The NVIDIA Deep Learning Institute (DLI) is offering instructor-led, hands-on training on how to implement multiple AI-based approaches to solve a specific use case of identifying network intrusions for telecommunications.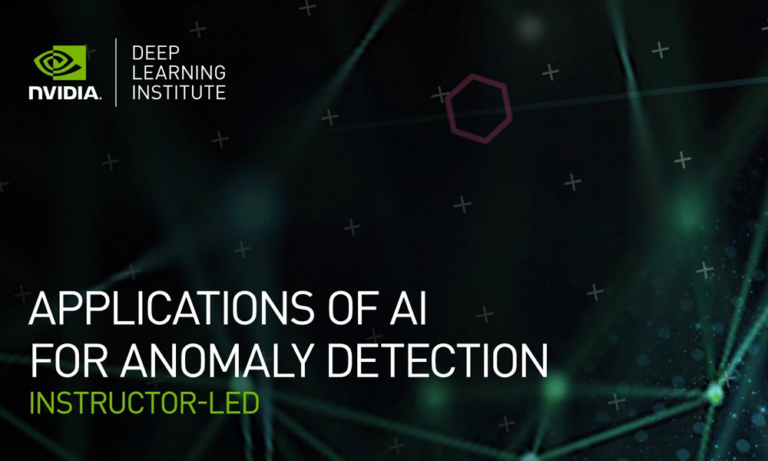
Whether you need to monitor cybersecurity threats, fraudulent financial transactions, product defects, or equipment health, artificial intelligence can help you catch data abnormalities before they impact your business. AI models can be trained and deployed to automatically analyze datasets, define “normal behavior,” and identify breaches in patterns quickly and effectively. These models can then be used to predict future anomalies. With massive amounts of data available across industries and subtle distinctions between normal and abnormal patterns, it’s critical that organizations use AI to quickly detect anomalies that pose a threat.
The NVIDIA Deep Learning Institute (DLI) is offering instructor-led, hands-on training on how to implement multiple AI-based approaches to solve a specific use case of identifying network intrusions for telecommunications. You’ll learn three different anomaly detection techniques using GPU-accelerated XGBoost, deep learning-based autoencoders, and generative adversarial networks (GANs) and then implement and compare supervised and unsupervised learning techniques. At the end of the workshop, you’ll be able to use AI to detect anomalies in your work across telecommunications, cybersecurity, finance, manufacturing, and other key industries.
By participating in this workshop, you’ll:
- Prepare data and build, train, and evaluate models using XGBoost, autoencoders, and GANs
- Detect anomalies in datasets with both labeled and unlabeled data
- Classify anomalies into multiple categories regardless of whether the original data was labeled
This training will be offered:
Tue, Sep 21, 2021, 9:00 a.m. – 5:00 p.m. CEST/EMEA, UTC+2
Tue, Sep 21, 2021, 9:00 a.m. – 5:00 p.m. PDT, UTC-7
Space is limited, register now.