The recently released Cumulus Linux 4.4. In CL 4.4 provides innovation, advanced features and scale enhancements based on the guiding principles of simplicity.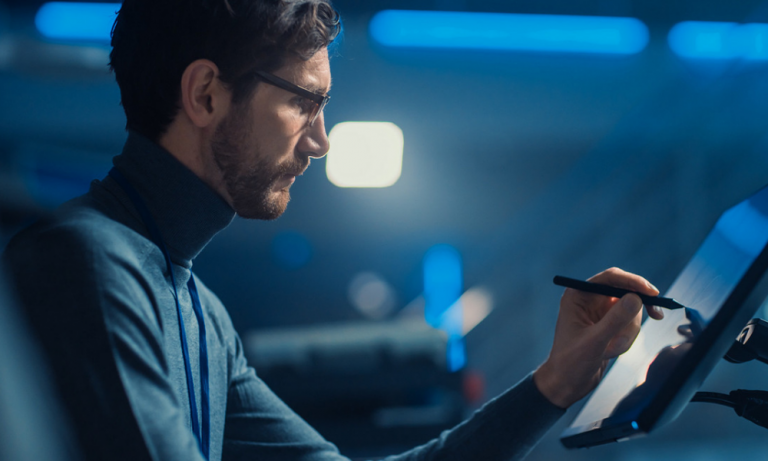
NVIDIA Cumulus Linux is the industry’s most innovative open network operating system that allows you to automate, customize, and scale your data center network like no other.
The recently released Cumulus Linux 4.4. In CL 4.4 provides innovation, advanced features and scale enhancements based on the guiding principles of simplicity.
CL 4.4 includes the following notable new features:
- NVUE (NVIDIA User Experience)
- No license required for users on version 4.4 and forward
- Scale enhancements
- VxLAN EVPN and Multihoming enhancements
- ISSU – Warm boot
- QoS and buffer management enhancements
- PTP enhancements
For these features and all other features, please refer to the CL 4.4 User Guide for details.
NVUE – NVIDIA User Experience
The new release introduces NVUE. NVUE is a brand-new management infrastructure in Cumulus Linux. It was created with the intention to create a better, more efficient, and standard way to manage and automate your network.
This API-driven infrastructure defines an object model for every element in the NOS, and exposes a brand new CLI and a REST API. This new infrastructure enables adding more clients, and with all the API clients having the interface to the same models, it is our first step towards the support of standard YANG models and other programmable APIs in the future.
Cumulus 4.4 provides you the option to explore and use NVUE. Please note that in 4.4 the legacy NCLU command is still available as the default CLI, and you can decide which CLI you would like to enable manually.
*NCLU and NVUE do not interoperate. For further clarification, please refer to the Cumulus Linux 4.4 User Guide.
No License required
From CL 4.4 there is no need anymore to install a license key, you turn up the system and it’s up and running, simple as that.
Scale enhancements
Multiple VLAN-aware bridges mean that you can have overlapping and unique VLANs across bridges. Additionally, multiple VNIs (VxLAN tunnels) can now be mapped to a single Linux “device”.
These new capabilities allow for thousands of tunnels: An order of magnitude more than the previous release! We also increased the number of allowed VLANs and the number of IPv4 LPMs, and our plan is to continue and increase the scale in different vectors, so follow us up on the next release.
VxLAN EVPN and Multihoming enhancements
Multihoming is the modern way to design your high availability in your VxLAN EVPN environment. It simplifies the network, removes the need for MLAG, and the need for dedicated peer links.
In this version we are adding HERP (Head End Replication) support for Multihoming. HREP removes the need for multicast. We also added support for SVI-IP reuse across racks for scalability.
In addition to the multihoming enhancements, we have added support for Downstream VNIs, enabling additional VxLAN EVPN use cases.
ISSU – Warm boot
Cumulus 4.4 offers a variety of design and technology options for a safe upgrade progress with minimal impact, spending less “keeping the lights on” and more time innovating.
In particular, ISSU (In service Software update) allows software updates throughout a process that assures no or limited hit on the traffic.
In this release we are also introducing the warm-boot option. This provides the ability to restart and upgrade the operating system in a single switch with no traffic impact. This is in addition to the fast-boot option which we already support that allows software updates with a very short downtime.
QoS and buffer management enhancements
Our Spectrum systems based on the Spectrum series ASICs offer a full shared buffer for complete fairness in your datacenter network. Complementing this, we now support improved default behavior with the default dynamic buffer configuration. We also added support for ETS (Enhanced Transmission Selection).
PTP enhancements (as Early Access)
In Cumulus 4.4 we present some impressive new features we’ve added to our PTP feature set. Here is a partial list of the PTP new features:
- PTP Boundary Clock: Spectrum2 and Spectrum3 based platforms
- PTP Support for VLAN, LAG (per port), L2 (per VLAN), L3, VRF & IPv6
- User configurable TTL and DSCP values
- PTPv1 message forwarding
As this is only a partial list of our new features, please refer to the Cumulus Linux datasheet for more details.
Finally, NVIDIA AIR is also updated with Cumulus 4.4. Create your first networking digital twin simulation, and check out and upgrade your environment to take advantage of all the new capabilities!To learn more, visit the NVIDIA ethernet switching solutions webpage.