being biased against Black patients who, due to societal factors such as lack of access to healthcare and underdiagnosis due to bias on average, do not always spend more on healthcare when they have complex healthcare needs. A key open question is how can we ethically and equitably elicit causal theories from the people and communities who are most proximate to problems of inequity and transform them into useful structured knowledge?
![]() |
Illustrative version of societal context reference frame. |
![]() |
Taxonomic version of societal context reference frame. |
Working with communities to foster the responsible application of AI to healthcare
Since its inception, SCOUTS has worked to build capacity in historically marginalized communities to articulate the broader societal context of the complex problems that matter to them using a practice called community based system dynamics (CBSD). System dynamics (SD) is a methodology for articulating causal theories about complex problems, both qualitatively as causal loop and stock and flow diagrams (CLDs and SFDs, respectively) and quantitatively as simulation models. The inherent support of visual qualitative tools, quantitative methods, and collaborative model building makes it an ideal ingredient for bridging the problem understanding chasm. CBSD is a community-based, participatory variant of SD specifically focused on building capacity within communities to collaboratively describe and model the problems they face as causal theories, directly without intermediaries. With CBSD we’ve witnessed community groups learn the basics and begin drawing CLDs within 2 hours.
![]() |
Data 4 Black Lives community members learning system dynamics. |
There is a huge potential for AI to improve medical diagnosis. But the safety, equity, and reliability of AI-related health diagnostic algorithms depends on diverse and balanced training datasets. An open challenge in the health diagnostic space is the dearth of training sample data from historically marginalized groups. SCOUTS collaborated with the Data 4 Black Lives community and CBSD experts to produce qualitative and quantitative causal theories for the data gap problem. The theories include critical factors that make up the broader societal context surrounding health diagnostics, including cultural memory of death and trust in medical care.
The figure below depicts the causal theory generated during the collaboration described above as a CLD. It hypothesizes that trust in medical care influences all parts of this complex system and is the key lever for increasing screening, which in turn generates data to overcome the data diversity gap.
![]() |
![]() |
Causal loop diagram of the health diagnostics data gap |
These community-sourced causal theories are a first step to bridge the problem understanding chasm with trustworthy societal context knowledge.
Conclusion
As discussed in this blog, the problem understanding chasm is a critical open challenge in responsible AI. SCOUTS conducts exploratory and applied research in collaboration with other teams within Google Research, external community, and academic partners across multiple disciplines to make meaningful progress solving it. Going forward our work will focus on three key elements, guided by our AI Principles:
- Increase awareness and understanding of the problem understanding chasm and its implications through talks, publications, and training.
- Conduct foundational and applied research for representing and integrating societal context knowledge into AI product development tools and workflows, from conception to monitoring, evaluation and adaptation.
- Apply community-based causal modeling methods to the AI health equity domain to realize impact and build society’s and Google’s capability to produce and leverage global-scale societal context knowledge to realize responsible AI.
![]() |
SCOUTS flywheel for bridging the problem understanding chasm. |
Acknowledgments
Thank you to John Guilyard for graphics development, everyone in SCOUTS, and all of our collaborators and sponsors.
Modeling time series data can be challenging (and fascinating) due to its inherent complexity and unpredictability. Long-term trends in time series can change…
Modeling time series data can be challenging (and fascinating) due to its inherent complexity and unpredictability. Long-term trends in time series can change drastically due to certain events, for example. Recall the beginning of the global pandemic, when businesses such as airlines or brick-and-mortar shops saw a quick decline in the number of customers and sales. In contrast, e-commerce businesses continued to operate with less disruption.
Interaction terms can help model such patterns. They capture complex relationships between variables and, as a result, lead to more accurate predictions.
This post explores:
- Interaction terms in the context of time series forecasting
- Benefits of interaction terms when modeling complex relationships
- How to effectively implement interaction terms in your models
Overview of interaction terms
Interaction terms enable you to investigate whether the relationship between the target and a feature changes depending on the value of another feature. For more details, see my previous post, A Comprehensive Guide to Interaction Terms in Linear Regression.
Figure 1 shows a scatterplot that represents the relationship between miles per gallon (target) and the weight of a vehicle (feature). The relationship is quite different depending on the transmission type (another feature).
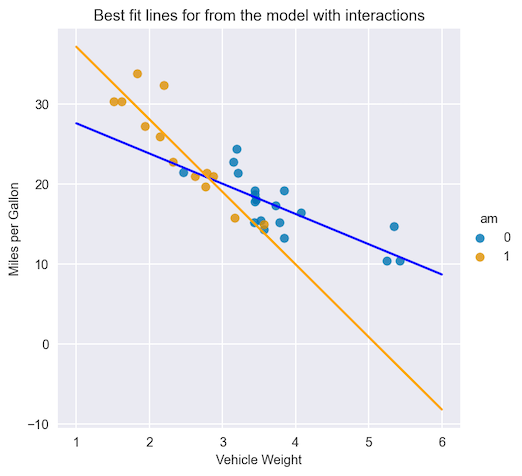
Improving linear model accuracy
Without using interaction terms, a linear model would not be able to capture such a complex relationship. Effectively, it would assign the same coefficient for the weight feature, regardless of the type of transmission. Figure 1 shows the coefficients (slope of the line) by weight feature, which are drastically different for different transmission types.
To overcome this fallacy and make the linear model more flexible, add interaction terms. In general, they are a multiplication of the original features. By adding these new variables to the regression model, you can measure the effects of the interaction between them and the target.
Interaction terms in time series forecasting
Interaction terms make linear models more flexible. The following example shows how they work in the context of time series forecasting.
Prerequisites
First, load the required libraries:
import numpy as np
import pandas as pd
from sklearn.linear_model import LinearRegression
import seaborn as sns
import matplotlib.pyplot as plt
Dataset generation
Then, generate some artificial time series data with the following characteristics:
- 10 years of daily data
- Repeating patterns (seasonality) present in the time series
- A decreasing trend over the first 7 years
- No trend in the last 3 years
- Random noise, added as the last step
# for reproducibility
np.random.seed(42)
# generate the DataFrame with dates
range_of_dates = pd.date_range(
start="2010-01-01",
end="2019-12-30"
)
df = pd.DataFrame(index=range_of_dates)
# create a sequence of day numbers
df["linear_trend"] = range(len(df))
df["trend"] = 0.004 * df["linear_trend"].values[::-1]
df.loc["2017-01-01":, "trend"] = 4
# generate the components of the target
signal_1 = 10 + 4 * np.sin(df["linear_trend"] / 365 * 2 * np.pi)
noise = np.random.normal(0, 0.85, len(df))
# combine them to get the target series
df["target"] = signal_1 + noise + df["trend"]
# plot
df["target"].plot(title="Generated time series");
Figure 2 shows the generated time series, which includes all the desired characteristics.
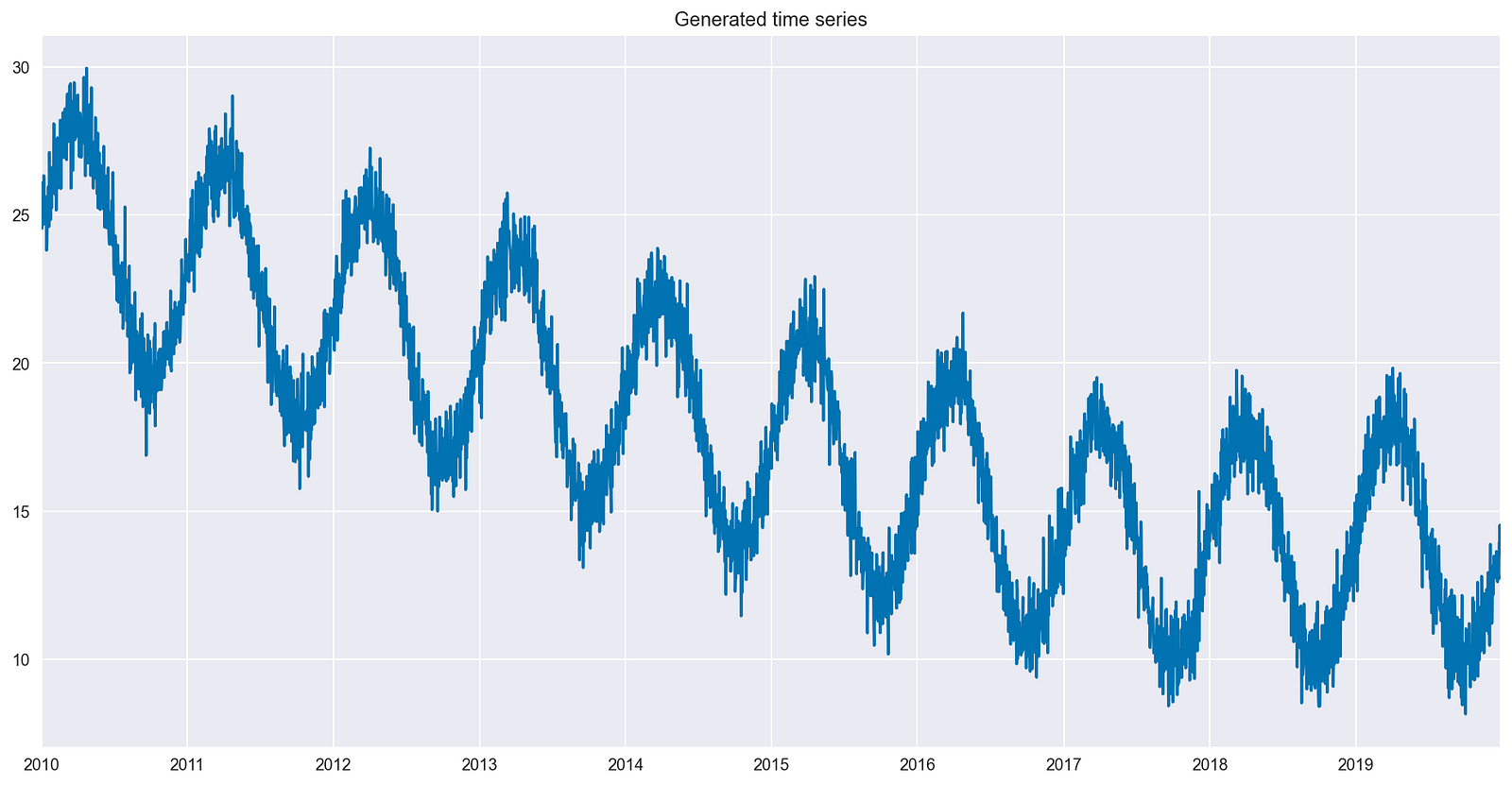
Training the benchmark model
Now train a linear model and inspect the best fit line. For this step, create very simple models with a few features. This enables you to visually inspect the impact of the interaction term on the model’s fit.
The simplest model possible contains one feature — an indicator of the passage of time. The linear_trend
column created for the time series is effectively the row number of the DataFrame (ordered by date).
X = df[["linear_trend"]]
y = df[["target"]]
lm = LinearRegression()
lm.fit(X, y)
df["model_1"] = lm.predict(X)
df[["target", "model_1"]].plot(title="Linear trend");
It is worth mentioning that the point is not to properly evaluate the forecasts using separate train and test sets, but rather to explain the impact of the interaction terms on the model’s fit. It is easier to observe the interaction term’s impact by inspecting the fitted values (prediction on the training set) and comparing those fitted values to the original time series.
Figure 3 shows that the linear model identified a decreasing trend for the entire time series. At the same time, the fit seems off for the last 3 years of data, as there is no trend there.
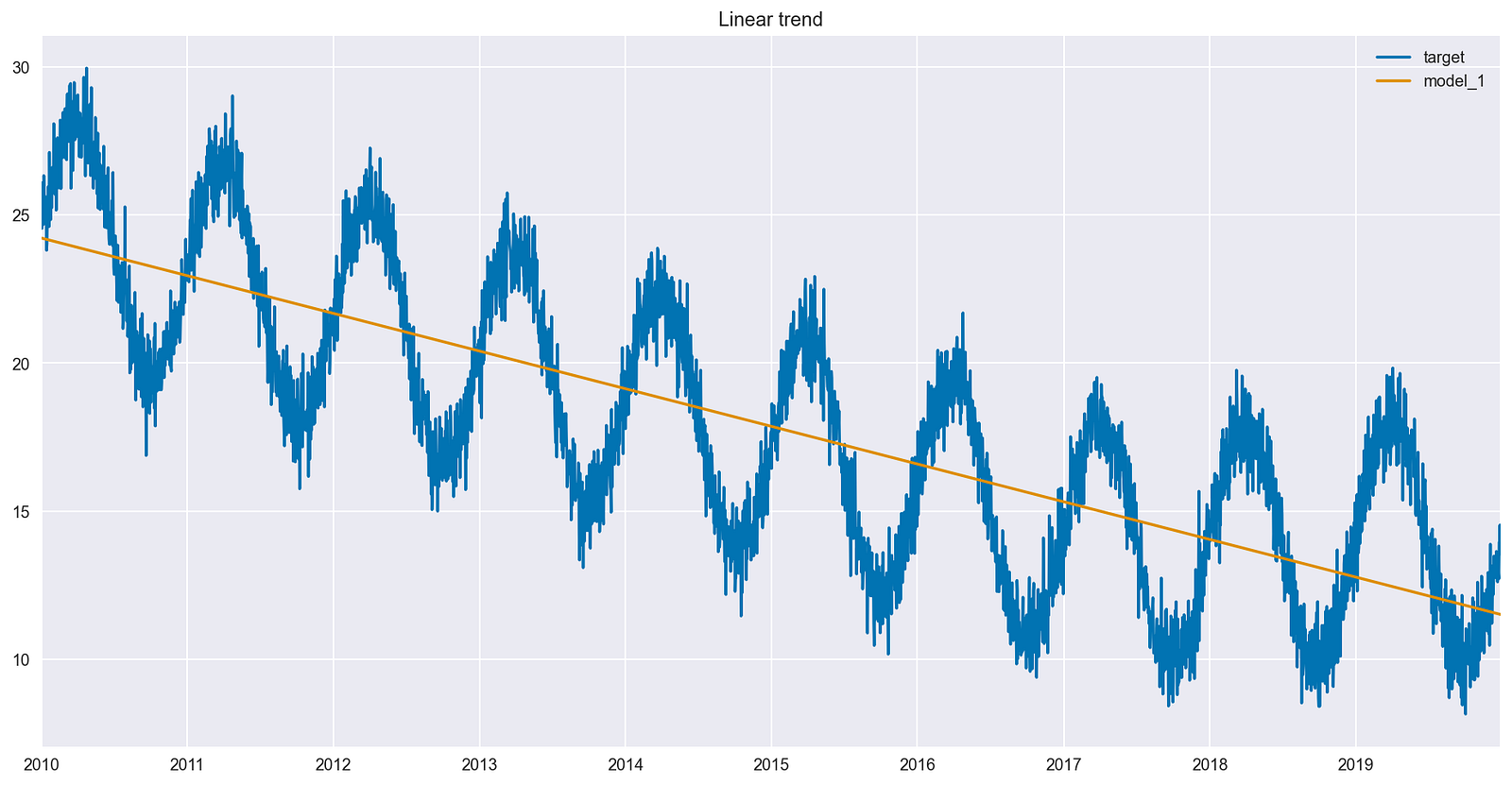
Add a breakpoint
Next, try to make the model learn the new pattern (trend change) using feature engineering. To do so, create a breakpoint, which is a placeholder variable indicating whether a given observation is after January 1, 2017. In this case, the exact point in time when the trend change happened is known.
Next, train another linear model, this time with two features:
df["after_2017_breakpoint"] = np.where(df.index >= pd.Timestamp('2017-01-01'), 1, 0)
X = df[["linear_trend", "after_2017_breakpoint"]]
y = df[["target"]]
lm = LinearRegression()
lm.fit(X, y)
df["model_2"] = lm.predict(X)
df[["target", "model_2"]].plot(title="Linear trend + breakpoint");
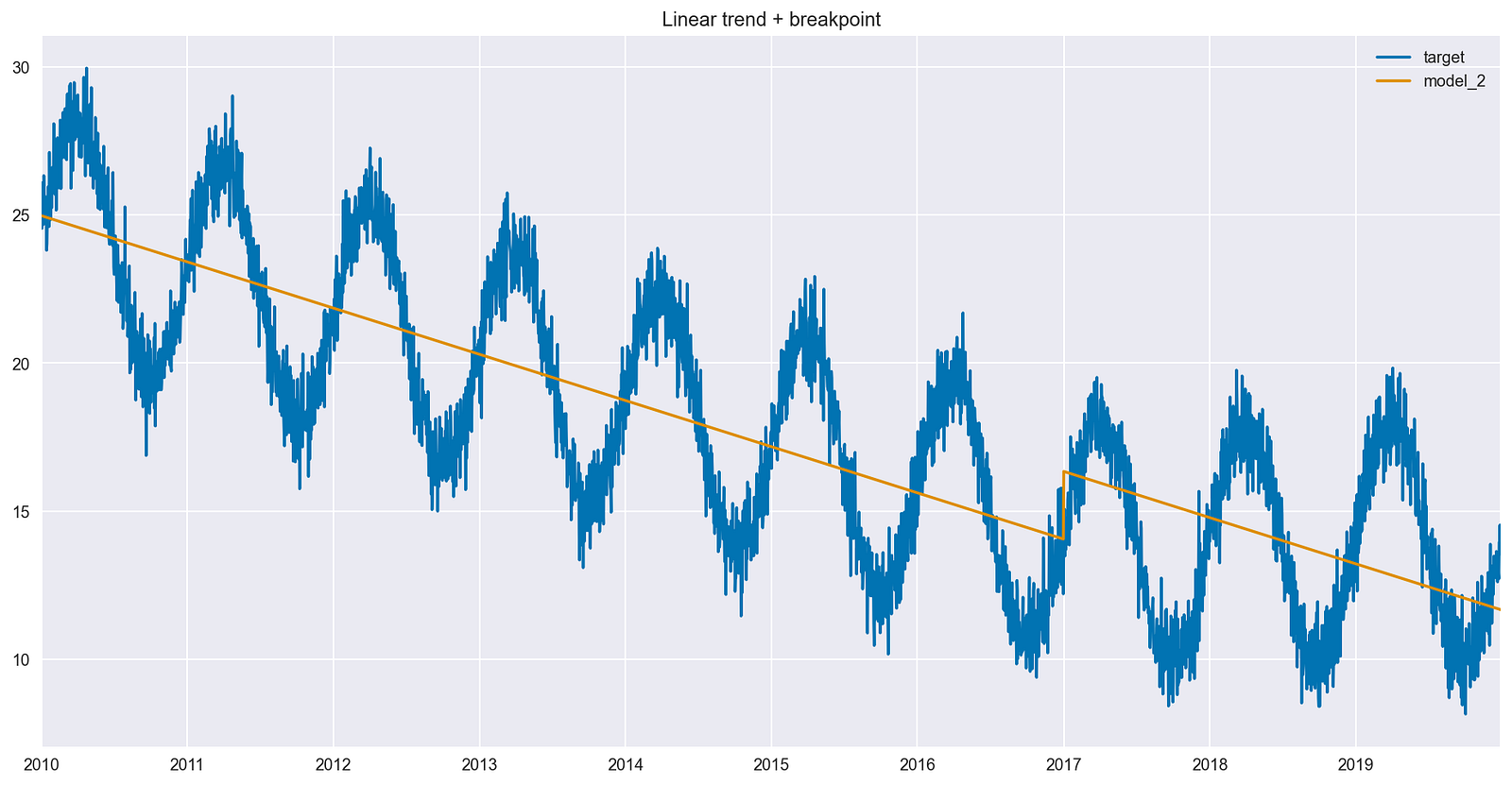
Figure 4 shows a few important changes, as listed below:
- The fitted line displays a vertical jump, which corresponds to the coefficient by the new Boolean feature.
- The vertical jump occurs exactly on the first date when the feature becomes active (a value of 1 instead of 0).
- The slope of the line is the same before and after the introduced breakpoint.
- The model is trying to compensate for the incorrect slope by adding a fixed amount to the predictions after the breakpoint.
There is no trend in the last 3 years of data, so ideally the line should be close to flat after January 1, 2017.
Adding an interaction term
To change the slope after the breakpoint, add a more complex dependency on the timestamp (represented by a linear trend). That is exactly what an interaction term does–it is a multiplication of the linear trend and the placeholder variable.
df["interaction_term"] = df["after_2017_breakpoint"] * df["linear_trend"]
X = df[["linear_trend", "after_2017_breakpoint", "interaction_term"]]
y = df[["target"]]
lm = LinearRegression()
lm.fit(X, y)
df["model_3"] = lm.predict(X)
df[["target", "model_3"]].plot(title="Linear trend + breakpoint + interaction term");
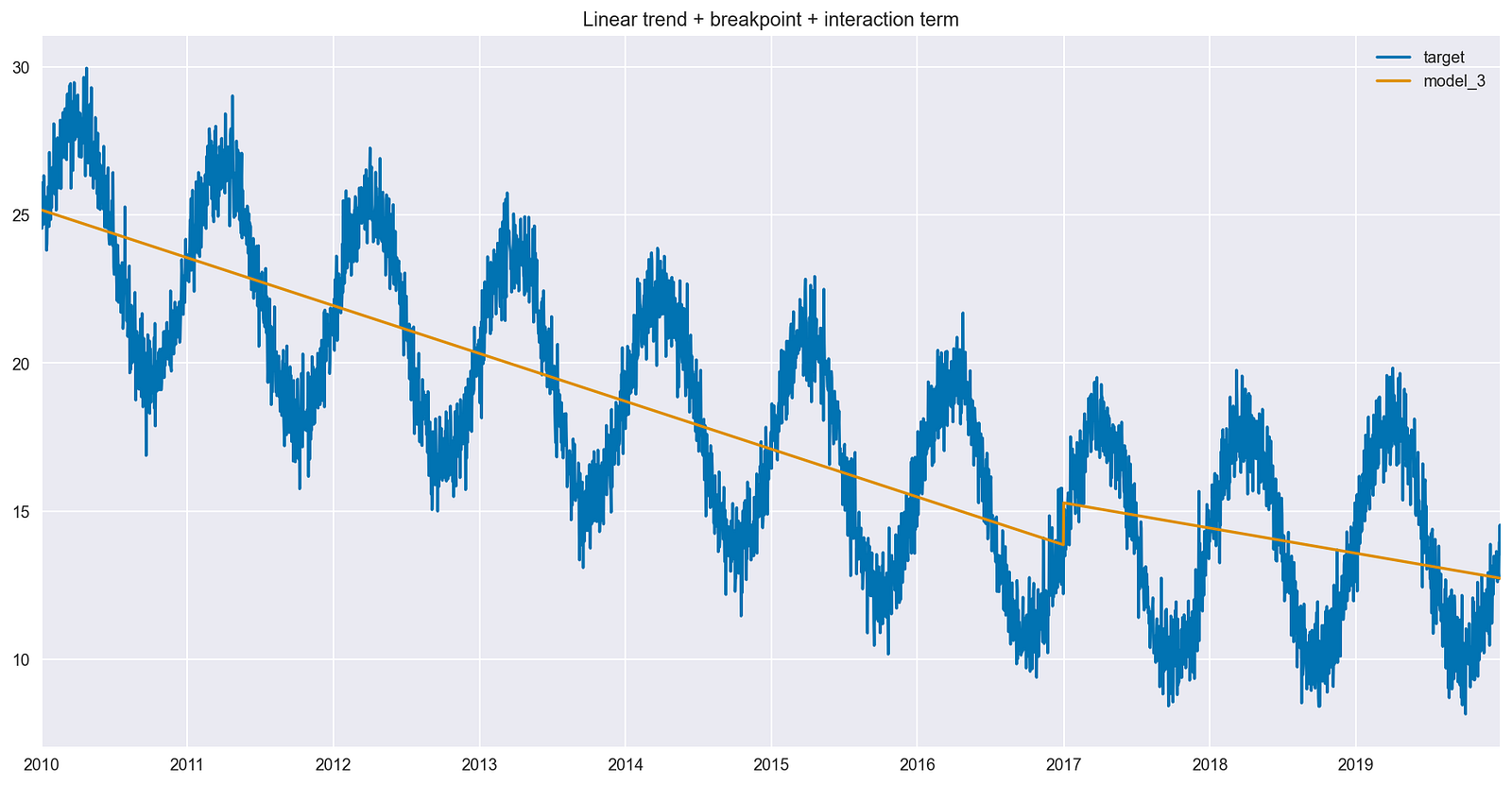
Figure 5 shows the impact of having the interaction term in the model. Compared to Figure 4, the slope of the best fit line is different after the breakpoint.
To be more precise, the difference is actually the value of the coefficient by the interaction term. While the new line did not flatten out, it is still less steep than it used to be in the earlier parts of the time series.
Introducing the breakpoint together with an interaction term increased the model’s ability to capture the trend of the time series. In turn, that should increase the predictive performance of the model.
Summary
Using interaction terms can make the specification of a linear model more flexible (different slopes for different lines), which can result in a better fit to the data and better predictive performance. You can add interaction terms as a multiplication of the original features. In the context of time series, you can use interaction terms to better capture any changes to the trend.
Find the code used in this post in A Comprehensive Guide on Interaction Terms in Time Series Forecasting on GitHub. Additionally, the code in the notebook shows how to leverage cuDF and cuML to train your models using GPU acceleration. As always, feedback is welcome. You can reach out to me on Twitter or in the comments.
It’s a party this GFN Thursday with several newly launched titles streaming on GeForce NOW. Revel in gaming goodness with Xenonauts 2, Viewfinder and Techtonica, among the four new games joining the cloud this week. Portal fans, stay tuned — the Portal: Prelude RTX mod will be streaming on GeForce NOW to members soon. Plus, Read article >
Oracle is one of the top cloud service providers in the world, supporting over 22,000 customers and reporting revenue of nearly $4 billion per quarter and…
Oracle is one of the top cloud service providers in the world, supporting over 22,000 customers and reporting revenue of nearly $4 billion per quarter and annual growth of greater than 40%. Oracle Cloud Infrastructure (OCI) is growing at an even faster rate and offers a complete cloud infrastructure for every workload.
Having added 11 regions in the last 18 months, OCI currently offers 41 regions and supports hosted, on-premises, hybrid, and multi-cloud deployments. It enables customers to run a mix of custom-built, third-party ISVs and Oracle applications on a scalable architecture. OCI provides scalable networking and tools to support security, observability, compliance, and cost management.
One of the differentiators of OCI is its ability to offer high-performance computing (HPC), Oracle Exadata and Autonomous Database, and GPU-powered applications such as AI and machine learning (ML), with fast infrastructure-as-a-service (IaaS) performance that rivals dedicated on-premises infrastructure. A key component to delivering this high performance is a scalable, low-latency network that supports remote direct memory access (RDMA). For more details, see First Principles: Building a High-Performance Network in the Public Cloud.
Networking challenge of HPC and GPU-powered compute
A commonality across HPC applications, GPU-powered AI workloads, and the Oracle Autonomous Database on Exadata is that they all run as distributed workloads. Data processing occurs simultaneously on multiple nodes, using a few dozen to thousands of CPUs and GPUs. These nodes must communicate with each other, share intermediate results in multi-stage problem solving with gigabytes to petabytes of storage to access common data, and often assemble the results of distributed computing into a cohesive solution.
These applications require high throughput and low latency to communicate across nodes to solve problems quickly. Amdahl’s law states that the speedup from parallelizing a task is limited by how much of the task is inherently serial and cannot be parallelized. The amount of time needed to transfer information between nodes adds inherently serial time to the task because nodes must wait for the data transfer to complete and for the slowest node in the task to finish before starting the next parallelizable part of the job.
For this reason, the performance of the cluster network becomes paramount, and an optimized network can enable a distributed compute cluster to deliver results much sooner than the same computing resources running on a slower network. This time-saving speeds job completion and reduces costs.
What is RDMA?
RDMA is remote direct memory access, the most efficient means of transferring data between different machines. It enables a server or storage appliance to communicate and share data over a network without making extra copies and without interrupting the host CPU. It is used for AI, big data, and other distributed technical computing workloads.
Traditional networking interrupts the CPU multiple times and makes multiple copies of the data being transmitted as it passes from the application through the OS kernel, to the adapter, then back up the stack on the receiving end. RDMA uses only one copy of the data on each end and typically bypasses the kernel, placing data directly in the receiving machine’s memory without interrupting the CPU.
This process enables lower latency and higher throughput on the network and lower CPU utilization for the servers and storage systems. Today, the majority of HPC, technical computing, and AI applications can be accelerated by RDMA. For more details, see How RDMA Became the Fuel for Fast Networks.
What is InfiniBand?
InfiniBand is a lossless network optimized for HPC, AI, big data, and other distributed technical computing workloads. It typically supports the highest bandwidth available (currently 400 Gbps per connection) for data center networks and RDMA, enabling machines to communicate and share data without interrupting the host CPU.
InfiniBand adapters offload networking and data movement tasks from the CPU and feature an optimized, efficient networking stack, enabling CPUs, GPUs, and storage to move data rapidly and efficiently. The InfiniBand adapters and switches can also perform specific compute and data aggregation tasks in the network, mostly oriented around message passing interface (MPI) collective operations.
This in-network computing speeds up distributed applications, enabling faster problem solving. It also frees up server CPU cores and improves energy efficiency. InfiniBand can also automatically balance traffic loads and reroute connections around broken links.
As a result, many computing clusters that are dedicated to AI, HPC, big data, or other scientific computing run on an InfiniBand network to provide the highest possible performance and efficiency. When distributed computing performance is the top priority, and the adoption of a specialized stack of network adapters, switches, and management is acceptable, InfiniBand is the network of choice. But a data center might choose to run Ethernet instead of InfiniBand, for other reasons.
What is RoCE?
RDMA over Converged Ethernet (RoCE) is an open standard enabling remote direct memory access and network offloads over an Ethernet network. The current and most popular implementation is RoCEv2. It uses an InfiniBand communication layer running on top of UDP (Layer 4) and IP (Layer 3), which runs on top of high-speed Ethernet (Layer 2) connections.
It also supports remote direct memory access, zero-copy data transfers, and bypassing the CPU when moving data. Using the IP protocol on Ethernet enables RoCEv2 to be routable over standard Ethernet networks. RoCE brings many of the advantages of InfiniBand to Ethernet networks. RoCEv2 runs the InfiniBand transport layer over UDP and IP protocols on an Ethernet network. iWARP ran the iWARP protocol on top of the TCP protocol on an Ethernet network but failed to gain popular adoption because of performance and implementation challenges (Figure 1).
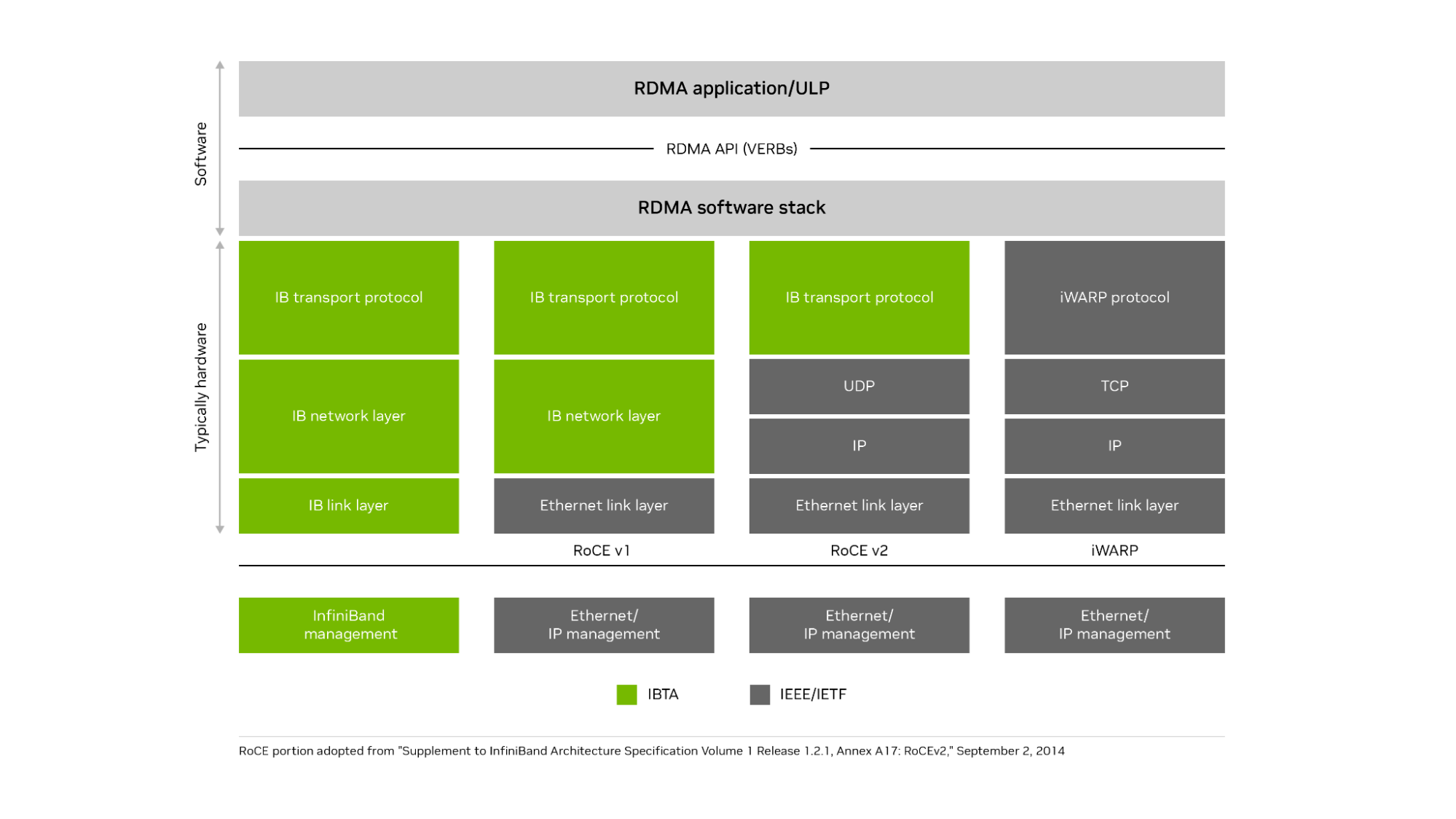
How do RoCE networks address scalability?
RoCE operates most efficiently on networks with very low levels of packet loss. Traditionally, small RoCE networks use priority flow control (PFC), based on the IEEE 802.1Qbb specification, to make the network lossless. If any destination is too busy to process all incoming traffic, it sends a pause frame to the next upstream switch port, and that switch holds traffic for the time specified in the pause frame.
If needed, the switch can also send a pause frame up to the next switch in the fabric and eventually onto the originator of the traffic flow. This flow control avoids having the port buffers overflow on any host or switch and prevents packet loss. You can manage up to eight traffic classes with PFC, each class having its own flows and pauses separate from the others.
However, PFC has some limitations. It operates only at the Layer 2 (Ethernet) level of the Open System Interconnection (OSI) 7-layer model, so it cannot work across different subnets. While a subnet can have thousands or millions of nodes, a typical subnet is limited to 254 IP addresses and consists of a few racks (often one rack) within a data center, which does not scale for large distributed applications.
PFC operates on a coarse-grained port level and cannot distinguish between flows sharing that port. Also, if you use PFC in a multi-level switch fabric, congestion at one destination switch for one flow can spread to multiple switches and block unrelated traffic flows that share one port with the congested flow. The solution is usually to implement a form of congestion control.
Congestion management for large RoCE networks
The TCP protocol includes support for congestion management based on dropped packets. When an endpoint or switch is overwhelmed by a traffic flow, it drops some packets. When the sender fails to receive an acknowledgment from the transmitted data, the sender assumes the packet was lost because of network congestion, slows its rate of transmission, and retransmits the presumably lost data.
This congestion management scheme does not work well for RDMA on Ethernet (and therefore for RoCE). RoCE does not use TCP and the process of waiting for packets to timeout and then retransmitting the lost data introduces too much latency—and too much variability in latency or jitter—for efficient RoCE operation.
Large RoCE networks often implement a more proactive congestion control mechanism known as explicit congestion notification (ECN), in which the switches mark packets if congestion occurs in the network. The marked packets alert the receiver that congestion is imminent, and the receiver alerts the sender with a congestion notification packet or CNP. After receiving the CNP, the sender knows to back off, slowing down the transmission rate temporarily until the flow path is ready to handle a higher rate of traffic.
Congestion control works across Layer 3 of the OSI model, so it functions across different subnets and scales up to thousands of nodes. However, it requires setting changes to both switches and adapters supporting RoCE traffic. Implementation details of when switches mark packets for congestion, how quickly senders back off sending data, and how aggressively senders resume high-speed transmissions are all critical to determining the scalability and performance of the RoCE network.
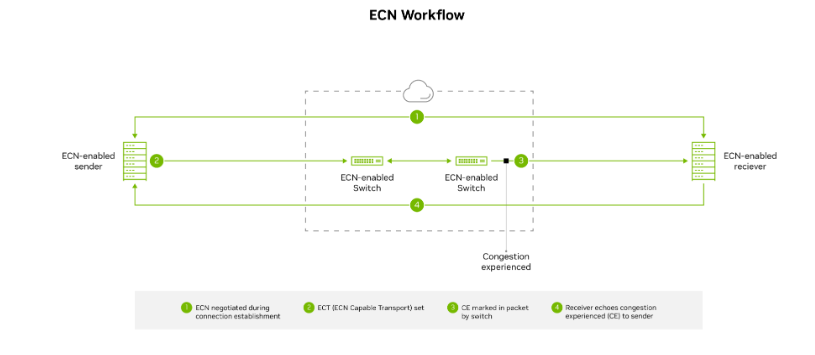
Other Ethernet-based congestion control algorithms include quantized congestion notification (QCN) and data center TCP (DCTCP). In QCN, switches notify flow senders directly with the level of potential congestion, but the mechanism functions only over L2. Consequently, it cannot work across more than one subnet. DCTCP uses the sender’s network interface card (NIC) to measure the round-trip time (RTT) of special packets to estimate how much congestion exists and how much the sender must slow down data transmissions.
But DCTCP lacks a fast start option to quickly start or resume sending data when no congestion exists, places a heavy load on host CPUs, and does not have a good mechanism for the receiver to communicate with the sender. In any case, DCTCP requires TCP, so it does not work with RoCE.
Smaller RoCE networks using newer RDMA-capable ConnectX SmartNICs from NVIDIA, or newer NVIDIA BlueField DPUs, can use Zero Touch RoCE (ZTR). ZTR enables excellent RoCE performance without setting up PFC or ECN on the switch, which greatly simplifies network setup. However, initial deployments of ZTR have been limited to small RoCE network clusters, and a more scalable version of ZTR that uses RTT for congestion notification is still in the proving stages.
How OCI implements a scalable RoCE network
OCI determined that certain cloud workloads required RDMA for maximum performance. These include AI, HPC, Exadata, autonomous databases, and other GPU-powered applications. Out of the two standardized RDMA options on Ethernet, they chose RoCE for its performance and wider adoption.
The RoCE implementation needed to scale to run across clusters containing thousands of nodes and deliver consistently low latency to ensure an excellent experience for cloud customers.
After substantial research, testing, and careful design, OCI decided to customize their own congestion control solution based on the data center quantized congestion notification (DC-QCN) algorithm, which they optimized for different RoCE-accelerated application workloads. The OCI DC-QCN solution is based on ECN with minimal use of PFC.
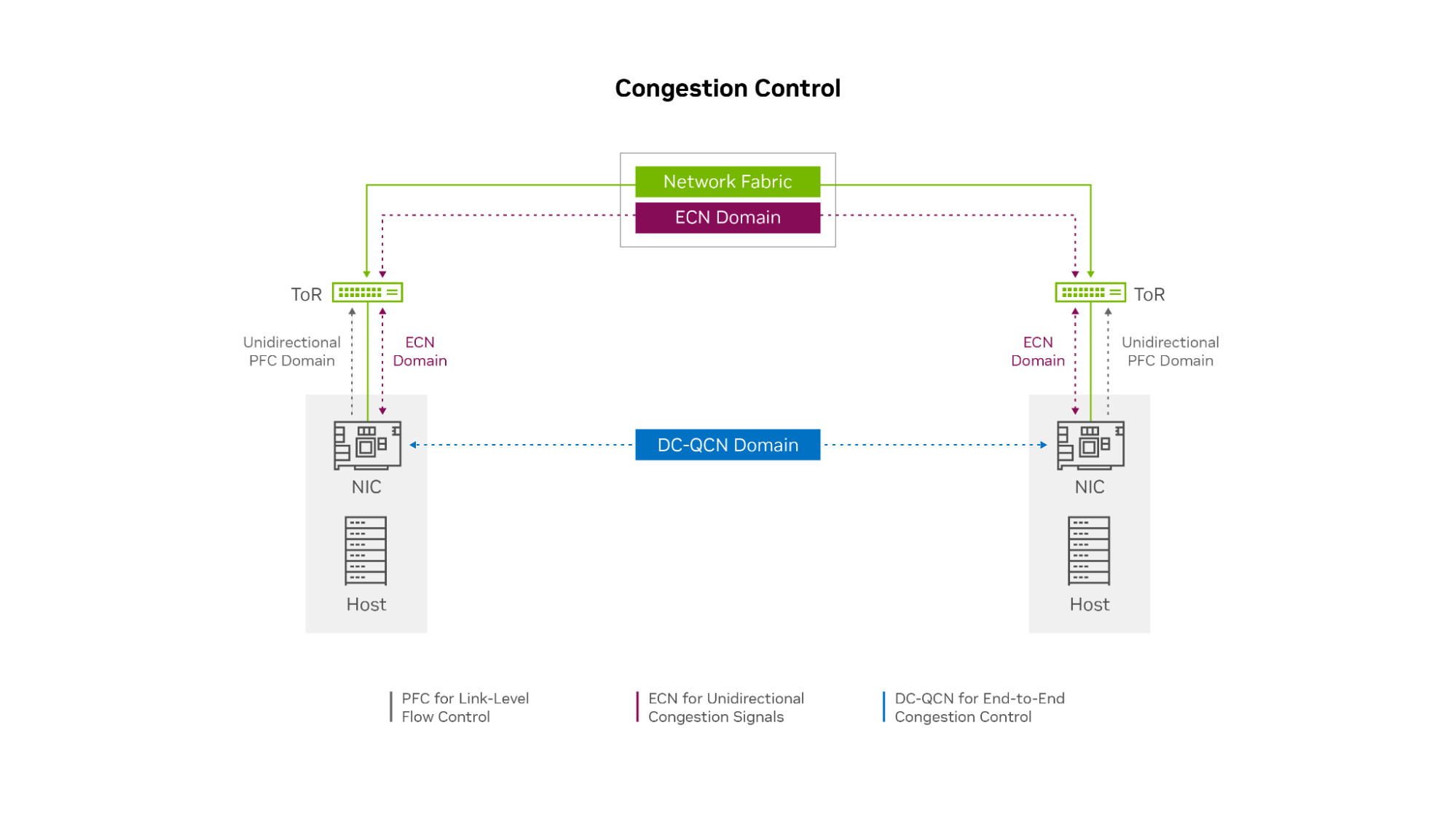
A separate network for RoCE
OCI built a separate network for RoCE traffic because the needs of the RDMA network tend to differ from the regular data center network. The different types of application traffic, congestion control, and routing protocols each prefer to have their own queues. Each NIC typically supports only eight traffic classes, and the NIC and switch configuration settings and firmware might be different for RDMA from non-RDMA workloads. For these reasons, having a separate Ethernet network for RoCE traffic and RoCE-accelerated applications makes sense.
Limited use of PFC at the edge
OCI implemented a limited level of PFC, only unidirectionally at the network edge. Endpoints can ask the top-of-rack (ToR) switch to pause transmission if their NICs buffer fills up. However, the ToR switches never ask the endpoints to pause and do not pass pause requests up the network to leaf or spine switches. This process prevents head-of-line blocking and congestion spreading if the incoming traffic flow rate temporarily exceeds the receiver’s ability to process and buffer data.
The ECN mechanism ensures that PFC is very rarely needed. In the rare case that a receiving node’s NIC buffer is temporarily overrun while the ECN feedback mechanism is activating, PFC enables the receiving node to briefly pause the incoming data flow until the sender receives the CNPs and slows its transmission rate.
In this sense, you can use PFC as a last resort safeguard to prevent buffer overrun and packet loss at the network edge (at the endpoints). OCI envisions that with the next generation of ConnectX SmartNICs, you might not need PFC, even at the edge of the network.
Multiple classes of congestion control
OCI determined that they need at least three customized congestion control profiles within DC-QCN for different workloads. Even within the world of distributed applications that require RDMA networking, the needs vary across the following categories:
- Latency sensitive, requiring consistently low latency throughput
- Sensitive, high throughput
- Mixed, requires a balance of low-latency and high throughput
The primary setting for customizing congestion control is the probability P (ranging from 0 to 1) of the switch adding the ECN marking to an outgoing packet, based on queue thresholds Kmin and Kmax. P starts at 0 when the switch queue is not busy, which means it has no chance of congestion.
When the port queue reaches Kmin, the value P rises above 0, increasing the chance that any packet is marked with ECN. When the queue fills to value Kmax, P is set to Pmax (typically 1), meaning every outgoing packet of that flow on that switch is marked with ECN. Different DC-QCN profiles typically have a no-congestion range where P is 0, a potential congestion range where P is between 0 and 1, and a congestion range where P is 1.
A more aggressive set of thresholds has lower values for Pmin and Pmax, resulting in earlier ECN packet marking and lower latency but possibly also lower maximum throughput. A relaxed set of thresholds has higher values for Pmin and Pmax, marking fewer packets with ECN, resulting in some higher latencies but also higher throughput.
To the right side of Figure 4 are three examples of OCI workloads: HPC, Oracle Autonomous DataBase and Exadata Cloud Service, and GPU workloads. These services use different RoCE congestion control profiles. HPC workloads are latency-sensitive and give up some throughput to guarantee lower latency. Consequently, Kmin and Kmax are identical and low (aggressive), and at a low amount of queuing, they mark 100% of all packets with ECN.
Most GPU workloads are more forgiving on latency but need maximum throughput. The DC-QCN profile gradually marks more packets as buffers ramp from Kmin to Kmax and sets those values relatively higher to enable switch buffers to get closer to full before signaling to flow endpoints that they slow down.
For Autonomous Database and Exadata Cloud Service workloads, the required balance of latency and bandwidth is in between. Marking or increasing P value gradually increases between Kmin and Kmax, but these values are set at lower threshold values than for GPU workloads.
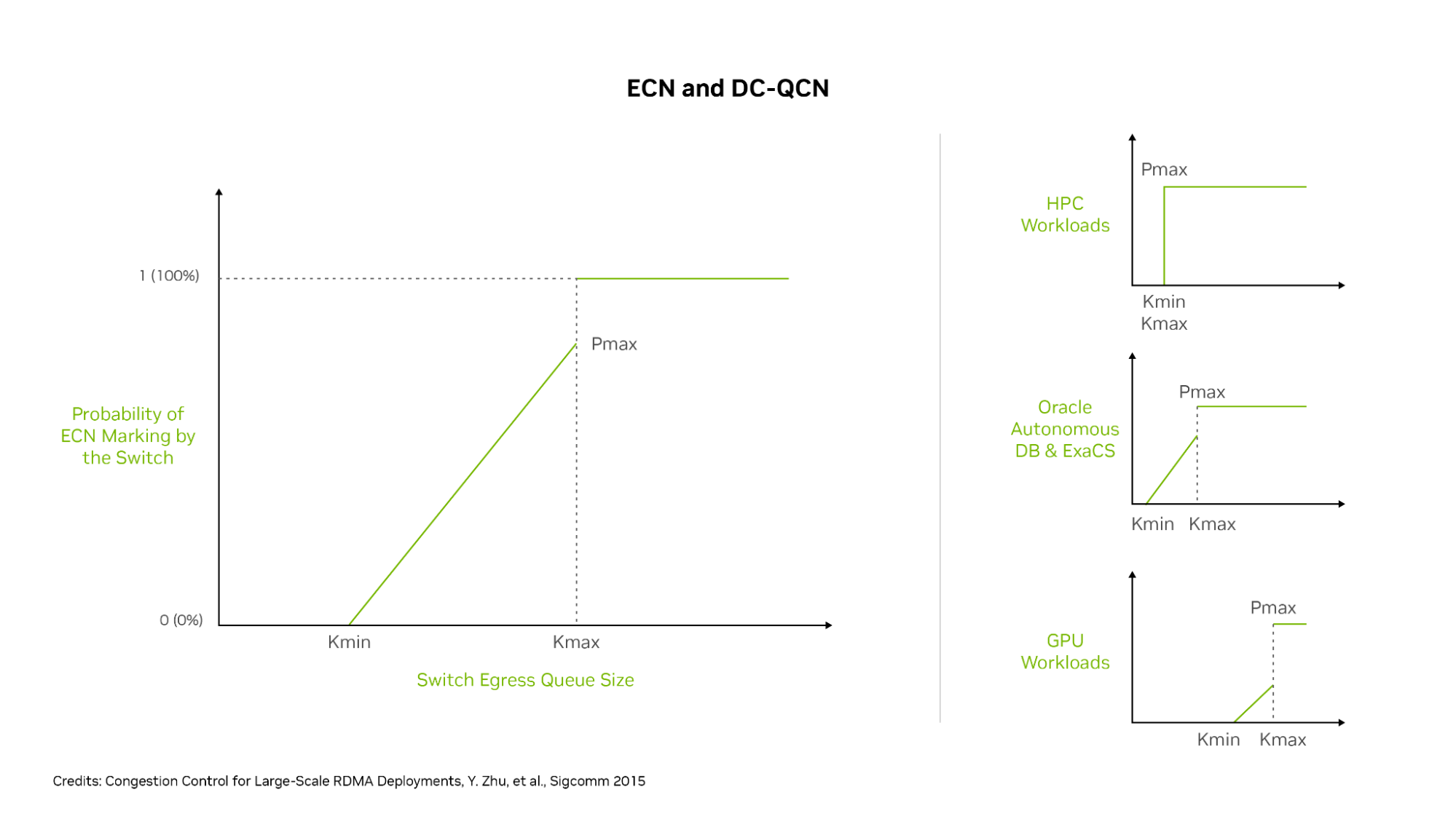
With these settings, HPC flows get 100% ECN packet marking as soon as the queues hit the Kmin level (which is the same here as Kmax) for early and aggressive congestion control engagement. Oracle Autonomous Database and Exadata flows see moderately early ECN marking, but only a portion of packets is marked until buffers reach the Kmax level.
Other GPU workloads have a higher Kmin setting so ECN marking does not begin until switch queues are relatively fuller, and 100% ECN marking only happens when the queues are close to full. Different workloads get the customized congestion control settings needed to provide the ideal balance of latency and throughput for maximum application performance.
Leveraging advanced network hardware
An important factor in achieving high performance for RoCE networks is the type of network card used. The NIC offloads the networking stack, including RDMA, to a specialized chip to offload the work from the CPUs and GPUs. OCI uses ConnectX SmartNICs, which have market-leading network performance for both TCP and RoCE traffic.
These SmartNICs also support rapid PFC and ECN reaction times for detecting ECN-marked packets or PFC pause frames, sending CNPs, and adjusting the data transmission rates downward and upward in response to congestion notifications.
NVIDIA has been a long time leader in the development and support of RDMA, PFC, ECN, and DC-QCN technology, and a leader in high-performance GPUs and GPU connectivity. The advanced RoCE offloads in ConnectX enable higher throughput and lower latency on the OCI network, and their rapid, hardware-based ECN reaction times help ensure that DC-QCN functions smoothly.
By implementing an optimized congestion control scheme on a dedicated RoCE network, plus a combination of localized PFC, multiple congestion control profiles, and NVIDIA network adapters, OCI has built a very scalable cluster network. It’s ideal for distributed workloads, such as AI and ML, HPC, and Oracle Autonomous Database, and delivers high throughput and low-latency performance close to what an InfiniBand network can achieve.
Emphasizing data locality
With optimizing cluster network performance, OCI also manages data locality to minimize latency. With the large size of RoCE-connected clusters that often span multiple data center racks and halls, even in an era of 100-, 200-, and 400-Gbps networking connections, the speed of light has not changed, and longer cables result in higher latency.
Connections to different halls in the data center traverse more switches, and each switch hop adds some nanoseconds to connection latency. OCI shares server locality information with both its customers and the job scheduler, so they can schedule jobs to use servers and GPUs that are close to each other in the network.
For example, the NVIDIA Collective Communication Library (NCCL) understands the OCI network topology and server locality information and can schedule GPU work accordingly. So, the compute and storage connections traverse fewer switch hops and shorter cable lengths, to reduce the average latency within the cluster.
It also sends less traffic to spine switches, simplifying traffic routing and load-balancing decisions. OCI also worked with its switch vendors to make the switches more load-aware, so flows can be routed to less-busy connections. Each switch generally has two connections up and down the network, enabling multiple datapaths for any flow.
Conclusion
By investing in a dedicated RoCE network with an optimized implementation of DC-QCN, advanced ConnectX NICs, and customized congestion control profiles, OCI delivers a highly scalable cluster that supports accelerated computing for many different workloads and applications. OCI cluster networks simultaneously deliver high throughput and low latency. For small clusters, latency-half the round trip time-can be as little as 2 microseconds. For large clusters, latency is typically under 4 microseconds. For extremely large superclusters, latencies are in the range of 4-8 microseconds, with most traffic seeing latencies at the lower end of this range.
Oracle Cluster Infrastructure uses an innovative approach to deliver scalable, RDMA-powered networking on Ethernet for a multitude of distributed workloads, providing higher performance and value to their customers.
For more information, see the following resources:
Heterogeneous computing architectures—those that incorporate a variety of processor types working in tandem—have proven extremely valuable in the continued…
Heterogeneous computing architectures—those that incorporate a variety of processor types working in tandem—have proven extremely valuable in the continued scalability of computational workloads in AI, machine learning (ML), quantum physics, and general data science.
Critical to this development has been the ability to abstract away the heterogeneous architecture and promote a framework that makes designing and implementing such applications more efficient. The most well-known programming model that accomplishes this is CUDA Toolkit, which enables offloading work to thousands of GPU cores in parallel following a single-instruction, multiple-data model.
Recently, a new form of node-level coprocessor technology has been attracting the attention of the computational science community: the quantum computer, which relies on the non-intuitive laws of quantum physics to process information using principles such as superposition, entanglement, and interference. This unique accelerator technology may prove useful in very specific applications and is poised to work in tandem with CPUs and GPUs, ushering in an era of computational advances previously deemed unfeasible.
The question then becomes: If you enhance an existing classically heterogeneous compute architecture with quantum coprocessors, how would you program it in a manner fit for computational scalability?
NVIDIA is answering this question with CUDA Quantum, an open-source programming model extending both C++ and Python with quantum kernels intended for compilation and execution on quantum hardware.
This post introduces CUDA Quantum, highlights its unique features, and demonstrates how researchers can leverage it to gather momentum in day-to-day quantum algorithmic research and development.
CUDA Quantum: Hello quantum world
To begin with a look at the CUDA Quantum programming model, create a two-qubit GHZ state with the Pythonic interface. This will accustom you to its syntax.
import cudaq
# Create the CUDA Quantum Kernel
kernel = cudaq.make_kernel()
# Allocate 2 qubits
qubits = kernel.qalloc(2)
# Prepare the bell state
kernel.h(qubits[0])
kernel.cx(qubits[0], qubits[1])
# Sample the final state generated by the kernel
result = cudaq.sample(kernel, shots_count = 1000)
print(result)
{11:487, 00:513}
The language specification borrows concepts that CUDA has proven successful; specifically, the separation of host and device code at the function boundary level. The code snippet below demonstrates this functionality on a GHZ state preparation example in C++.
#include
int main() {
// Define the CUDA Quantum kernel as a C++ lambda
auto ghz =[](int numQubits) __qpu__ {
// Allocate a vector of qubits
cudaq::qvector q(numQubits);
// Prepare the GHZ state, leverage standard
// control flow, specify the x operation
// is controlled.
h(q[0]);
for (int i = 0; i (q[i], q[i + 1]);
};
// Sample the final state generated by the kernel
auto results = cudaq::sample(ghz, 15);
results.dump();
return 0;
}
CUDA Quantum enables the definition of quantum code as stand-alone kernel expressions. These expressions can be any callable in C++ (a lambda is shown here, and implicitly typed callable) but must be annotated with the __qpu__
attribute enabling the nvq++ compiler to compile them separately. Kernel expressions can take classical input by value (here the number of qubits) and leverage standard C++ control flow, for example for loops and if statements.
The utility of GPUs
The experimental efforts to scale up QPUs and move them out of research labs and host them on the cloud for general access have been phenomenal. However, current QPUs are noisy and small-scale, hindering advancement of algorithmic research. To aid this, circuit simulation techniques are answering the pressing requirements to advance research frontiers.
Desktop CPUs can simulate small-scale qubit statistics; however, memory requirements of the state vector grow exponentially with the number of qubits. A typical desktop computer possesses eight GB of RAM, enabling one to sluggishly simulate approximately 15 qubits. The latest NVIDIA DGX H100 enables you to surpass the 35-qubit mark with unparalleled speed.
Figure 1 shows a comparison of CUDA Quantum on CPU and GPU backend for a typical variational algorithmic workflow. The need for GPUs is evident here, as the speedup at 14 qubits is 425x and increases with qubit count. Extrapolating to 30 qubits, the CPU-to-GPU runtime is 13 years, compared to 2 days. This unlocks researchers’ abilities to go beyond small-scale proof of concept results to implementing algorithms closer to real-world applications.
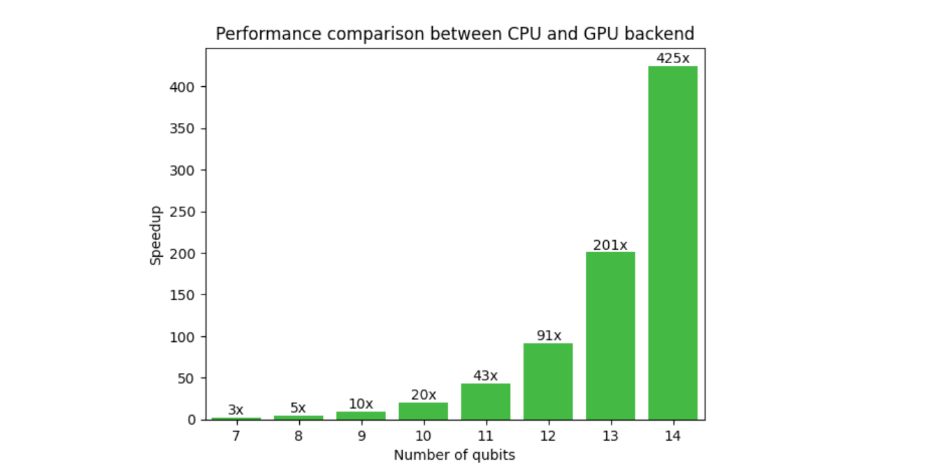
Along with CUDA Quantum, NVIDA has developed cuQuantum, a library enabling lightning-fast simulation of a quantum computer using both state vector and tensor network methods through hand-optimized CUDA kernels. Memory allocation and processing happens entirely on GPUs resulting in dramatic increases in performance and scale. CUDA Quantum in combination with cuQuantum forms a powerful platform for hybrid algorithm research.
Figure 2 compares CUDA Quantum with a leading quantum computing SDK, both leveraging the NVIDIA cuQuantum backend to optimally offload circuit simulation onto NVIDIA GPUs. In this case, the benefits of using CUDA Quantum are isolated and yield a 5x performance improvement on average compared to a leading framework.
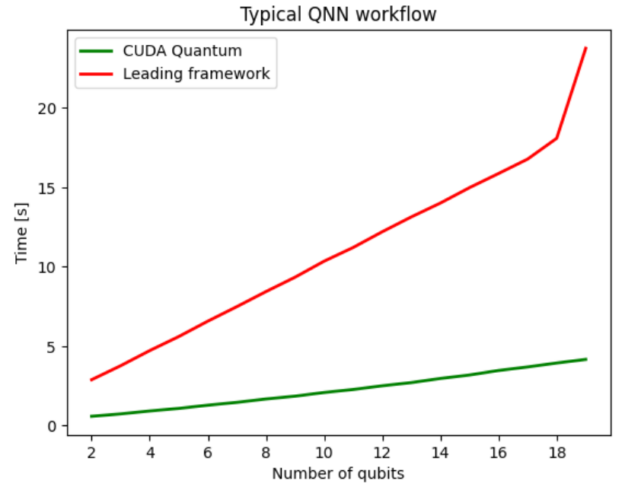
Enabling multi-QPU workflows of the future
CUDA Quantum is not limited to consideration of current cloud-based quantum execution models, but is fully anticipating tightly coupled, system-level quantum acceleration. Moreover, CUDA Quantum enables application developers to envision workflows for multi-QPU architectures with multi-GPU backends.
For the preceding quantum neural network (QNN) example, you can use the multi-GPU functionality to run a forward pass of the dataset enabling us to perform multi-QPU workflows of the future. Figure 3 shows results for distributing the QNN workflow across two GPUs and demonstrates strong scaling performance indicating effective usage of all GPU compute resources. Using two GPUs makes the overall workflow twice as fast compared to a single GPU, demonstrating strong scaling.

Another common workflow that benefits from multi-QPU parallelization is the Variational Quantum Eigensolver (VQE). This requires the expectation value of a composite Hamiltonian made up of multiple single Pauli tensor product terms. The CUDA Quantum observe call, shown below, automatically batches terms (Figure 4), and offloads to multiple GPUs or QPUs if available, demonstrating strong scaling (Figure 5).
numQubits, numTerms = 30, 1e5
hamiltonian = cudaq.SpinOperator.random(numQubits, numTerms)
cudaq.observe(ansatz, hamiltonian, parameters)
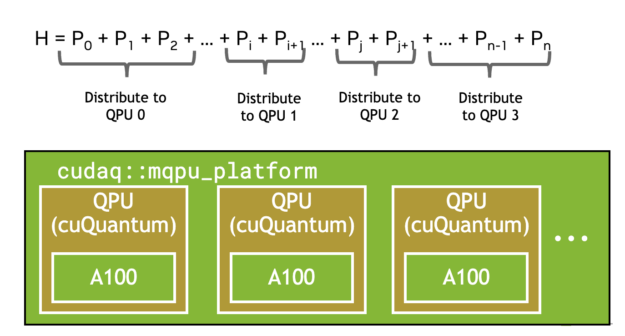
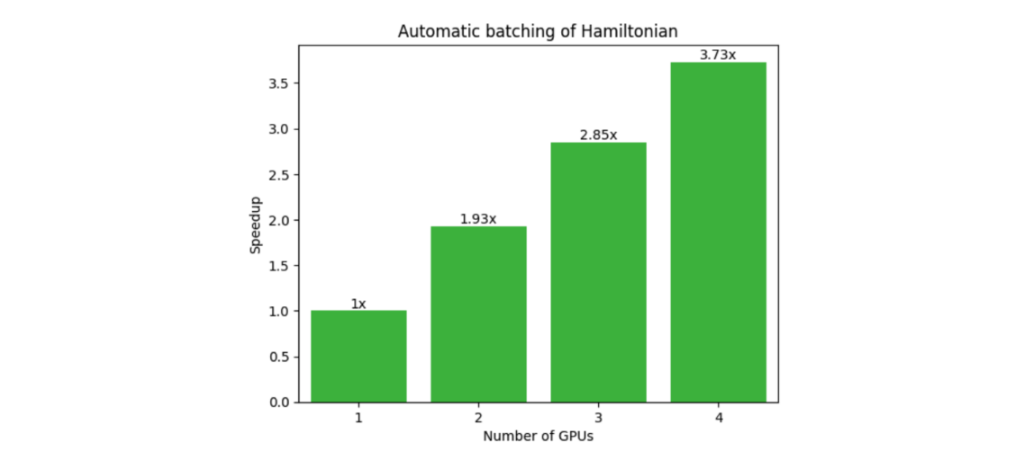
GPU-QPU workflows
This post has so far explored using GPUs for scaling quantum circuit simulation beyond what is possible on CPUs, as well as multi-QPU workflows. The following sections dive into true heterogeneous computing with a hybrid quantum neural network example using PyTorch and CUDA Quantum.
As shown in Figure 6, a hybrid quantum neural network encompasses a quantum circuit as a layer within the overall neural network architecture. An active area of research, this is poised to be advantageous in certain areas, improving generalization errors.
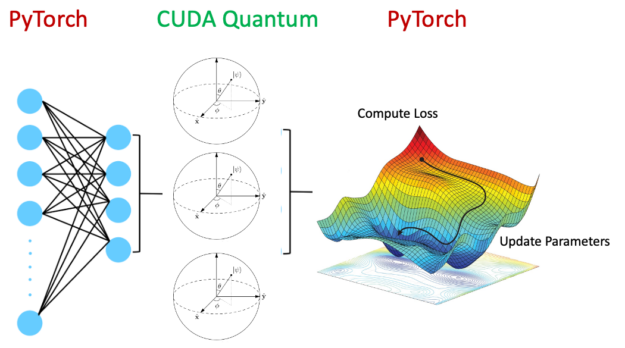
Evidently, it is advantageous to run the classical neural network layers on GPUs and the quantum circuits on QPUs. Accelerating the whole workflow with CUDA Quantum is made possible by setting the following:
quantum_device = cudaq.set_target('ion-trap')
classical_device = torch.cuda.set_device(gpu0)
The utility of this is profound. CUDA Quantum enables offloading relevant kernels suited for QPUs and GPUs in a tightly integrated, seamless fashion. In addition to hybrid applications, workflows involving error correction, real-time optimal control, and error mitigation through Clifford data regression would all benefit from tightly coupled compute architectures.
QPU hardware providers
The foundational information unit embedded within the CUDA Quantum programming paradigm is the qudit, which represents a quantum bit capable of accessing d-states. Qubit is a specific instance where d=2. By using qudits, CUDA Quantum can efficiently target diverse quantum computing architectures, including superconducting circuits, ion traps, neutral atoms, diamond-based, photonic systems, and more.
You can conveniently develop workflows, and the nvq++ compiler automatically compiles and executes the program on the designated architecture. Figure 7 shows the compilation speedups that the novel compiler yields. Compilation involves circuit optimization, decomposing into the native gate sets supported by the hardware and qubit routing. The nvq++ compiler used by CUDA Quantum is on average 2.4x faster compared to its competition.
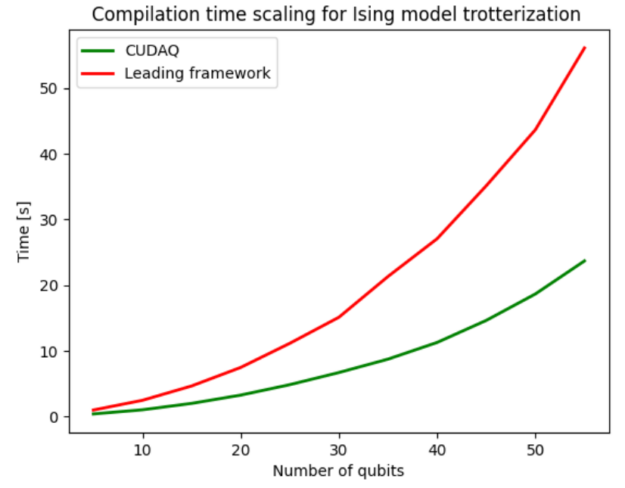
To accommodate the desired backend, you can simply modify the set_target()
flag. Figure 8 shows an example of how you can seamlessly switch between the simulated backend and the Quantinuum H1 ion trap system. The top shows the syntax to set the desired backend in Python and the bottom in C++.
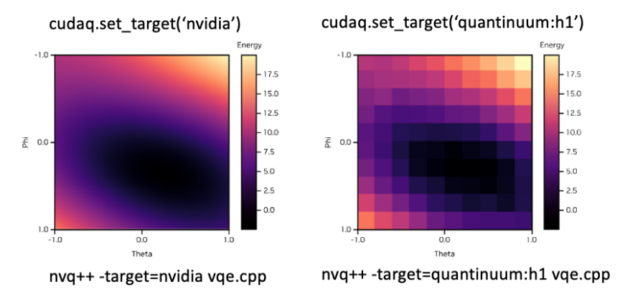
Getting started with CUDA Quantum
This post has just briefly touched on some of the features of the CUDA Quantum programming model. Reach out to the CUDA Quantum community on GitHub and get started with some example code snippets. We are excited to see the research CUDA Quantum enables for you.