![]() |
submitted by /u/Kagermanov [visit reddit] [comments] |
Implementing ONNX models in Rails
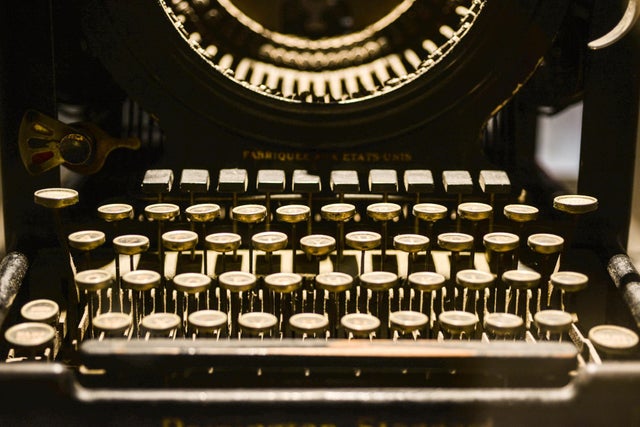
![]() |
submitted by /u/Kagermanov [visit reddit] [comments] |
Imagine walking through the bustling streets of London’s Piccadilly Circus, when suddenly you’re in a tropical rainforest, surrounded by vibrant flowers and dancing butterflies. That’s what audiences will see in the virtual world of The Green Planet AR Experience, an interactive, augmented reality experience that blends physical and digital worlds to connect people with nature. Read article >
The post Beyond Be-leaf: Immersive 3D Experience Transports Audiences to Natural Worlds With Augmented Reality appeared first on NVIDIA Blog.
We present benchmarks and usage of cuTensorNet, a cuQuantum library providing high-performance tensor network computations for quantum circuit simulation.
Quantum computing aspires to deliver more powerful computation in faster time for problems that cannot currently be addressed with classical computing. NVIDIA recently announced the cuQuantum SDK, a high-performance library for accelerating the development of quantum information science. cuQuantum recently was used to break the world record for the MaxCut quantum algorithm simulation running on the DGX SuperPOD, with 8x more qubits than prior work.
The initial target application for cuQuantum is acceleration of quantum circuit simulations, and it consists of two major libraries:
In this post, we provide an overview of both libraries, with a more detailed discussion of cuTensorNet.
The cuStateVec library from the cuQuantum SDK provides a high-performance solution for state vector-based simulation through optimized GPU kernels for most use cases that arise in simulators. While the state vector method is great for running deep quantum circuits, simulations of quantum circuits with large numbers of qubits, which grow exponentially, are impossible to run even on today’s largest supercomputers.
As an alternative, the tensor network method is a technique that represents the quantum state of N qubits as a series of tensor contractions. This enables quantum circuit simulators to handle circuits with many qubits by trading space required by the algorithms with computation. Depending on circuit topology and depth, this can also get prohibitively expensive. Then, the main challenge is to compute these tensor contractions efficiently.
The cuTensorNet library from the cuQuantum SDK provides a high-performance solution for these types of tensor network computations.
The cuTensorNet library offers both C and Python APIs to provide access to high-performance tensor network computations for accelerating quantum circuit simulation. The APIs are flexible, enabling you to control, explore, and investigate each of the algorithmic techniques implemented.
In this section, we discuss the different algorithms and techniques used in cuTensorNet. It includes two main components: pathfinder and execution.
The pathfinder provides an optimal contraction path with minimal cost in a short elapsed time and the execution step computes that path on the GPU using efficient kernels. These two components are independent of each other and are interoperable with any other external library providing similar functionality.
At a high level, the approach taken in cuTensorNet is hyper-optimization around a graph partitioning-based pathfinder. For more information, see Hyper-optimized tensor network contraction.
The role of a pathfinder is to find a contraction path that minimizes the cost of contracting the tensor network. Many algorithmic advancements and optimization were developed to make this step fast, and it will become even faster.
Finding an optimal contraction path is strongly dependent on the size of the network. The larger the network, the more techniques and computational effort are needed to find the optimal contraction path.
The cuTensorNet pathfinder consists of three algorithmic modules (Figure 2).
cutensornetContractionOptimizerConfigSetAttribute
. For more information, see the cuTensorNet documentation.The generated path from the first step might not be close to optimal, so the reconfiguration adjustment is usually performed. Reconfiguration chooses several small subtrees within the overall contraction tree and attempts to improve their contraction cost, decreasing the overall cost if possible.
Another feature of the path computation module is the slicing technique. The primary goal of slicing is to fit the network contraction process into the available device memory. Slicing accomplishes this by excluding certain tensor modes and explicitly unrolling their extents. This generates many similar contraction trees, or slices, where each corresponds to one of the excluded modes.
The contraction path, or tree, does not change. Only some modes are excluded in this case and the computation of each slice is independent from the others. Consequently, slicing can be considered as one of the best techniques to create independent work for different devices.
Practical experience indicates that finding an optimal contraction path can be sensitive to the choice of configuration parameters of each of the techniques used here. To increase the probability of finding the best contraction path, we encapsulate this module inside a hyper-optimizer.
There are two relevant metrics when considering the performance of a pathfinder: the quality of the path found, and the time taken to find that path. The former is plotted in Figure 3, measured by the cost of the resulting contraction in FLOPS. The circuits used for benchmarking are random quantum circuits from Google Quantum AI’s 2019 quantum supremacy paper, at depth 12, 14, and 20.
cuTensorNet performs well compared to the opt_einsum
library in finding an optimal path, and slightly better than Cotengra for these circuits.
cuTensorNet also finds a high-quality path quickly. The time taken to find a contraction for cuTensorNet compared to Cotengra is plotted in Figure 4, for the Sycamore quantum circuits problems with different depth. For the most complex problem with over 3,000 tensors in the network, cuTensorNet still finds its optimal path in just 40 seconds.
The execution component relies on the cuTENSOR library as the backend for efficient execution on the GPU. It consists of the following phases:
Figure 5 measures the speedup of the contraction execution for cuTensorNet compared to CuPy, for several different circuits. Depending on the circuit, cuTensorNet offers around an 8-20x speedup for the contraction execution.
cuTensorNet provides both C and Python APIs that allow you to compute tensor network contractions efficiently without requiring any expertise on how to find the best contraction path or how to execute it on GPUs.
cuTensorNet offers high-level Python APIs that are interoperable with NumPy and CuPy ndarrays and PyTorch tensors. For example, the einsum
expression of a tensor network can be used in a single function call to contract
. cuTensorNet performs all the required steps, returning the contracted network as a result.
import cupy as cp import cuquantum # Compute D_{m,x,n,y} = A_{m,h,k,n} B_{u,k,h} C_{x,u,y} # Create an array of extents (shapes) for each tensor extentA = (96, 64, 64, 96) extentB = (96, 64, 64) extentC = (64, 96, 64) extentD = (96, 64, 96, 64) # Generate input tensor data directly on GPU A_d = cp.random.random(extentA, dtype=cp.float32) B_d = cp.random.random(extentB, dtype=cp.float32) C_d = cp.random.random(extentC, dtype=cp.float32) # Set the pathfinder options options = cuquantum.OptimizerOptions() options.slicing.disable_slicing = 1 # disable slicing options.samples = 100 # number of hyper-optimizer samples # Run the contraction on a CUDA stream stream = cp.cuda.Stream() D_d, info = cuquantum.contract( 'mhkn,ukh,xuy->mxny', A_d, B_d, C_d, optimize=options, stream=stream, return_info=True) stream.synchronize() # Check the optimizer info print(f"{info[1].opt_cost/1e9} GFLOPS")
From this code example, you can see that all cuTensorNet operations are encapsulated in the single contract
API. The output for this example is 14.495514624 GFLOPS: the number of floating-point operations estimated based on the contraction path found by the path finder. To perform the same steps manually, you can also use the cuQuantum.Network object.
As previously discussed, the C and Python APIs are designed in a straightforward expressive fashion. You can call the pathfinder function to get an optimized path, followed by a call to perform the contraction on the GPU using that path.
For advanced users, the cuTensorNet library API is designed to grant access to all algorithmic choices available to enable research in this field. For example, you can control how many hyper-optimizer samples the pathfinder can try to find the best contraction path.
There are dozens of parameters that you can modify or control. These are accessible through the helper functions and allow the simple functionalities API to remain unchanged. You are also allowed to provide your own path. For more information about the lower-level options and examples of how to use them, see cuquantum.Network.
The cuTensorNet library of the NVIDIA cuQuantum SDK aims to accelerate tensor network computation on GPUs. In this post, we showed the speedup over state-of-the-art tensor network libraries on key quantum algorithms.
There is extensive development to improve cuTensorNet and expand it with new algorithmic advancements as well as multi-node, multi-GPU execution.
The cuTensorNet library goal is to provide a useful tool for groundbreaking developments in quantum computing. Have feedback and suggestions on how we can improve the cuQuantum libraries? Send email to cuquantum-feedback@nvidia.com.
For more information, see the following resources:
How do you shorten your AI application’s TTM? Here are 3 methods to eliminate framework complexity and cut your training time in half using TAO Toolkit.
AI applications are powered by models. Deep learning models are built on mathematical algorithms and trained using data and human expertise. These models can accurately predict outcomes based on input data such as images, text, or speech.
Building, training, and optimizing these tasks are both critical and time-intensive. Domain expertise and countless hours of computation are needed to develop production-quality models. This is at odds with how quickly enterprises must operationalize their AI initiatives and reduce their time to market (TTM).
Fortunately, there is a solution: pretrained models. Using transfer learning, a pretrained model is one that has already been trained on representative datasets and fine-tuned with weights and biases. Unlike traditional AI algorithms that require significant time and resources to train, AI solutions built with pretrained models are delivered as fully operational, ready-to-use AI engines for a variety of use cases.
In most cases, an “out-of-box” pretrained model may not fit your use case or deliver the accuracy and performance you need. For these instances, you will have to modify or customize the pretrained model to fit your use-case needs.
So how do you customize a pretrained model without spending too much time and effort? You can use NVIDIA TAO, an AI-model-adaptation framework, to simplify your development workflow. The TAO Toolkit is a CLI- and Jupyter notebook-based solution of NVIDIA TAO that makes it very easy to fine-tune pretrained models with your own data. No AI expertise is required.
The TAO Toolkit is highly extensible and helps you adapt your model to new environments, augment your data, or add new classes.
Below are three examples highlighted in the NVIDIA whitepaper that explores proven methodologies for speeding up your AI workflow process.
When you eliminate AI framework complexity, you can focus on what matters: shortening your AI application’s TTM. The TAO Toolkit makes it incredibly easy for you to train, adapt and optimize pretrained models, without the need for large training datasets and AI expertise.
Visit the Github page to get started with the use cases described in the whitepaper. Learn more about the TAO Toolkit.
Proteins are essential molecules found in all living things. They play a central role in our bodies’ structure and function, and they are also featured in many products that we encounter every day, from medications to household items like laundry detergent. Each protein is a chain of amino acid building blocks, and just as an image may include multiple objects, like a dog and a cat, a protein may also have multiple components, which are called protein domains. Understanding the relationship between a protein’s amino acid sequence — for example, its domains — and its structure or function are long-standing challenges with far-reaching scientific implications.
An example of a protein with known structure, TrpCF from E. coli, for which areas used by a model to predict function are highlighted (green). This protein produces tryptophan, which is an essential part of a person’s diet. |
<!–
An example of a protein with known structure, TrpCF from E. coli, for which areas used by a model to predict function are highlighted (green). This protein produces tryptophan, which is an essential part of a person’s diet. |
–>
Many are familiar with recent advances in computationally predicting protein structure from amino acid sequences, as seen with DeepMind’s AlphaFold. Similarly, the scientific community has a long history of using computational tools to infer protein function directly from sequences. For example, the widely-used protein family database Pfam contains numerous highly-detailed computational annotations that describe a protein domain’s function, e.g., the globin and trypsin families. While existing approaches have been successful at predicting the function of hundreds of millions of proteins, there are still many more with unknown functions — for example, at least one-third of microbial proteins are not reliably annotated. As the volume and diversity of protein sequences in public databases continue to increase rapidly, the challenge of accurately predicting function for highly divergent sequences becomes increasingly pressing.
In “Using Deep Learning to Annotate the Protein Universe”, published in Nature Biotechnology, we describe a machine learning (ML) technique to reliably predict the function of proteins. This approach, which we call ProtENN, has enabled us to add about 6.8 million entries to Pfam’s well-known and trusted set of protein function annotations, about equivalent to the sum of progress over the last decade, which we are releasing as Pfam-N. To encourage further research in this direction, we are releasing the ProtENN model and a distill-like interactive article where researchers can experiment with our techniques. This interactive tool allows the user to enter a sequence and get results for a predicted protein function in real time, in the browser, with no setup required. In this post, we’ll give an overview of this achievement and how we’re making progress toward revealing more of the protein universe.
The Pfam database is a large collection of protein families and their sequences. Our ML model ProtENN helped annotate 6.8 million more protein regions in the database. |
Protein Function Prediction as a Classification Problem
In computer vision, it’s common to first train a model for image classification tasks, like CIFAR-100, before extending it to more specialized tasks, like object detection and localization. Similarly, we develop a protein domain classification model as a first step towards future models for classification of entire protein sequences. We frame the problem as a multi-class classification task in which we predict a single label out of 17,929 classes — all classes contained in the Pfam database — given a protein domain’s sequence of amino acids.
Models that Link Sequence to Function
While there are a number of models currently available for protein domain classification, one drawback of the current state-of-the-art methods is that they are based on the alignment of linear sequences and don’t consider interactions between amino acids in different parts of protein sequences. But proteins don’t just stay as a line of amino acids, they fold in on themselves such that nonadjacent amino acids have strong effects on each other.
Aligning a new query sequence to one or more sequences with known function is a key step of current state-of-the-art methods. This reliance on sequences with known function makes it challenging to predict a new sequence’s function if it is highly dissimilar to any sequence with known function. Furthermore, alignment-based methods are computationally intensive, and applying them to large datasets, such as the metagenomic database MGnify, which contains >1 billion protein sequences, can be cost prohibitive.
To address these challenges, we propose to use dilated convolutional neural networks (CNNs), which should be well-suited to modeling non-local pairwise amino-acid interactions and can be run on modern ML hardware like GPUs. We train 1-dimensional CNNs to predict the classification of protein sequences, which we call ProtCNN, as well as an ensemble of independently trained ProtCNN models, which we call ProtENN. Our goal for using this approach is to add knowledge to the scientific literature by developing a reliable ML approach that complements traditional alignment-based methods. To demonstrate this, we developed a method to accurately measure our method’s accuracy.
Evaluation with Evolution in Mind
Similar to well-known classification problems in other fields, the challenge in protein function prediction is less in developing a completely new model for the task, and more in creating fair training and test sets to ensure that the models will make accurate predictions for unseen data. Because proteins have evolved from shared common ancestors, different proteins often share a substantial fraction of their amino acid sequence. Without proper care, the test set could be dominated by samples that are highly similar to the training data, which could lead to the models performing well by simply “memorizing” the training data, rather than learning to generalize more broadly from it.
We create a test set that requires ProtENN to generalize well on data far from its training set. |
<!–
We create a test set that requires ProtENN to generalize well on data far from its training set. |
–>
To guard against this, it is essential to evaluate model performance using multiple separate setups. For each evaluation, we stratify model accuracy as a function of similarity between each held-out test sequence and the nearest sequence in the train set.
The first evaluation includes a clustered split training and test set, consistent with prior literature. Here, protein sequence samples are clustered by sequence similarity, and entire clusters are placed into either the train or test sets. As a result, every test example is at least 75% different from every training example. Strong performance on this task demonstrates that a model can generalize to make accurate predictions for out-of-distribution data.
For the second evaluation, we use a randomly split training and test set, where we stratify examples based on an estimate of how difficult they will be to classify. These measures of difficulty include: (1) the similarity between a test example and the nearest training example, and (2) the number of training examples from the true class (it is much more difficult to accurately predict function given just a handful of training examples).
To place our work in context, we evaluate the performance of the most widely used baseline models and evaluation setups, with the following baseline models in particular: (1) BLAST, a nearest-neighbor method that uses sequence alignment to measure distance and infer function, and (2) profile hidden Markov models (TPHMM and phmmer). For each of these, we include the stratification of model performance based on sequence alignment similarity mentioned above. We compared these baselines against ProtCNN and the ensemble of CNNs, ProtENN.
We measure each model’s ability to generalize, from the hardest examples (left) to the easiest (right). |
Reproducible and Interpretable Results
We also worked with the Pfam team to test whether our methodological proof of concept could be used to label real-world sequences. We demonstrated that ProtENN learns complementary information to alignment-based methods, and created an ensemble of the two approaches to label more sequences than either method could by itself. We publicly released the results of this effort, Pfam-N, a set of 6.8 million new protein sequence annotations.
After seeing the success of these methods and classification tasks, we inspected these networks to understand whether the embeddings were generally useful. We built a tool that enables users to explore the relation between the model predictions, embeddings, and input sequences, which we have made available through our interactive manuscript, and we found that similar sequences were clustered together in embedding space. Furthermore, the network architecture that we selected, a dilated CNN, allows us to employ previously-discovered interpretability methods like class activation mapping (CAM) and sufficient input subsets (SIS) to identify the sub-sequences responsible for the neural network predictions. With this approach, we find that our network generally focuses on the relevant elements of a sequence to predict its function.
Conclusion and Future Work
We’re excited about the progress we’ve seen by applying ML to the understanding of protein structure and function over the last few years, which has been reflected in contributions from the broader research community, from AlphaFold and CAFA to the multitude of workshops and research presentations devoted to this topic at conferences. As we look to build on this work, we think that continuing to collaborate with scientists across the field who’ve shared their expertise and data, combined with advances in ML will help us further reveal the protein universe.
Acknowledgments
We’d like to thank all of the co-authors of the manuscripts, Maysam Moussalem, Jamie Smith, Eli Bixby, Babak Alipanahi, Shanqing Cai, Cory McLean, Abhinay Ramparasad, Steven Kearnes, Zack Nado, and Tom Small.
The Metropolis Partner Program expanded to include a certification that ensures partner applications can be securely deployed to any location with NVIDIA Fleet Command.
Building production-ready AI applications is hard, especially when starting from scratch. That’s why NVIDIA created Metropolis, a suite of tools to help developers build and bring to market vision AI applications.
Deploying these applications in production, especially outside of the data center, can be just as difficult. For many organizations, determining the best way to deploy an application at customer sites is a task started after customer conversations are underway. The Metropolis Partner Program is addressing this with a certification that ensures partner applications can be securely deployed to any location with NVIDIA Fleet Command.
Fleet Command—a cloud service that centrally connects systems at edge locations—helps organizations securely deploy, manage, and scale AI applications from one dashboard. It’s the best way to orchestrate AI across hundreds or even thousands of devices covering vast physical distances. Now, Metropolis partners can use Fleet Command for free to deploy and scale their applications in production environments.
Deploying an application using Fleet Command gives partners a platform to easily conduct POCs at customer sites. This can be done without building custom tools to get applications operational in unique customer environments. Additionally, after the evaluation is complete, the partner has all of the necessary infrastructure to easily scale an application from an evaluation environment to the entire production environment. Saving time gives partners the freedom to focus all their resources on building valuable AI applications.
Once an application is ready for deployment on Fleet Command, partners have free access to the platform for a year. The platform includes cloud access to servers with the latest NVIDIA GPUs, and NVIDIA experts on AI and optimizations, amounting to over a $100,000 value.
Partners looking to demonstrate the value of their application to customers have access to NVIDIA LaunchPad. LaunchPad provides enterprises immediate, short-term access to all of the features and functionality of Fleet Command including provisioning edge infrastructure, deploying and managing applications, and monitoring their edge fleet. Partners can use Fleet Command on LaunchPad for customers to experience the application in an isolated environment before moving to a full evaluation in a production setting.
Over 50 Metropolis Partner Program members are integrating their applications to be deployed and managed on Fleet Command. Partners include Milestone for AI-enabled video management software, OSARO for efficient pick and place robotics solutions, and IronYun for intelligent video analytics in smart buildings.
“With NVIDIA Fleet Command, we can easily deploy and manage our vision AI apps across the edge infrastructure in minutes rather than days,” said Paul Sun, CEO of IronYun. “Our sales and testing cycles are drastically reduced.”
The Metropolis Partner Program enables AI developers with tools to streamline every stage of the process to acquire and keep new customers. This ranges from application development on Metropolis to demos and evaluations on LaunchPad, to POCs and deployments on Fleet Command.
Get access to Fleet Command and LaunchPad today by applying to join the Metropolis Partner Program. If you’re already a member, reach out to your representative to learn how to get started.
The very thing that makes the internet so useful to so many people — the vast quantity of information that’s out there — can also make going online frustrating. There’s so much available that the sheer volume of choices can be overwhelming. That’s where recommender systems come in, explains NVIDIA AI Podcast host Noah Kravitz. Read article >
The post Podsplainer: What’s a Recommender System? NVIDIA’s Even Oldridge Breaks It Down appeared first on NVIDIA Blog.
Is there a way to convert support vector classifier with rbf kernel to tf model?
I am aware of converting support vector classifier with linear kernel because there exists coef_ where we can find parameters and assign to tf model. Got this idea from how to convert saved model from sklearn into tensorflow/lite.
However _coef wont be there for rbf , so i am not sure on how to convert this model to an tf model.
Any suggestions are highly helpful. Thanks
submitted by /u/Mother-Beyond9493
[visit reddit] [comments]
![]() |
submitted by /u/josh-r-meyer [visit reddit] [comments] |
I have a problem, which I could not solve yet.
I need to create a conda environment with tensorflow=2.6.0, python=3.8 and cudatoolkit>=11 (using ampere GPU)
I cannot use conda default channel. Until now I tried using conda-forge and nvidia channel.
I have not succeeded in creating a conda environment with the above requirements. Anyone got a hint?
The machine got centos with Nvidia driver 495 and cuda 11.5
submitted by /u/alex_bababu
[visit reddit] [comments]