Join this webinar on June 14 and learn how to program computer vision algorithms using VPI’s Python interface.
Gamers know NVIDIA powers great gaming experiences. Researchers know NVIDIA speeds world-changing breakthroughs. Businesses know us for the AI engines transforming their industries. And NVIDIA employees know the company as one of the best places to work on the planet. More people than ever have a piece of NVIDIA. Roboticists, visual artists, data scientists — Read article >
The post Solving the World’s Biggest Challenges, Together appeared first on NVIDIA Blog.
Monitor DPUs, validate RoCE deployments, gain network insights through flow-based telemetry analysis, and centrally view network events with NetQ 4.2.0.
NVIDIA NetQ is a highly scalable, modern networking operations tool providing actionable visibility for the NVIDIA Spectrum Ethernet platform. It combines advanced telemetry with a user interface, making it easier to troubleshoot and automate network workflows while reducing maintenance and downtime.
We have recently released NetQ 4.2.0, which includes:
- Simplified events management
- Enhanced flow telemetry analysis
- New RoCE validation
- New DPU monitoring
For more information about new features and enhancements, see the NetQ 4.2.0 User’s Guide.
Simplified events management
With NetQ 4.2, we have simplified the way network events are communicated through the interface. Events vary in terms of severity—some events are network alarms that may require further investigation, while others are informational notices that may not require intervention. Before this release, NetQ displayed alarms and information events as two separate cards. The NetQ 4.2 release merges the two cards into a single card that, when expanded, displays a dashboard to help you quickly visualize all network events.
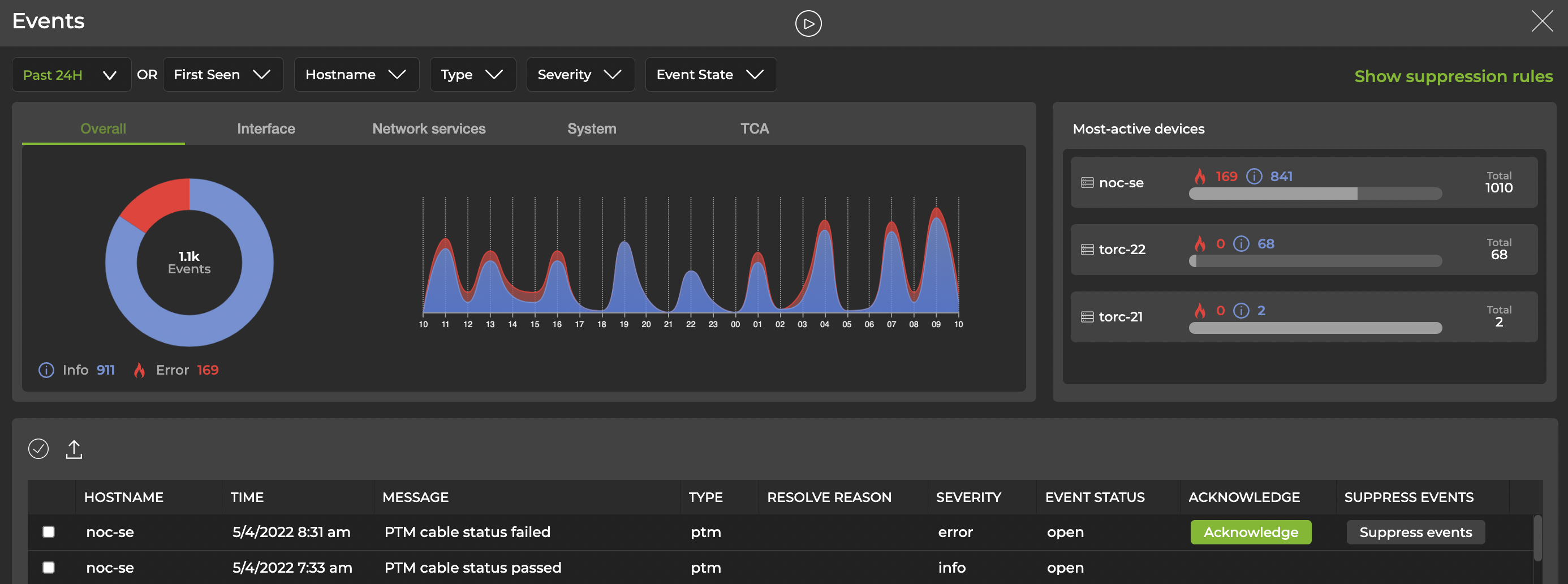
The dashboard presents a timeline of events alongside the switches that are causing the most events. You can filter events by type, including interface, network services, system, and threshold-crossing events.
Acknowledging events helps you focus on active events that need your attention. From the dashboard, you can also create rules to suppress events. This feature is also designed to help you focus on active events, so that known issues or false alarms are not displayed in the same way that errors are displayed.
Enhanced flow telemetry analysis
NetQ 4.1.0 introduced fabric-wide network latency and buffer occupancy analysis for Cumulus Linux 5.x data center fabrics. Now, NetQ 4.2 supports partial-path flow telemetry analysis in mixed fabrics—those that use Cumulus Linux 5.x switches in combination with other switches (including non-Cumulus Linux 5.x and third-party switches). Cumulus Linux 5.x devices in the path display flow statistics, such as latency and buffer occupancy. Unsupported devices are represented in the flow analysis as a black bar with a red X, and the device does not display flow statistics.

In addition, NetQ 4.2 flow telemetry analysis shows contextual ‘What Just Happened’ (WJH) events and drops for the flow under analysis. Switches with WJH events are represented in the flow analysis graph as a red, striped bar. Hovering over the device with the red bar presents a WJH events summary.
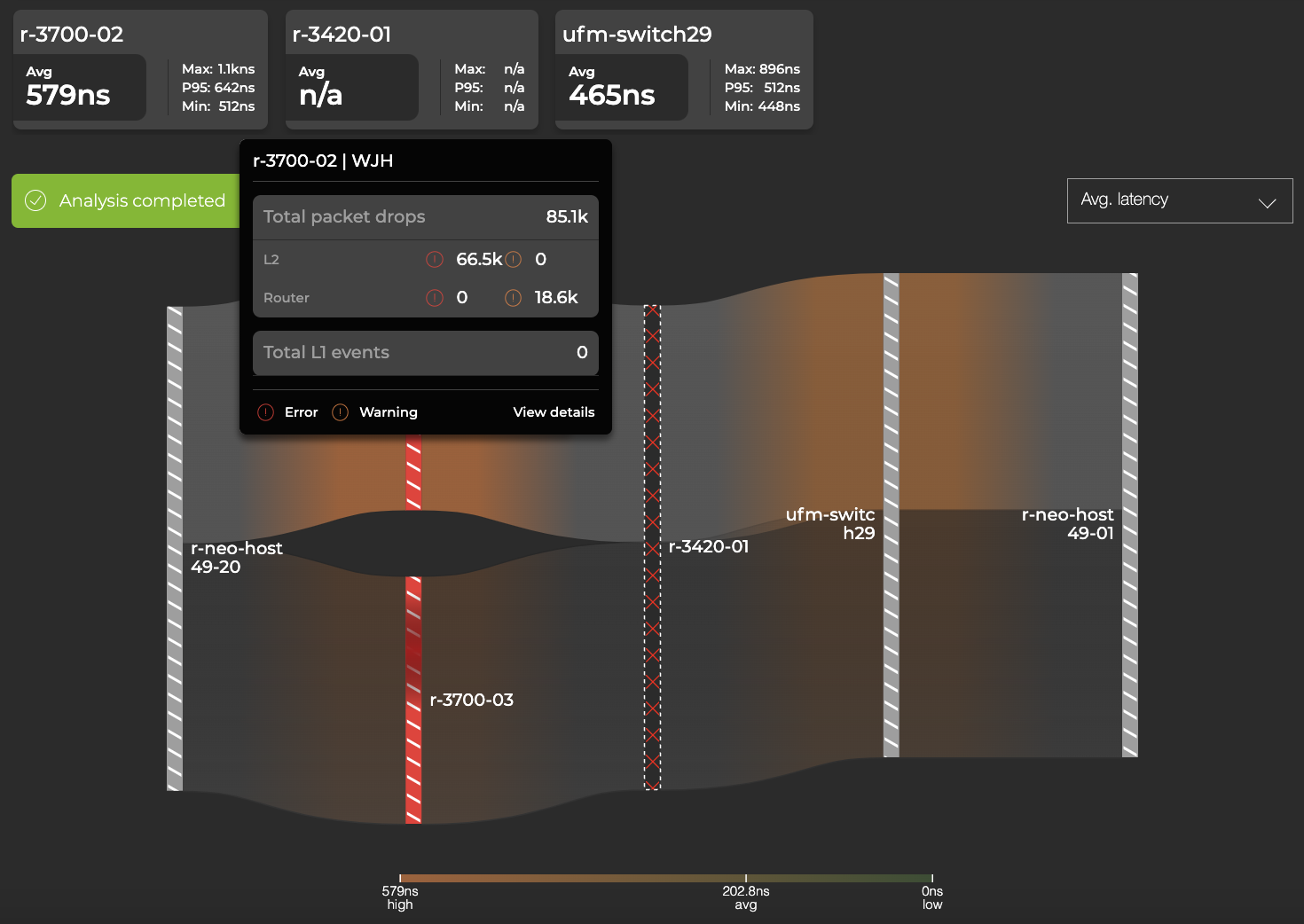
New RoCE validation
With RDMA over Converged Ethernet (RoCE), you can write to compute or storage elements using remote direct memory access (RDMA) over an Ethernet network instead of using host CPUs. NetQ 4.0.0 introduced RoCE configuration and counters, including the ability to set up various RoCE threshold-crossing alerts (TCAs).
With NetQ 4.2.0, RoCE validation checks:
- Lossy- or lossless-mode configuration consistency across switches
- Consistency of DSCP, service pool, port group, and traffic class settings
- Consistency of ECN threshold settings
- Consistency of PFC configuration for lossless mode
- Consistency of Enhanced Transmission Selection settings
You can schedule RoCE validation to run periodically or on-demand.
New DPU monitoring
NVIDIA BlueField data processing units (DPUs) provide a secure and accelerated infrastructure for any workload by offloading, accelerating, and isolating a broad range of advanced networking, storage, and security services.
NetQ helps you monitor your DPU inventory across the network. You can monitor a DPU OS, ASIC, CPU model, disk, and memory information to help manage upgrades, compliance, and other planning tasks. With NetQ, you can view and monitor key DPU attributes, including installed packages and CPU, disk, and memory utilization.
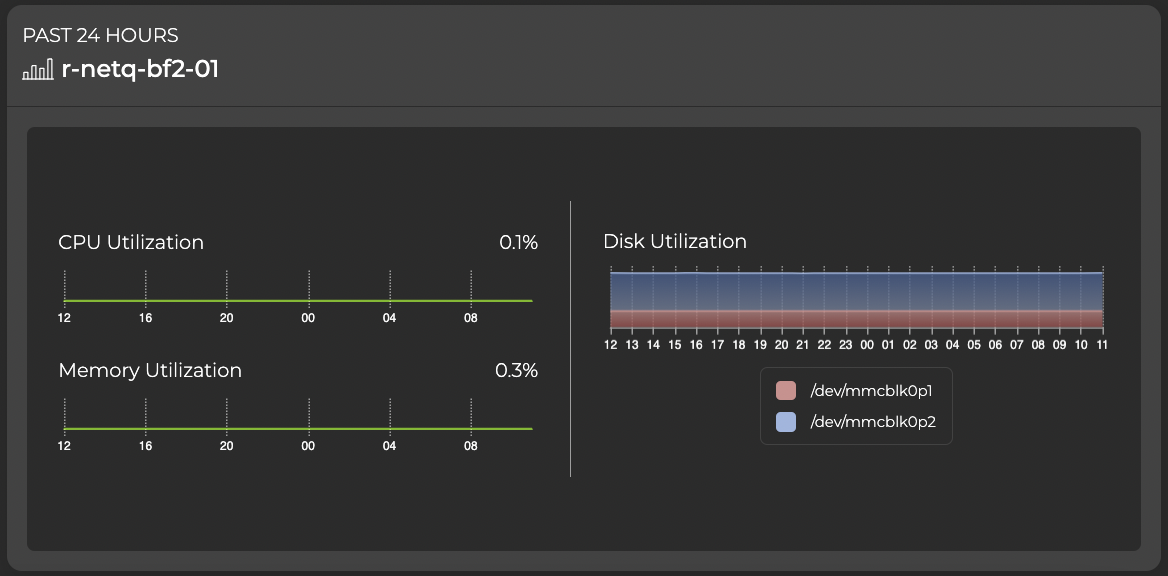
In this post, you have seen an overview of some of the new capabilities available with NetQ 4.2.0. For more information, see the NetQ 4.2.0 User’s Guide and explore NetQ with NVIDIA Air.
tensorflow lite reduce types of detections
I only need the tensorflow lite example model to detect cars and people, but it detects Many more types of objects. Is there any way to make it detect just these two
submitted by /u/MatsudaYagami
[visit reddit] [comments]
Deep Learning with R, 2nd Edition
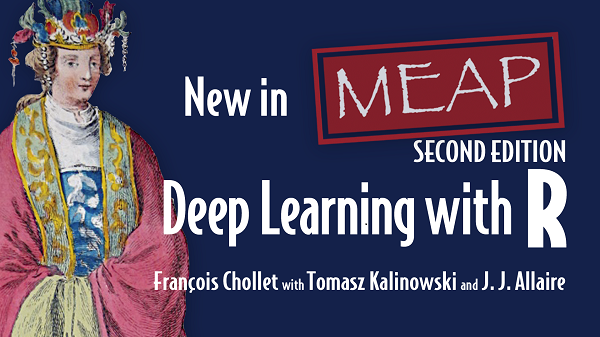
Announcing the release of “Deep Learning with R, 2nd Edition,” a book that shows you how to get started with deep learning in R.
Deep Learning with R, 2nd Edition
Announcing the release of “Deep Learning with R, 2nd Edition,” a book that shows you how to get started with deep learning in R.
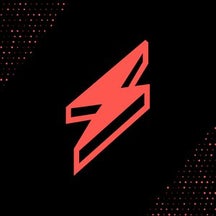
![]() |
submitted by /u/RichardGrant_ [visit reddit] [comments] |
The NVIDIA platform, powered by the A100 Tensor Core GPU, delivers leading performance and versatility for accelerated HPC.
High-performance computing (HPC) has become the essential instrument of scientific discovery.
Whether it is discovering new, life-saving drugs, battling climate change, or creating accurate simulations of our world, these solutions demand an enormous—and rapidly growing—amount of processing power. They are increasingly out of reach of traditional computing approaches.
That is why industry has embraced NVIDIA GPU-accelerated computing. Combined with AI, it is bringing millionfold leaps in performance for scientific advancement. Today, 2,700 applications can benefit from NVIDIA GPU acceleration, and that number continues to rise, backed by a growing community of three million developers.
HPC application performance improvements
Delivering the many-fold speedups across the entire breadth of HPC applications takes relentless innovation at every level of the stack. This starts with chips and systems and goes through to the application frameworks themselves.
The NVIDIA platform continues to deliver significant performance improvements each year, with relentless advancements in architecture and across the NVIDIA software stack. Compared to the P100 released just six years ago, the H100 Tensor Core GPU is expected to deliver an estimated 26x higher performance, more than 3x faster than Moore’s Law.
Core to the NVIDIA platform is a feature-rich and high-performance software stack. To facilitate GPU acceleration for the widest range of HPC applications, the platform includes the NVIDIA HPC SDK. The SDK provides unmatched developer flexibility, enabling the creation and porting of GPU-accelerated applications using standard languages, directives, and CUDA.
The power of the NVIDIA HPC SDK lies in a vast suite of highly optimized GPU-accelerated math libraries, enabling you to harness the full performance potential of NVIDIA GPUs. For the best multi-GPU and multi-node performance, the NVIDIA HPC SDK also provides powerful communications libraries:
- NVSHMEM creates a global address space for data that spans the memory of multiple GPUs.
- NVIDIA Collective Communications Library (NCCL) optimizes inter-GPU communication.
Altogether, this platform provides the highest performance and flexibility to support the large and growing universe of GPU-accelerated HPC applications.
HPC performance and energy efficiency
To showcase how the NVIDIA full-stack innovation translates into the highest performance for accelerated HPC, we compared the performance of a server from HPE with four NVIDIA GPUs with that of a similarly configured server based on an equal number of accelerator modules from another vendor.
We tested a set of five widely used HPC applications using a wide variety of datasets. While the NVIDIA platform accelerates 2,700 applications spanning every industry, the applications we could use in this comparison were limited by the selection of software and application versions that are available for the other vendor’s accelerators.
For all workloads except for NAMD, which is software for molecular dynamics simulation, our results are calculated using the geomean of results across multiple datasets to minimize the influence of outliers and to be representative of customer experiences.
We also tested these applications in multi-GPU and single-GPU scenarios.
In the multi-GPU scenario, with all accelerators in the tested systems being used to run a single simulation, the A100 Tensor Core GPU-based server delivered up to 2.1x higher performance than the alternative offering.
Fueled by continued advances in compute performance, the field of molecular dynamics is moving towards simulating ever-larger systems of atoms for longer periods of simulated time. These advances enable researchers to simulate an increasing set of biochemical mechanisms, such as photosynthetic electron transport and vision signal transduction. These and other processes have long been the subject of scientific debate because they have been beyond the reach of simulation, which is the primary tool for validation. This was due to the prohibitively long amount of time needed to complete the simulations.
However, we recognize that not all users of these applications run them with multiple GPUs per simulation. For optimal throughput, the best execution method is often to assign one GPU per simulation.
When running these same applications on a single accelerator module—a full GPU on the NVIDIA A100 and both compute dies on the alternative product—the NVIDIA A100-based system delivered up to 1.9x faster performance.
Energy costs represent a significant portion of the total cost of ownership (TCO) of data centers and supercomputing centers alike, underscoring the importance of power-efficient computing platforms. Our testing showed that the NVIDIA platform provided up to 2.8x higher throughput-per-watt than the alternative offering.
Efficiency ratio of A100 to MI250 shown – higher is better for NVIDIA. Geomean over multiple datasets (varies) per application. Efficiency is Performance / Power consumption (Watts) as measured for the GPUs using measured using NVIDIA SMI and equivalent functionality in ROCm |
AMD MI250 measured on a GIGABYTE M262-HD5-00 with (2) AMD EPYC 7763 with 4x AMD Instinct MI250 OAM (128 GB HBM2e) 500W GPUs with AMD Infinity Fabric
technology. NVIDIA runs on ProLiant XL645d Gen10 Plus using dual EPYC 7713 CPUs and 4x A100 (80 GB) SXM4
LAMMPS develop_db00b49(AMD) develop_2a35ec2(NVIDIA) datasets ReaxFF/c, Tersoff, Leonard-Jones, SNAP | NAMD 3.0alpha9 dataset STMV_NVE | OpenMM 7.7.0 Ensemble runs for datasets: amber20-stmv, amber20-cellulose, apoa1pme, pme|
GROMACS 2021.1(AMD) 2022(NVIDIA) datasets ADH-Dodec (h-bond), STMV (h-bond) | AMBER 20.xx_rocm_mr_202108(AMD) and 20.12-AT_21.12 (NVIDIA) datasets Cellulose_NVE, STMV_NVE | 1x MI250 has 2x GCD
The excellent performance and power efficiency of the NVIDIA A100 GPU is the result of many years of relentless software-hardware co-optimization to maximize application performance and efficiency. For more information about the NVIDIA Ampere architecture, see the NVIDIA A100 Tensor Core GPU whitepaper.
A100 also presents as a single processor to the operating system, requiring that only one MPI rank be launched to take full advantage of its performance. And, A100 delivers excellent performance at scale thanks to the 600-GB/s NVLink connections between all GPUs in a node.
AI and HPC convergence
Just as accelerated computing is bringing many-fold speedups to modeling and simulation applications, the combination of AI and HPC will deliver the next step-function increase in performance to unlock the next wave of scientific discovery.
In the three years between our first MLPerf training submissions and the most recent results, the NVIDIA platform has delivered 20x more deep learning training performance on this industry-standard, peer-reviewed suite of benchmarks. The gains come from a combination of chip, software, and at-scale improvements.
Scientists and researchers are already using the power of AI to deliver dramatic improvements in performance, turbocharging scientific discovery:
- Enabling a 105 reduction in the time required for identifying gravitational waves.
- Providing a 1,000x speed-up for simulating the Delta SARS-CoV-2 virus in a respiratory droplet with more than a billion atoms.
- Accelerating the development of clean fusion energy.
- Creating predictive digital twins for heat recovery steam generator (HRSG) plants.
Supercomputing centers around the world are continuing to adopt accelerated AI supercomputers.
- The Polaris supercomputer at the Argonne Leadership Computing Facility (ALCF), Perlmutter at NERSC, and Leonardo at CINECA are all powered by A100 Tensor Core GPUs.
- The upcoming Alps supercomputer based on our upcoming Grace Hopper Superchip will come online in 2023.
- The upcoming Venado system at Los Alamos National Laboratory, scheduled for delivery in 2023, will include both the Grace Hopper Superchip as well as Grace CPU Superchip nodes.
For more information about the latest performance data, see HPC Application Performance.
The home-buying process can feel like an obstacle course — finding the perfect place, putting together an offer and, the biggest hurdle of all, securing a mortgage. San Francisco-based real-estate technology company Doma is helping prospective homeowners clear that hurdle more quickly with the support of AI. Its machine learning models accelerate properties through the Read article >
The post The Closer: Machine Learning Helps Banks, Buyers Finalize Real Estate Transactions appeared first on NVIDIA Blog.
Learn more about new features and ways to improve system performance using Nsight Compute 2022.2
NVIDIA Nsight Compute is an interactive kernel profiler for CUDA applications. It provides detailed performance metrics and API debugging through a user interface and a command-line tool. Nsight Compute 2022.2 includes features to expand the supported environments and workflows for CUDA kernel profiling and optimization.
The following outlines the feature highlights of Nsight Compute 2022.2.
NVIDIA OptiX acceleration structure viewer
With the new NVIDIA OptiX acceleration structure viewer, users can inspect the structures they build before launching a ray-tracing pipeline. Acceleration structures describe a rendered scene’s geometries for ray-tracing intersection calculations. Users create these acceleration structures and OptiX translates them to internal data structures. Sometimes the description created by the user is error prone and it can be difficult to understand why the rendered result is not as expected or what is limiting performance.
With this new feature, users can navigate through them in a 3D visualizer and view the parameters used during their creation like build flags, triangle mesh vertices, and AABB coordinates. This viewer is useful to identify overlaps or inefficient hierarchies, resulting in subpar ray-tracing performance.
![Nsight Compute Acceleration Structure Viewer provides 3D Scene Navigation and metrics]](https://developer-blogs.nvidia.com/wp-content/uploads/2022/05/image3-3-625x379.png)
Issues detection per kernel
The latest version adds a new “Issues Detected” column to the summary page for users to sort all profiled kernels by the number of performance issues detected. This gives users guidance on where to focus their efforts across multiple results (kernel profiles). If users are unsure which kernel to focus their optimization efforts on, a long running kernel with a high number of detected issues is a good starting point.

Additional improvements
There are improvements to the metric grouping and selection options on the source page to make them easier to use. Additionally, this release adds support for running the Nsight Compute user interface on ARM SBSA and L4T based platforms, for users to profile without needing remote connections or separate host machines for the user interface.
Check out the sessions below released at NVIDIA GTC 2022 showcasing Nsight tool capabilities, support with Jetson Orin, and more.
- How To Understand and Optimize Shared Memory Accesses using Nsight Compute
- What, Where, and Why? – Use CUDA Developer Tools to Detect, Locate, and Explain Bugs and Bottlenecks
- Orin Developer Tools: The Next Frontier
Nsight Compute Resources
- Learn more and download
- Documentation
- Developer forums
- Additional videos and blog posts