This month, we spotlight Lorenzo Baraldi, Assistant Professor at the University of Modena and Reggio Emilia in Italy.
‘Meet the Researcher’ is a monthly series in which we spotlight different researchers in academia who are using NVIDIA technologies to accelerate their work. This month, we spotlight Lorenzo Baraldi, Assistant Professor at the University of Modena and Reggio Emilia in Italy.
Before working as a professor, Baraldi was a research intern at Facebook AI Research. He serves as an Associate Editor of the Pattern Recognition Letters journal and works at the integration of Vision, Language, and Embodied AI.
What are your research areas of focus?
I work within the AimageLab research group on Computer Vision and Deep Learning. I focus mainly on the integration of vision, language, and action. The final goal of our research is to develop agents that can perceive and act in our world while being capable of communicating with humans.
What motivated you to pursue this research area of focus?
Combining the ability to perceive the visual world around us, with that of acting and that of expressing in natural language is something that humans do quite naturally and is one of the keys to human intelligence. In the last few years, we have witnessed tremendous achievements in areas that consider only one of those abilities: Computer Vision, Natural Language Processing, and Robotics. How to combine these abilities, instead, still needs to be understood and is a thrilling field of research.
Tell us about your current research projects.
We are mainly working in three directions: 1 – we integrate vision and language, for example by developing algorithms that can describe images in natural language. A recent paper of this work was presented at CVPR Transformer-based model for image captioning; 2 – we integrate vision and action, by developing agents for autonomous navigation. We are interested in agents moving in indoor and outdoor scenarios, and possibly interacting with people, also in crowded situations; 3 – we integrate all of this with the ability to understand language, for instance by training agents that can move following an instruction or curiosity-driven agents that can describe what they see along their path.
What problems or challenges does your research address?
I think one of the main challenges we need to solve is to find the right way of integrating multi-modal information, which can come from either visual, textual, or motorial perception. In other words, we need to find the right architecture for dealing with this information, that is why a lot of our research involves the design of new architectures. Secondly, most of the approaches we design are generative and sequential: we generate sentences, we generate actions or paths for robots, and so on. Again, how to generate sequences conditioned on multi-modal information is still a challenge.
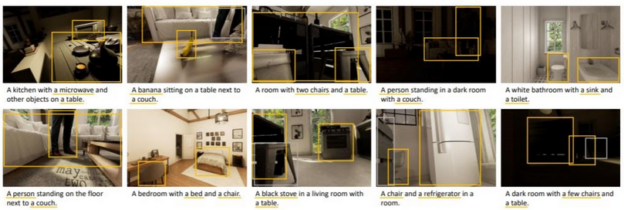
What is the (expected) impact of your work on the field/community/world?
If the research efforts that the community is devoting to this area will be successful, we will have algorithms that can understand us and help us in our daily lives, seeing with us and acting in the world to help us. I think in the long run this might also change the way we interact with computers, which might become a lot easier and language-based.
How have you used NVIDIA technology either in your current or previous research?
Performing large-scale training on NVIDIA GPUs is one of the most important ingredients which power our research, and I am sure this will become even more important in the next future. We do that locally, with a distributed GPU cluster in our lab, and we do that at a bigger scale in conjunction with CINECA, the Italian supercomputing center, and with the NVIDIA AI Technical Centre (NVAITC) of Modena. The partnership we have with NVAITC and CINECA has not only increased our computational capacity, but has also provided us with the knowledge and support we needed to exploit the technologies NVIDIA provides, at their maximum. I would say this collaboration is really having an important impact on our research capabilities.
Did you achieve any breakthroughs in that research or any interesting results using NVIDIA technology?
Most, if not all, of the research works we carry out, are somehow powered by NVIDIA technologies. Apart from the results on the integration of vision, language, and action, we also have a few other research lines of which I am particularly proud. One is related to video understanding: detecting people and objects, understanding their relationships, and finding the best way of extracting Spatio-temporal features is an important challenge. Sometimes we also like to apply our research to the cultural heritage: using NVIDIA GPUs we have developed algorithms for retrieving paintings in natural language, and generative networks for translating artworks to reality.
What is next for your research?
Even though things are evolving rapidly in our area, there are still a lot of key issues that need to be addressed, and that is what our lab concentrating on. One is that going beyond the limitations of traditional supervised learning and fighting dataset bias: in the end, we would like our algorithms to describe and understand any connection between images and text, not just those that are annotated in current datasets. To this end, are working towards algorithms that can describe objects which are not present in the training dataset, and we constantly explore the new possibilities given by self-supervised and weakly-supervised learning. How to properly manage the temporal dimension is also another key issue that has been central in our research, and which has brought advancements in terms of new architectural design, not only for managing sequences of words, but also for understanding video streams.
Any advice for new researchers?
There are at least three capabilities I would recommend pursuing. One is to learn to code well and elegantly because translating ideas to reality is always going to involve implementation. The second is to learn to have good ideas: that is potentially the trickiest part, but it is even more important because every valuable research needs to start from a good idea. I think reading papers, especially from the past, and think openly, freely and on a large-scale is of great help in this sense. The third is time management: always focus on what is impactful.
Baraldi’s colleague, Matteo Tomei, will be presenting their lab’s recent work at NVIDIA GTC in April, “More Efficient and Accurate Video Networks: A New Approach to Maximize the Accuracy/Computation Trade-off”.