This post shows how the SOTA Visual Transformer model, VOLO, is trained on the NVIDIA DGX SuperPOD. VOLO_D5 model.
Recent work has demonstrated that large transformer models can achieve or advance the SOTA in computer vision tasks such as semantic segmentation and object detection. However, unlike convolutional network models that can do it only with the standard public dataset, it takes a proprietary dataset that is magnitudes larger.
VOLO model architecture
The recent project VOLO (Vision Outlooker) from SEA AI Lab, Singapore showed an efficient and scalable vision transformer mode architecture that greatly closed the gap using only the ImageNet-1K dataset.
VOLO introduces a novel outlook attention and presents a simple and general architecture, termed Vision Outlooker. Unlike self-attention, which focuses on global dependency modeling at a coarse level, the outlook attention efficiently encodes finer-level features and contexts into tokens. This is shown to be critically beneficial to recognition performance but largely ignored by self-attention.
Experiments show that the VOLO achieves 87.1% top-1 accuracy on ImageNet-1K classification, which is the first model exceeding 87% accuracy on this competitive benchmark, without using any extra training data.
In addition, the pretrained VOLO transfers well to downstream tasks, such as semantic segmentation.
Settings | LV-ViT | CaiT | NFNet-F6 | NFNNet-F5 | VOLO-D5 |
Test Resolution | 448×448 | 448×448 | 576×576 | 544×544 | 448×448/512×512 |
Model Size | 140M | 356M | 438M | 377M | 296M |
Computations | 157B | 330B | 377B | 290B | 304B/412B |
Architecture | Vision Transformer | Vision Transformer | Convolutions | Convolutions | VOLO |
Extra Augmentations | Token Labeling | Knowledge Distill | SAM | SAM+augmult | Token Labeling |
ImageNet Top-1 Acc. | 86.4 | 86.5 | 86.5 | 86.8 | 87.0/87.1 |
Though VOLO models demonstrated outstanding computational efficiency, training the SOTA performance model is not trivial.
In this post, we present the techniques and experience that we gained training the VOLO models on the NVIDIA DGX SuperPOD based on the NVIDIA ML software stack and Infiniband clustering technologies.
Training methods
Training VOLO models requires considering training strategy, infrastructure, and configuration planning. In this section, we discuss some of the techniques applied in this solution.
Training strategy
Training the model using the original ImageNet sample quality data all the way and performing a neural network (NN) architecture search at a fine grain makes a more consolidated investigation in theory. However, this requires a large percentage of the computing resources budget.
In the scope of this project, we adopted a coarse-grained training approach that does not visit as many NN architecture possibilities as the fine-grained approach. However, it enables showing EIOFS with less time and a lower resource budget. In this alternative strategy, we first trained the potential neural network candidates using image samples with lower resolution and then performed fine-tuning using high-resolution images.
This approach has been proved to be efficient in earlier work in terms of cutting down the computational cost within marginal model performance lost.
Infrastructure
In practice, we used two types of clusters for this training:
- One for base model pretraining, which is an NVIDIA DGX A100 based DGX POD that consists of 5x NVIDIA DGX A100 systems clustered using the NVIDIA Mellanox HDR Infiniband network.
- One for fine-tuning, which is an NVIDIA DGX SuperPOD that consists of DGX A100 systems with the NVIDIA Mellanox HDR Infiniband network.
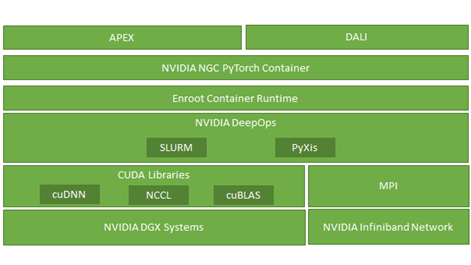
Software infrastructure also played important role in this procedure. Figure 2 shows that, in addition to the underlying standard deep learning optimization CUDA libraries such as cuDNN and cuBLAS, we leveraged NCCL, enroot, PyXis, APEX, and DALI extensively to achieve the sub-linear scalability of the training performance.
The DGX A100 POD cluster is mainly used for base model pretraining using lower size image samples. This is because base model pretraining is less memory-bound and can leverage the compute power advantage of the NVIDIA A100 GPU.
In comparison, the fine-tuning was performed on an NVIDIA DGX SuperPOD of NVIDIA DGX-2 because the fine-tuning process uses bigger images, which requires more memory per compute power.
Training configurations
NEED LEAD-IN SENTENCE
D1 | D2 | D3 | D4 | D5 | |
MLP Ratio | 3 | 3 | 3 | 3 | 4 |
Optimizer | AdamW | ||||
LR Scaling | LR = LRbase x Batch_Size/1024, where LRbase=8.0e-4 | ||||
Weight Decay | 5e-2 | ||||
LRbase | 1.6e-2 | 1e-3 | 1e-3 | 1e-3 | 1e-4 |
Stochastic Depth Rate | 0.1 | 0.2 | 0.5 | 0.5 | 0.75 |
Crop Ratio | 0.96 | 0.96 | 0.96 | 1.15 | 1.15 |
Table 2. Model settings (for all models, the batch size is set to 1024)
We evaluated our proposed VOLO models on the ImageNet dataset. During training, no extra training data was used. Our code was based on PyTorch, the Token Labeling toolbox, and PyTorch Image Models (timm). We used the LV-ViT-S model with Token Labeling as our baseline.
Setup notes
- We used the AdamW optimizer with a linear learning rate scaling strategy LR = LRbase x Batch_Size/1024 and 5 ×10−2 weight decay rate as suggested by previous work, and LRbase are given in Table 3 for all VOLO models.
- Stochastic Depth is used.
- We trained our models on the ImageNet dataset for 300 epochs.
- For data augmentation methods, we used CutOut, RandAug, and the Token Labeling objective with MixToken.
- We did not use MixUp or CutMix as they conflict with MixToken.
Pretraining
In this section, we use VOLO-D5 as an example to demonstrate how the model is trained.
Figure 3 shows that the training throughput for VOLO-D5 using one single DGX A100 is about 500 image/sec. By estimation, it roughly takes about 170 hours to finish one full pretraining cycle, which needs 300 epochs with ImageNet-1K. This is equal to about one week for 1 million images.
To speed up a little bit, based on a simple parameter-server architecture cluster of five DGX A100 nodes, we roughly achieved a 2100 image/sec throughput, which can cut down the pretraining time to ~52 hours.

The VOLO-D5 model pretraining can be started on one single node using the following code example:
CUDA_VISIBLE_DEVICES=0,1,2,3,4,5,6,7 ./distributed_train.sh 8 /path/to/imagenet --model volo_d5 --img-size 224 -b 44 --lr 1.0e-4 --drop-path 0.75 --apex-amp --token-label --token-label-size 14 --token-label-data /path/to/token_label_data
For the MNMG training case, it requires training cluster details as part of the command line input. First, we set CPU, MEM, IB Binding according to the node and cluster architecture. The cluster for the pre-training phase was DGX A100 POD, which has four NUMA domains per CPU socket and 1 IB port per A100 GPU, therefore we bind each rank to all CPU cores in the NUMA node nearest its GPU.
- For memory binding, we bind each rank to the nearest NUMA node.
- For IB binding, we bind one IB card per GPU, or as close to such a setup as possible.
Because the VOLO model training is PyTorch-based, and simply leveraged on the default PyTorch distributed training approach, our multinode, multi-GPU training is based on a simple parameter-server architecture that fits into the fat-tree network topology of NVIDIA DGX SuperPOD.
To simplify the scheduling, the first node in the list of allocated nodes is always used as both parameter server and worker node, and all other nodes are worker nodes. To avoid the potential storage I/O overhead, the dataset, all code, intermediate/milestone checkpoints, and results are kept on a single high-performance DDN-based distributed storage backend. They are mounted to all the worker nodes through a 100G NVIDIA Mellanox EDR Infiniband network.
To accelerate the data preprocessing and pipelining data loading, NVIDIA DALI is configured to use one dedicated data loader per GPU process.

Fine-tuning
Running VOLO-D5 model fine-tuning on one single node is quite straightforward using the following code example:
CUDA_VISIBLE_DEVICES=0,1,2,3,4,5,6,7 ./distributed_train.sh 8 /path/to/imagenet --model volo_d5 --img-size 512 -b 4 --lr 2.3e-5 --drop-path 0.5 --apex-amp --epochs 30 --weight-decay 1.0e-8 --warmup-epochs 5 --ground-truth --token-label --token-label-size 24 --token-label-data /path/to/token_label_data --finetune /path/to/pretrained_224_volo_d5/
As we mentioned earlier, because the image size for fine-tuning is much larger than the one used in the pretraining phase, the batch size must be cut down accordingly. Get the workload to fit into the GPU memory, which makes further scaling out the training to larger numbers of GPUs in parallel mandatory.
Most of the fine-tuning configurations are similar to the pretraining phase.
Conclusion
In this post, we showed the main techniques and procedures for training the SOTA large-scale Visual Transformer models, such as VOLO_D5, on a large-scale AI supercomputer, such as NVIDIA DGX A100 based DGX SuperPOD. The trained VOLO_D5 model achieved the best Top-1 accuracy in the image classification model ranking without using any additional data beyond the ImageNet-1k dataset.
The code resource of this work including the Docker image for running the experiment and the Slurm scheduler script is open source in the sail-sg/volo GitHub repo to allow future work to be leveraged on VOLO_D5 for more extensive study. For more information, see VOLO: Vision Outlooker for Visual Recognition.
In the future, we are looking to scale this work further towards training more intelligent, self-supervised, larger-scale models with larger public datasets and more modern infrastructure, for example, NVIDIA DGX SuperPOD with NVIDIA H100 GPUs.