Cloud-native is one of the most important concepts associated with deploying edge AI applications. Find out how to get AI applications cloud-native ready.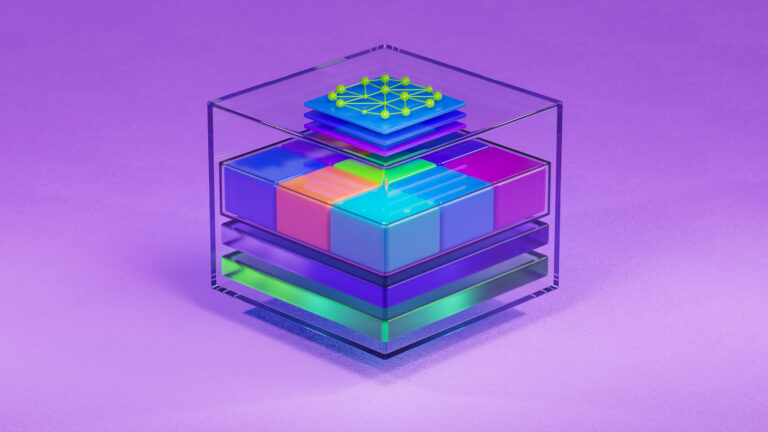
Sign up for Edge AI News to stay up to date with the latest trends, customers use cases, and technical walkthroughs.
Cloud-native is one of the most important concepts associated with edge AI. That’s because cloud-native delivers massive scale for application deployments. It also delivers performance, resilience, and ease of management, all critical capabilities for edge AI. Cloud-native and edge AI are so entwined that we believe the future of edge AI is cloud-native.
This post is an overview of cloud-native components and the steps to get an application cloud-native ready. I show you how to practice using these concepts with NVIDIA Fleet Command, a cloud service for deploying and managing applications at the edge built with cloud-native principles.
If all these steps are followed, the result is a cloud-native application that can be easily deployed on Fleet Command and other cloud-native deployment and management platforms.
What is cloud-native?
Cloud-native is an approach to developing and operating applications that encompass the flexibility, scalability, and resilience of the cloud computing delivery model. The cloud-native approach allows organizations to build applications that are resilient and manageable, allowing more agile application deployments.
There are key principles of cloud-native development:
- Microservices
- Containers
- Helm charts
- CI/CD
- DevOps
What are microservices?
Microservices are a form of software development where an application is broken down into smaller, self-contained services that communicate with each other. These self-contained services are independent, meaning each of them can be updated, deployed, and scaled on their own without affecting the other services in the application.
Microservices make developing applications faster and the process of updating and deploying and scaling those updates easier.
What are containers?
A container is a software package that contains all of the information and dependencies necessary to run an application reliably in any computing environment. Containers can be easily deployed on different operating systems and offer portability and application isolation.
A whole application can be containerized, but pieces of an application can be containerized as well. For instance, containers work extremely well with microservices where applications are broken into small, self-sufficient components. Each microservice can be packaged, deployed, and managed in containers). Additionally, multiple containers can be deployed and managed in clusters.
Containers are perfect for edge deployments because they enable you to install your application, dependencies, and environment variables one time into the container image, rather than on each system that the application runs on, making managing multiple deployments significantly easier.
That’s important for edge commuting because an organization may need to install and manage hundreds or thousands of different deployments across a vast physical distance, so automating as much of the deployment process as possible is critical.
What are Helm charts?
For complex container deployments, such as deploying multiple applications across several sites with multiple systems, many organizations use Helm charts. Helm is an application package manager running on top of Kubernetes (discussed later). Without it, you have to manually create separate YAML files for each workload that specifies all the details needed for a deployment, from pod configurations to load balancing.
Helm charts eliminate this tedious process by allowing organizations to define reusable templates for deployments, in addition to other benefits like versioning and the capability to customize applications mid-deployment.
What is CI/CD?
Continuous integration (CI) enables you to iterate and test new code collaboratively, usually by integrating it into a shared repository.
Continuous delivery (CD) is the automated process of taking new builds from the CI phase and loading them into a repository where they can easily be deployed into production.
A proper CI/CD process enables you to avoid disruptions in service when integrating new code into existing solutions.
What is DevOps?
The term DevOps refers to the process of merging developer and operations groups to streamline the process for developing and delivering applications to customers.
DevOps is important for cloud-native technologies, as the philosophies of both concepts are focused on delivering solutions to customers continuously and easily, and creating an end-to-end development pipeline to accelerate updates and iterations.
What is cloud-native management?
Now that the core principles of cloud-native have been explained, it is important to discuss how to manage cloud-native applications in production.
The leading platform for orchestrating containers is Kubernetes. Kubernetes is open source and allows organizations to deploy, manage, and scale containerized applications.
Several organizations have built enterprise-ready solutions on top of Kubernetes to offer unique benefits and functionality:
The process for getting an application ready for any Kubernetes platform, whether Kubernetes itself or a solution built on top of Kubernetes, is essentially the same. Each solution has specific configuration steps needed to ensure an organization’s cloud-native applications can run effectively without issue.
Deploying a cloud-native application with NVIDIA Fleet Command
This section walks through the configuration process by using NVIDIA Fleet Command as an example and noting the specific configurations needed.
Step 0: Understand Fleet Command
Fleet Command is a cloud service for managing applications across disparate edge locations. It’s built on Kubernetes and deploys cloud-native applications, so the steps to get an application onto Fleet Command are the same steps to get an application onto other cloud-native management platforms.
Assuming the application is already built, there are just four steps to get that application onto Fleet Command:
- Containerize the application
- Determine the application requirements
- Build a Helm chart
- Deploy on Fleet Command
Step 1: Containerize the application
Fleet Command deploys applications as containers. By using containers, you can deploy multiple applications on the same system and also easily scale the application across multiple systems and locations. Also, all the dependencies are packed inside of the container, so you know that the application will perform the same across thousands of systems.
Building a container for an application is easy. For more information, see the Docker guide on containers.
Here’s an example of a Dockerfile for a customized deep learning container built using an NVIDIA CUDA base image:
FROM nvcr.io/nvidia/cuda:11.3.0-base-ubuntu18.04
CMD nvidia-smi
#set up environment
RUN apt-get update && apt-get install --no-install-recommends --no-install-suggests -y curl
RUN apt-get install unzip
RUN apt-get -y install python3
RUN apt-get -y install python3-pip
#copies the application from local path to container path
COPY app/ /app/
WORKDIR /app
#Install the dependencies
RUN pip3 install -r /app/requirements.txt
ENV MODEL_TYPE='EfficientDet'
ENV DATASET_LINK='HIDDEN'
ENV TRAIN_TIME_SEC=100
CMD ["python3", "train.py"]
In this example, /app/
contains all the source code. After a Dockerfile is created for the container, a container can be built using the file and then uploaded to a private registry in the cloud so that the container can be easily deployed anywhere.
Step 2: Determine the application requirements
When the container is complete, it is necessary to determine what the application requires to function properly. This typically involves considering security, networking, and storage requirements.
Fleet Command is a secured software stack that offers the ability to control which hardware and software the application has access to within the system on which it is deployed. As a result, there are security best practices, which your application should be designed around:
- Avoiding privileged containers
- Separating your admin and app traffic from your storage traffic
- Minimizing system device access
- And so on
Design your application deployment around these security requirements, keeping them in mind when configuring the networking and storage later.
The next step is to determine what networking access requirements are needed and how to expose the networking from your container.
Typically, an application requires different ports and routes to access any edge sensors and devices, admin traffic, storage traffic, and application (cloud) traffic. These ports can be exposed from Fleet Command using either NodePorts
or more advanced Kubernetes networking configurations, such as the ingress controller.
Lastly, the application may require access to local or remote storage for saving persistent data. Fleet Command supports the capability for hostPath
volume mounts. Additional Kubernetes capabilities can also be used, such as persistent volumes and persistent volume claims.
Local path or NFS provisioners can be deployed separately onto the Fleet Command system, if required, to configure local or remote storage. Applications, if they support the capability, can also be configured to connect to cloud storage.
For more information, see the Fleet Command Application Development Guide.
Step 3: Build a Helm chart
Now that the application requirements have been determined, it’s time to create a Helm chart.
Like containers, there are a few specific requirements for Helm charts on Fleet Command. These requirements are described in the Helm Chart Requirements section of the Application Development Guide. Here is an example of an NVIDIA DeepStream Helm chart as a reference to help build a Helm chart deployed in Fleet Command.
To create your own Helm chart from scratch, first run the following command to create a sample Helm chart. This command generates a sample Helm chart with a NGINX Docker container, which can then be customized for any application.
$ helm create deepstream
After the Helm chart is created, this is how the chart’s directory structure appears:
deepstream
|-- Chart.yaml
|-- charts
|-- templates
| |-- NOTES.txt
| |-- _helpers.tpl
| |-- deployment.yaml
| |-- ingress.yaml
| `-- service.yaml
`-- values.yaml
Next, modify the values.yaml file with the following highlighted values to configure the sample Helm chart for DeepStream container and networking.
image:
repository: nvcr.io/nvidia/deepstream
pullPolicy: IfNotPresent
# Overrides the image tag whose default is the chart appVersion.
tag: 5.1-21.02-samples
imagePullSecrets: []
nameOverride: ""
fullnameOverride: ""
serviceAccount:
# Specifies whether a service account should be created
create: false
# Annotations to add to the service account
annotations: {}
# The name of the service account to use.
# If not set and create is true, a name is generated using the fullname template
name: ""
podAnnotations: {}
podSecurityContext: {}
# fsGroup: 2000
securityContext: {}
# capabilities:
# drop:
# - ALL
# readOnlyRootFilesystem: true
# runAsNonRoot: true
# runAsUser: 1000
service:
type: NodePort
port: 8554
nodeport: 31113
After you create a customized Helm chart, it can then be uploaded to a private registry alongside the container.
Step 4: Deploy on Fleet Command
With the application containerized and a Helm chart built, load the application onto Fleet Command. Applications are loaded on NGC, a hub for GPU-accelerated applications, models, and containers, and then made available to deploy on Fleet Command. The application can be public, but can also be hosted in a private registry where access is restricted to the organization.
The entire process is covered step-by-step in the Fleet Command User Guide, but is also showcased in the Fleet Command demo video.
Bonus step: Join our ecosystem of partners
Recently, NVIDIA announced an expansion of the NVIDIA Metropolis Partner Program that now includes Fleet Command. Partners of Metropolis that configure their application to be deployed on Fleet Command have access to the solution for free to operate POCs for customers. By using Fleet Command, partners don’t need to build a bespoke solution in a customer environment for an evaluation. They can use Fleet Command and deploy their application at customer sites in minutes.
Get started on cloud-native
This post covered the core principles of cloud-native technology and how to get applications cloud-native ready with Fleet Command.
Your next step is to get hands-on experience deploying and managing applications in a cloud-native environment. NVIDIA LaunchPad can help.
LaunchPad provides immediate, short-term access to a Fleet Command instance to easily deploy and monitor real applications on real servers. Hands-on labs walk you through the entire process, from infrastructure provisioning and optimization to application deployment in the context of applicable use cases, like deploying a vision AI application at the edge of a network.
Get started on LaunchPad today for free.