submitted by /u/uvcrtok
[visit reddit] [comments]
As I asked here on StackOverflow, I’m having problems building a model with strings as input since the input layer is a tf.keras.Input(shape=(1,), dtype=tf.string, name=’text’) but the BERT tokenizer expects a string. How do you extract the input string from the keras input?
submitted by /u/childintime9
[visit reddit] [comments]
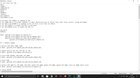
Hello, I’ve just started learning and messing around with neural networks. I’m not sure if this is a problem, or this is how neural networks work, but I’ve noticed, that whenever I try to predict a binary classification outcome with my model, the predictions vary completely based on the size of the data i pass it.
For example, if I try to predict a single outcome with one row of data, I get something like 0.4. Then if I add another row of data and predict again, the first prediction of row 1 becomes 0.9, even though the data in row 1 did not change, I only added an additional row of data for an additional prediction.
My training data consists of 1266 entries with 54 features. I’ve tried reducing the batch_size to 1, different optimizers, number of layers, number of neurons and the result is mostly the same. Is this normal behavior?
submitted by /u/CandyPoper
[visit reddit] [comments]
Idea generation, not hardware or software, needs to be the bottleneck to the advancement of AI, Bryan Catanzaro, vice president of applied deep learning research at NVIDIA, said this week at the AI Hardware Summit. “We want the inventors, the researchers and the engineers that are coming up with future AI to be limited only Read article >
The post Pushing Forward the Frontiers of Natural Language Processing appeared first on The Official NVIDIA Blog.
As neural network models and training data size grow, training efficiency is becoming an important focus for deep learning. For example, GPT-3 demonstrates remarkable capability in few-shot learning, but it requires weeks of training with thousands of GPUs, making it difficult to retrain or improve. What if, instead, one could design neural networks that were smaller and faster, yet still more accurate?
In this post, we introduce two families of models for image recognition that leverage neural architecture search, and a principled design methodology based on model capacity and generalization. The first is EfficientNetV2 (accepted at ICML 2021), which consists of convolutional neural networks that aim for fast training speed for relatively small-scale datasets, such as ImageNet1k (with 1.28 million images). The second family is CoAtNet, which are hybrid models that combine convolution and self-attention, with the goal of achieving higher accuracy on large-scale datasets, such as ImageNet21 (with 13 million images) and JFT (with billions of images). Compared to previous results, our models are 4-10x faster while achieving new state-of-the-art 90.88% top-1 accuracy on the well-established ImageNet dataset. We are also releasing the source code and pretrained models on the Google AutoML github.
EfficientNetV2: Smaller Models and Faster Training
EfficientNetV2 is based upon the previous EfficientNet architecture. To improve upon the original, we systematically studied the training speed bottlenecks on modern TPUs/GPUs and found: (1) training with very large image sizes results in higher memory usage and thus is often slower on TPUs/GPUs; (2) the widely used depthwise convolutions are inefficient on TPUs/GPUs, because they exhibit low hardware utilization; and (3) the commonly used uniform compound scaling approach, which scales up every stage of convolutional networks equally, is sub-optimal. To address these issues, we propose both a training-aware neural architecture search (NAS), in which the training speed is included in the optimization goal, and a scaling method that scales different stages in a non-uniform manner.
The training-aware NAS is based on the previous platform-aware NAS, but unlike the original approach, which mostly focuses on inference speed, here we jointly optimize model accuracy, model size, and training speed. We also extend the original search space to include more accelerator-friendly operations, such as FusedMBConv, and simplify the search space by removing unnecessary operations, such as average pooling and max pooling, which are never selected by NAS. The resulting EfficientNetV2 networks achieve improved accuracy over all previous models, while being much faster and up to 6.8x smaller.
To further speed up the training process, we also propose an enhanced method of progressive learning, which gradually changes image size and regularization magnitude during training. Progressive training has been used in image classification, GANs, and language models. This approach focuses on image classification, but unlike previous approaches that often trade accuracy for improved training speed, can slightly improve the accuracy while also significantly reducing training time. The key idea in our improved approach is to adaptively change regularization strength, such as dropout ratio or data augmentation magnitude, according to the image size. For the same network, small image size leads to lower network capacity and thus requires weak regularization; vice versa, a large image size requires stronger regularization to combat overfitting.
![]() |
Progressive learning for EfficientNetV2. Here we mainly focus on three types of regularizations: data augmentation, mixup, and dropout. |
We evaluate the EfficientNetV2 models on ImageNet and a few transfer learning datasets, such as CIFAR-10/100, Flowers, and Cars. On ImageNet, EfficientNetV2 significantly outperforms previous models with about 5–11x faster training speed and up to 6.8x smaller model size, without any drop in accuracy.
![]() |
EfficientNetV2 achieves much better training efficiency than prior models for ImageNet classification. |
CoAtNet: Fast and Accurate Models for Large-Scale Image Recognition
While EfficientNetV2 is still a typical convolutional neural network, recent studies on Vision Transformer (ViT) have shown that attention-based transformer models could perform better than convolutional neural networks on large-scale datasets like JFT-300M. Inspired by this observation, we further expand our study beyond convolutional neural networks with the aim of finding faster and more accurate vision models.
In “CoAtNet: Marrying Convolution and Attention for All Data Sizes”, we systematically study how to combine convolution and self-attention to develop fast and accurate neural networks for large-scale image recognition. Our work is based on an observation that convolution often has better generalization (i.e., the performance gap between training and evaluation) due to its inductive bias, while self-attention tends to have greater capacity (i.e., the ability to fit large-scale training data) thanks to its global receptive field. By combining convolution and self-attention, our hybrid models can achieve both better generalization and greater capacity.
We observe two key insights from our study: (1) depthwise convolution and self-attention can be naturally unified via simple relative attention, and (2) vertically stacking convolution layers and attention layers in a way that considers their capacity and computation required in each stage (resolution) is surprisingly effective in improving generalization, capacity and efficiency. Based on these insights, we have developed a family of hybrid models with both convolution and attention, named CoAtNets (pronounced “coat” nets). The following figure shows the overall CoAtNet network architecture:
![]() |
Overall CoAtNet architecture. Given an input image with size HxW, we first apply convolutions in the first stem stage (S0) and reduce the size to H/2 x W/2. The size continues to reduce with each stage. Ln refers to the number of layers. Then, the early two stages (S1 and S2) mainly adopt MBConv building blocks consisting of depthwise convolution. The later two stages (S3 and S4) mainly adopt Transformer blocks with relative self-attention. Unlike the previous Transformer blocks in ViT, here we use pooling between stages, similar to Funnel Transformer. Finally, we apply a classification head to generate class prediction. |
CoAtNet models consistently outperform ViT models and its variants across a number of datasets, such as ImageNet1K, ImageNet21K, and JFT. When compared to convolutional networks, CoAtNet exhibits comparable performance on a small-scale dataset (ImageNet1K) and achieves substantial gains as the data size increases (e.g. on ImageNet21K and JFT).
We also evaluated CoAtNets on the large-scale JFT dataset. To reach a similar accuracy target, CoAtNet trains about 4x faster than previous ViT models and more importantly, achieves a new state-of-the-art top-1 accuracy on ImageNet of 90.88%.
Conclusion and Future Work
In this post, we introduce two families of neural networks, named EfficientNetV2 and CoAtNet, which achieve state-of-the-art performance on image recognition. All EfficientNetV2 models are open sourced and the pretrained models are also available on the TFhub. CoAtNet models will also be open-sourced soon. We hope these new neural networks can benefit the research community and the industry. In the future we plan to further optimize these models and apply them to new tasks, such as zero-shot learning and self-supervised learning, which often require fast models with high capacity.
Acknowledgements
Special thanks to our co-authors Hanxiao Liu and Quoc Le. We also thank the Google Research, Brain Team and the open source contributors.
This feels like a profoundly stupid question, and maybe that’s why I’m not finding any answers to it… am new to machine learning.
I’m used to doing development inside VMs, but as I want to benefit from the GPU that’s not really an option here, right? I was thinking maybe I could do it in a Docker container instead (am on Windows) but not sure that’s viable, either. Would either a VM or Docker work for Windows and doing ML? Thanks.
submitted by /u/asking4afriend40631
[visit reddit] [comments]
I have an object detection model (Faster R-CNN sved as a frozen graph) that was trained over two years ago. It requires TF GPU 1.14 and the TF Object Detection API. It’s a bit of a hassle to setup that environment and was wondering if there was a more streamlined way to use that model with the latest version of TF/Keras?
submitted by /u/bc_uk
[visit reddit] [comments]
Want to play awesome PC games for free without having to buy an expensive gaming rig? This GFN Thursday takes a look at the 90+ free-to-play PC games — including this week’s Fortnite Season 8 release and the Epic Games Store free game of the week, Speed Brawl, free to claim Sept. 16-23 — all Read article >
The post GeForce NOW Members Are Free to Play a Massive Library of Most-Played Games, Included With Membership appeared first on The Official NVIDIA Blog.
I am on tf_nightly-2.7.0 and used tensorflow’s “make_csv_dataset” to make dataset from a TSV file, but it seems the Tensorflow PrefetchDataset doesn’t have shape information. I could have used Pandas dataframe but would like to try Tensorflow’s dataset. Here are codes without the import:
!wget https://cdn.freecodecamp.org/project-data/sms/train-data.tsv train_file_path = "train-data.tsv" train_data = tf.data.experimental.make_csv_dataset(train_file_path, header=False, field_delim='t', column_names=['label', 'text'], batch_size=5, label_name='label', num_epochs=1, ignore_errors=True) examples, labels = next(iter(train_data)) # Just the first batch. print("FEATURES: n", examples, "n") print("LABELS: n", labels) encoder = keras.layers.TextVectorization(max_tokens=None, output_mode='int', output_sequence_length=160) encoder.adapt(train_data)
Here is how the dataset looks in the print output:
FEATURES: OrderedDict([('text', <tf.Tensor: shape=(5,), dtype=string, numpy= array([b'rt-king pro video club>> need help? info@ringtoneking.co.uk or call 08701237397 you must be 16+ club credits redeemable at www.ringtoneking.co.uk! enjoy!', b'good afternoon sunshine! how dawns that day ? are we refreshed and happy to be alive? do we breathe in the air and smile ? i think of you, my love ... as always', b'they have a thread on the wishlist section of the forums where ppl post nitro requests. start from the last page and collect from the bottom up.', b'no current and food here. i am alone also', b'die... i accidentally deleted e msg i suppose 2 put in e sim archive. haiz... i so sad...'], dtype=object)>)]) LABELS: tf.Tensor([b'spam' b'ham' b'ham' b'ham' b'ham'], shape=(5,), dtype=string)
Here is the error on line encoder.adapt(train_data) :
AttributeError: 'NoneType' object has no attribute 'ndims
The desired outcome would be no error message after manipulating the Tensorflow dataset.
Thank you for the help in advance!
submitted by /u/na_haran
[visit reddit] [comments]