Posted by Candice Schumann and Susanna Ricco, Software Engineers, Google Research
In 2016, we introduced Open Images, a collaborative release of ~9 million images annotated with image labels spanning thousands of object categories and bounding box annotations for 600 classes. Since then, we have made several updates, including the release of crowdsourced data to the Open Images Extended collection to improve diversity of object annotations. While the labels provided with these datasets were expansive, they did not focus on sensitive attributes for people, which are critically important for many machine learning (ML) fairness tasks, such as fairness evaluations and bias mitigation. In fact, finding datasets that include thorough labeling of such sensitive attributes is difficult, particularly in the domain of computer vision.
Today, we introduce the More Inclusive Annotations for People (MIAP) dataset in the Open Images Extended collection. The collection contains more complete bounding box annotations for the person class hierarchy in 100k images containing people. Each annotation is also labeled with fairness-related attributes, including perceived gender presentation and perceived age range. With the increasing focus on reducing unfair bias as part of responsible AI research, we hope these annotations will encourage researchers already leveraging Open Images to incorporate fairness analysis in their research.
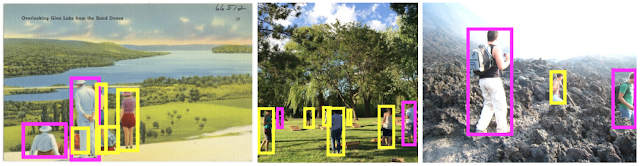 |
Examples of new boxes in MIAP. In each subfigure the magenta boxes are from the original Open Images dataset, while the yellow boxes are additional boxes added by the MIAP Dataset. Original photo credits — left: Boston Public Library; middle: jen robinson; right: Garin Fons; all used with permission under the CC- BY 2.0 license. |
Annotations in Open Images
Each image in the original Open Images dataset contains image-level annotations that broadly describe the image and bounding boxes drawn around specific objects. To avoid drawing multiple boxes around the same object, less specific classes were temporarily pruned from the label candidate set, a process that we refer to as hierarchical de-duplication. For example, an image with labels animal, cat, and washing machine has bounding boxes annotated for cat and washing machine, but not for the redundant class animal.
The MIAP dataset addresses the five classes that are part of the person hierarchy in the original Open Images dataset: person, man, woman, boy, girl. The existence of these labels make the Open Images dataset uniquely valuable for research advancing responsible AI, allowing one to train a general person detector with access to gender- and age-range-specific labels for fairness analysis and bias mitigation.
However, we found that the combination of hierarchical de-duplication and societally imposed distinctions between woman/girl and man/boy introduced limitations in the original annotations. For example, if annotators were asked to draw boxes for the class girl, they would not draw a box around a boy in the image. They may or may not draw a box around a woman depending on their assessment of the age of the individual and their cultural understanding of the concept of girl. These decisions could be applied inconsistently between images, depending on the cultural background of the individual annotator, the appearance of an individual, and the context of the scene. Consequently, the bounding box annotations in some images were incomplete, with some people who appeared prominently not being annotated.
Annotations in MIAP
The new MIAP annotations are designed to address these limitations and fulfill the promise of Open Images as a dataset that will enable new advances in machine learning fairness research. Rather than asking annotators to draw boxes for the most specific class from the hierarchy (e.g., girl), we invert the procedure, always requesting bounding boxes for the gender- and age-agnostic person class. All person boxes are then separately associated with labels for perceived gender presentation (predominantly feminine, predominantly masculine, or unknown) and age presentation (young, middle, older, or unknown). We recognize that gender is not binary and that an individual’s gender identity may not match their perceived or intended gender presentation and, in an effort to mitigate the effects of unconscious bias on the annotations, we reminded annotators that norms around gender expression vary across cultures and have changed over time.
This procedure adds a significant number of boxes that were previously missing.
Over the 100k images that include people, the number of person bounding boxes have increased from ~358k to ~454k. The number of bounding boxes per perceived gender presentation and perceived age presentation increased consistently. These new annotations provide more complete ground truth for training a person detector as well as more accurate subgroup labels for incorporating fairness into computer vision research.
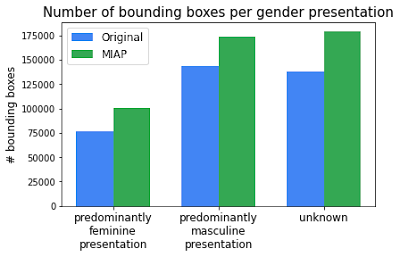 |
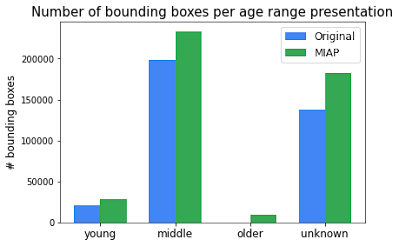 |
Comparison of number of person bounding boxes between the original Open Images and the new MIAP dataset. |
Intended Use
We include annotations for perceived age range and gender presentation for person bounding boxes because we believe these annotations are necessary to advance the ability to better understand and work to mitigate and eliminate unfair bias or disparate performance across protected subgroups within the field of image understanding. We note that the labels capture the gender and age range presentation as assessed by a third party based on visual cues alone, rather than an individual’s self-identified gender or actual age. We do not support or condone building or deploying gender and/or age presentation classifiers trained from these annotations as we believe the risks associated with the use of these technologies outside fairness research outweigh any potential benefits.
Acknowledgements
The core team behind this work included Utsav Prabhu, Vittorio Ferrari, and Caroline Pantofaru. We would also like to thank Alex Hanna, Reena Jana, Alina Kuznetsova, Matteo Malloci, Stefano Pellegrini, Jordi Pont-Tuset, and Mahima Pushkarna, for their contributions to the project.
