AWS and NVIDIA have collaborated to develop an online course that introduces Amazon SageMaker with EC2 Instances powered by NVIDIA GPUs.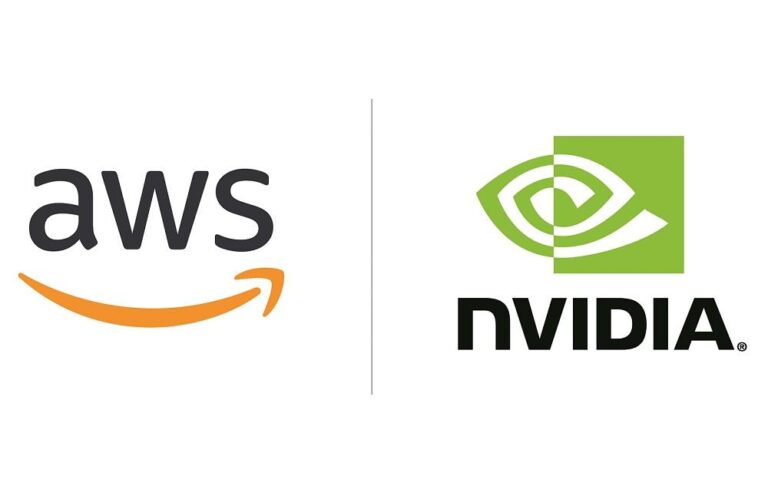
AWS and NVIDIA have collaborated to develop an online course that guides you through a simple-to-follow and practical introduction to Amazon SageMaker with EC2 Instances powered by NVIDIA GPUs. This course is grounded in the practical application of services and gives you the opportunity to learn hands-on from experts in machine learning development. Through a simple and straightforward approach, once completed, you will have the confidence and competency to immediately begin working on your ML project.
Machine learning can be complex, tedious, and time-consuming. AWS and NVIDIA provide the fastest, most effective, and easy-to-use ML tools to get you started on your ML project. Amazon SageMaker helps data scientists and developers prepare, build, train, and deploy high-quality ML models quickly by bringing together a broad set of capabilities purpose-built for ML. Amazon EC2 instances powered by NVIDIA GPUs along with NVIDIA software offer high-performance, GPU-optimized instances in the cloud for efficient model training and cost-effective model inference hosting.
In this course, you will first be given a high-level overview of modern machine learning. Then, we will dive right in and get you up and running with a GPU-powered SageMaker instance. You will learn how to prepare a dataset for training a model, how to build a model, how to execute the training of a model, and how to deploy and optimize a model. You will learn hands-on how to apply this workflow for computer vision (CV) and natural language processing (NLP) use cases.
After completing this course, you will be able to build, train, deploy, and optimize ML workflows with GPU acceleration in Amazon SageMaker and understand the key SageMaker services applicable to tabular, computer vision, and language ML tasks. You will feel empowered and have the confidence and competency to solve complex machine learning problems in a more efficient manner. By using SageMaker, you will simplify workflows so you can build and deploy ML models quickly, freeing you up to focus on other problems to solve.
Course Overview
This course is designed for machine learning practitioners, including data scientists and developers, who have a working knowledge of machine learning workflows. In this course, you will gain hands-on experience with Amazon SageMaker and Amazon EC2 instances powered by NVIDIA GPUs. There are four modules in the course:
Module 1 – Introduction to Amazon SageMaker and NVIDIA GPUs
In this module, you will learn about the purpose-built tools available within Amazon SageMaker for modern machine learning. This includes a tour of the Amazon SageMaker Studio IDE that can be used to prepare, build, train and tune, and deploy and manage your own ML models. Then you will learn how to use Amazon SageMaker classic notebooks and Amazon SageMaker Studio notebooks to develop natural language processing (NLP), computer vision (CV), and other ML models using RAPIDS. You will also dive deep into NVIDIA GPUs, the NGC Catalog, and instances available on AWS for ML.
Module 2 – GPU Accelerated Machine Learning Workflows with RAPIDS and Amazon SageMaker
In this module, you will apply your knowledge of NVIDIA GPUs and Amazon SageMaker. You will gain a background in GPU accelerated machine learning and perform the steps required to set up Amazon SageMaker. You will then learn about data acquisition and data transformation, move on to model design and training, and finish up by evaluating hyperparameter optimization, AutoML, and GPU accelerated inferencing.
Module 3 – Computer Vision
In this module, you will learn about the application of deep learning for computer vision (CV). As humans, half of our brains are devoted to visual processing, making it critical to how we perceive the world. Endowing machines with sight has been a challenging endeavor, but advancements in compute, algorithms, and data quality have made computer vision more accessible than ever before. From mobile cameras to industrial mechanic lenses, biological labs to hospital imaging, and self-driving cars to security cameras, data in pixel format is one of the most valuable types of data for consumers and companies. In this module, you will explore common CV applications, and you will learn how to build an end-to-end object detection model on Amazon SageMaker using NVIDIA GPUs.
Module 4 – Natural Language Processing
In this module, you will learn about applying deep learning technologies to the problem of language understanding. What does it mean to understand languages? What is language modeling? What is the BERT language model, and why are such language models used in many popular services like search, office productivity software, and voice agents? Are NVIDIA GPUs a fast and cost-efficient platform to train and deploy NLP Models? In this module, you will find answers to all those questions and more. Whether you are an experienced ML engineer considering implementation or a developer wanting to learn to deploy a language understanding model like BERT quickly, this module is for you.
Conclusion
AWS and NVIDIA provide fast, effective, easy-to-use ML tools to get you started on working on your ML project. Learn more about the course to guide you through your ML journey!