SANTA CLARA, Calif., April 12, 2021 — GTC — Volvo, Zoox and SAIC are among the growing ranks of leading transportation companies using the newest NVIDIA DRIVE™ solutions to power their…
Enterprises rely on GPU virtualization to keep their workforces productive, wherever they work. And NVIDIA virtual GPU (vGPU) performance has become essential to powering a wide range of graphics- and compute-intensive workloads from the cloud and data center. Now, designers, engineers and knowledge workers across industries can experience accelerated performance with the NVIDIA A10 and Read article >
The post NVIDIA Brings Powerful Virtualization Performance with NVIDIA A10 and A16 appeared first on The Official NVIDIA Blog.
SANTA CLARA, Calif., April 12, 2021 — GTC — NVIDIA today announced a range of eight new NVIDIA Ampere architecture GPUs for next-generation laptops, desktops and servers that make it possible…
It’s time to hail the new era of transportation. During his keynote at the GPU Technology Conference today, NVIDIA founder and CEO Jensen Huang outlined the broad ecosystem of companies developing next-generation robotaxis on NVIDIA DRIVE. These forward-looking manufacturers are set to transform the way we move with safer, more efficient vehicles for everyday mobility. Read article >
The post Top Robotaxi Companies Hail Rides on NVIDIA DRIVE appeared first on The Official NVIDIA Blog.
Kicking off NVIDIA’s GTC tech conference, NVIDIA CEO Jensen Huang weaves latest advancements in AI, automotive, robotics, 5G, real-time graphics, collaboration and data centers into a stunning vision of the future.
The post NVIDIA CEO Introduces Software, Silicon, Supercomputers ‘for the Da Vincis of Our Time’ appeared first on The Official NVIDIA Blog.
The electric vehicle revolution is about to reach the next level. Leading startups and EV brands have all announced plans to deliver intelligent vehicles to the mass market beginning in 2022. And these new, clean-energy fleets will achieve AI capabilities for greater safety and efficiency with the high-performance compute of NVIDIA DRIVE. The car industry Read article >
The post New Energy Vehicles Power Up with NVIDIA DRIVE appeared first on The Official NVIDIA Blog.
SANTA CLARA, Calif., April 12, 2021 (GLOBE NEWSWIRE) — NVIDIA today announced at its annual Investor Day that first quarter revenue for fiscal 2022 is tracking above its previously provided …
Data plays a crucial role in creating intelligent applications. To create an efficient AI/ ML app, you must train machine learning models with high-quality, labeled datasets. Generating and labeling such data from scratch has been a critical bottleneck for enterprises. Many companies prefer a one-stop solution to support their AI/ML workflow from data generation, data … Continued
Data plays a crucial role in creating intelligent applications. To create an efficient AI/ ML app, you must train machine learning models with high-quality, labeled datasets. Generating and labeling such data from scratch has been a critical bottleneck for enterprises. Many companies prefer a one-stop solution to support their AI/ML workflow from data generation, data labeling, model training/fine-tuning, and deployment.
To fast track the end-to-end workflow for developers, NVIDIA has been working with several partners who focus on generating large, diverse, and high-quality labeled data. Their platforms can be seamlessly integrated with NVIDIA Transfer Learning Toolkit (TLT) and NeMo for training and fine-tuning models. These efficiently trained and optimized models can then be deployed with NVIDIA DeepStream or NVIDIA Jarvis to create reliable computer vision or conversational AI applications.
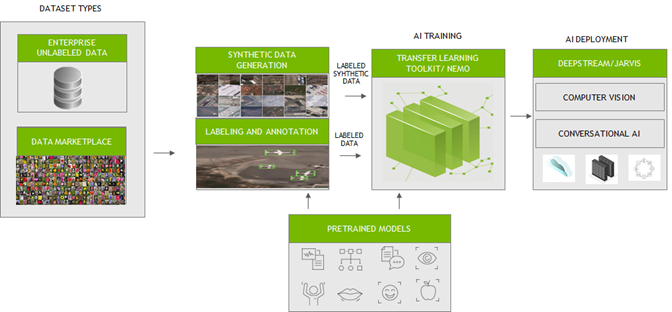
In this post, we outline the key challenges in data preparation and training. We also introduce how to integrate your data to fine-tune AI/ML models easily with our partner services.
Computer vision
Large amounts of labeled data are needed to train computer vision neural network models. They could be collected from the real world or synthesized through models. High-quality labeled data enables neural network models to contextualize the information and generate accurate results.
NVIDIA integrated with the platforms from the following partners to generate synthetic and label custom data in the formats compatible with TLT for training. TLT is a zero-coding transfer learning toolkit using purpose-built, pretrained models. Trained TLT models can be deployed using DeepStream SDK and achieve 10x speedup in development time.
AI Reverie and Sky Engine
Synthetic labeled data is becoming popular especially for computer vision tasks like object detection and image segmentation. Using the platforms from AI Reverie and Sky Engine, you can generate synthetic labeled data. AI Reverie offers a suite of synthetic data for model training and validation using 3D environments which exposes the neural network models to diverse scenarios that might be hard to find in data gleaned from the real world. Sky Engine uses ray-tracing image renderer techniques to generate labeled synthetic data in virtual environments that can be used with TLT for training.
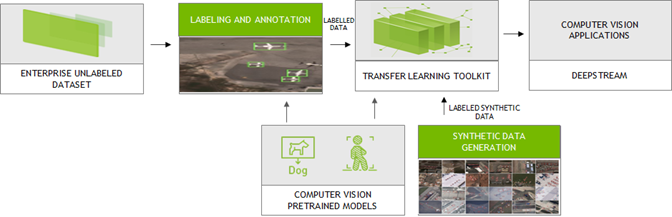
Appen
With Appen, you can generate and label custom data. Appen uses APIs and human intelligence to generate labeled training data. Integrated with the NVIDIA Transfer Learning Toolkit, the Appen Data Annotation platform and services allow you to eliminate time-consuming annotations and create the right training data to train with TLT for your use cases.
Hasty, Labelbox, and Sama
Few provide straightforward tools for annotation. By simple clicks and selecting the region around the objects on the image, you can quickly generate the annotations. With Hasty, Sama, and Labelbox, you can label datasets in the formats compatible with TLT. TLT is a zero-coding transfer learning toolkit using purpose-built, pretrained models. Trained TLT models can be deployed using the DeepStream SDK and achieve 10x speedup in development time.
Hasty provides such tools like DEXTR and GrabCut to create labeled data. With DEXTR, you click on the north, south, east, and west of an object and a neural network looks for the mask. For GrabCut, you select the area where the object is and add or remove regions with markings to improve the results.
With Labelbox, you can upload the data for annotation and can easily export the annotated data using Python SDK to TLT for training purposes.
Sama follows a different labeling mechanism and therefore uses a pretrained object detection model (Figure 2) to perform inference. IT uses those annotations to further enhance the labels in generating accurate results.
All these tools are tightly coupled with TLT for training to develop production-quality vision models.
Conversational AI
A typical conversational AI application may be built with automatic speech recognition (ASR), natural language understanding (NLU), dialog management (DM) and text-to-speech (TTS) services. Conversational AI models are huge and require large amounts of data for training to recognize speech in noisy environments in different languages or accents, understand every nuance of language and generate human-like speech.
NVIDIA collaborates with DefinedCrowd, LabelStudio, and DataSaur. You can use their platforms to create large and labeled datasets that can then be directly introduced into NVIDIA NeMo for training or fine-tuning. NVIDIA NeMo is an open-source toolkit for developing state-of-the-art conversational AI models.
DefinedCrowd
The popular approach for data generation/labeling is crowdsourcing. DefinedCrowd leverages a global crowd of over 500,000 contributors to collect, annotate, and validate training data by dividing the project into a series of micro-tasks through the online platform Neevo. The data is then used to train conversational AI models in a variety of languages, accents, and domains. You can now quickly download the data using the DefinedCrowd APIs and convert them into NeMo acceptable format for training.
LabelStudio
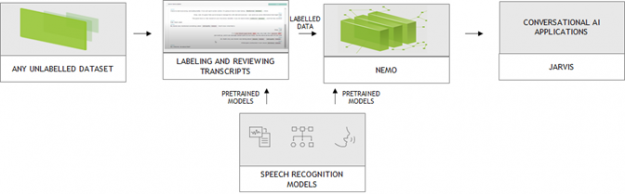
Advancement of machine learning models make AI-assisted data annotation possible, which significantly reduces labeling cost. More and more vendors are integrating AI capability to augment the labeling process. NVIDIA collaborated with Heartex who maintains the open-source LabelStudio and performs AI-assisted data annotation for speech. With this tool, you can now label speech data that is compatible with NeMo (Figure 3). Heartex also integrates NeMo into their workflow so that you can choose the pretrained NeMo models for speech pre-annotation.
Datasaur
For text labeling, there are easy-to-use tools that come with predefined labels which allow you to quickly annotate the data. For more information about how to annotate your text data and run Datasaur with NeMo for training, see Datasaur x NVIDIA NeMo Integration (video).
Conclusion
NVIDIA has partnered with many industry-leading data generation and annotation providers to speed up computer vision and conversational AI application workflows. This collaboration enables you to quickly generate superior quality data that can be readily used with TLT and NeMo for training models, which can then be deployed on NVIDIA GPUs with DeepStream SDK and Jarvis.
For more information, see the following resources:
NVIDIA HPC SDK 21.3 Now Available
The NVIDIA HPC SDK is a comprehensive suite of compilers, libraries, and tools enabling developers to program the entire HPC platform from the GPU foundation to the CPU, and through the interconnect.
Today, NVIDIA is announcing the availability of the HPC SDK version 21.3. This software can be downloaded now free of charge.
What’s New
- HPC-X toolkit, a comprehensive data communications package including MPI
- C++ stdpar support for multicore CPUs
- CUDA 11.2 Update 1
See the HPC SDK Release Notes for more information.
About the NVIDIA HPC SDK
The NVIDIA HPC SDK is a comprehensive suite of compilers, libraries, and tools enabling developers to program the entire HPC platform from the GPU foundation to the CPU, and through the interconnect. It is the only comprehensive, integrated SDK for programming accelerated computing systems.
The NVIDIA HPC SDK C++ and Fortran compilers are the first compilers to support automatic GPU acceleration of standard language constructs including C++17 parallel algorithms and Fortran intrinsics.
Learn more:
- GTC 2021: S31286 A Deep Dive into the Latest HPC Software — GTC is Free, Register Now
- GTC 2021: S31358 Inside NVC++
- NVIDIA HPC SDK Page
- NVIDIA HPC Compilers Page
- CUDA-X GPU-Accelerated Libraries Page
- Recent Developer Blog posts:
- 6 Ways to SAXPY
- Accelerating Standard C++ with GPUs Using stdpar
- Accelerating Fortran DO CONCURRENT with GPUs and the NVIDIA HPC SDK
- Bringing Tensor Cores to Standard Fortran
- Building and Deploying HPC Applications Using NVIDIA HPC SDK from the NVIDIA NGC Catalog
- Accelerating Python on GPUs with NVC++ and Cython
- HPC SDK Product Documentation
Designing rich content and graphics for VR experiences means creating complex materials and high-resolution textures. But rendering all that content at VR resolutions and frame rates can be challenging, especially when rendering at the highest quality. You can address this challenge by using variable rate shading (VRS) to focus shader resources on certain parts of … Continued
Designing rich content and graphics for VR experiences means creating complex materials and high-resolution textures. But rendering all that content at VR resolutions and frame rates can be challenging, especially when rendering at the highest quality.
You can address this challenge by using variable rate shading (VRS) to focus shader resources on certain parts of an image—specifically, the parts that have the greatest impact for the scene, such as where the user is looking or the materials that need higher sampling rates.
However, because not all developers could integrate the full NVIDIA VRS API, NVIDIA developed Variable Rate Supersampling (VRSS), which increases the image quality in the center of the screen (fixed foveated rendering). VRSS is a zero-coding solution, so you do not need to add code to implement it. All the work is done through NVIDIA drivers, which makes it easy for users to experience VRSS in games and applications.

NVIDIA is now releasing VRSS 2, which now includes gaze-tracked, foveated rendering. The latest version further improves the perceived image quality by supersampling the region of the image where the user is looking. Applications that benefited from the original VRSS look even better with dynamic foveated rendering in VRSS 2.
New VR features
NVIDIA and Tobii collaborated to enhance VRSS with dynamic foveated rendering enabled by Tobii Spotlight, an eye-tracking technology specialized for foveation. This technology powers the NVIDIA driver with the latest eye-tracking information at minimal latency, which is used to control the supersampled region of the render frame. HPI’s upcoming Omnicept G2 HMD will be the first HMD on the market that takes advantage of this integration, as it uses both Tobii’s gaze-tracking technology and NVIDIA VRSS 2.
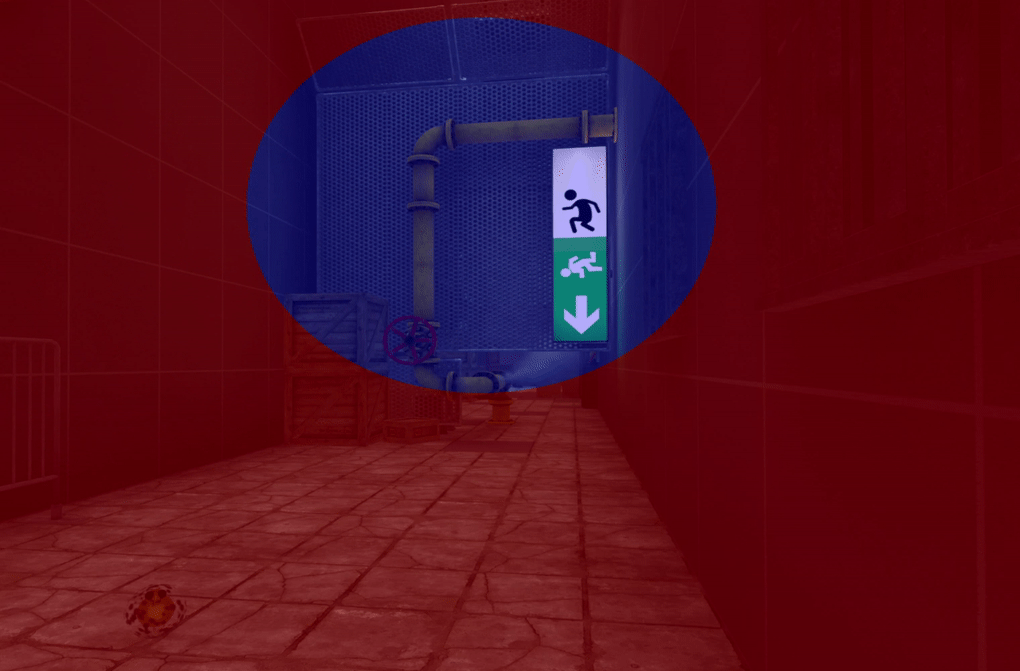
Accessing VRSS in the NVIDIA control panel
VRSS has two modes in the NVIDIA Control Panel: Adaptive and Always On.
Adaptive mode
This mode takes performance limits into consideration and tries to maximize the size of the supersampling region without hindering the VR experience. The size of the foveated region grows and shrinks in proportion to the available GPU headroom.
Figure 4. Depicting the dynamic sizing of the foveated region in Adaptive mode. The supersampled region is depicted with a green mask. Supersampled region shrinks and grows based on the GPU load (scene complexity).
Always On mode
A fixed-size foveated region is always supersampled, providing maximum image quality improvements. The size of the foveated region is adequate to cover the central portion of the user’s field of view. This mode helps users perceive the maximum IQ gains possible for a given title using VRSS. However, this may also result in frame drops for applications that are performance intensive.
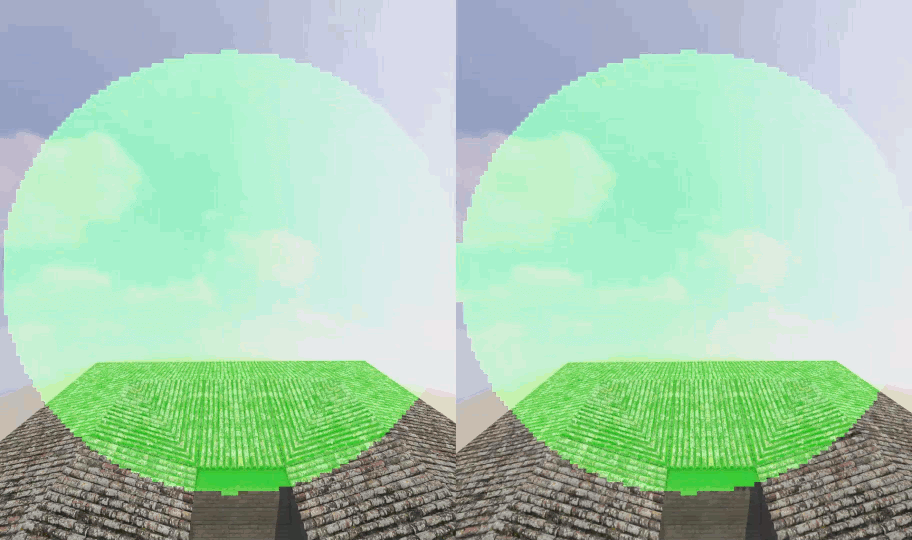
Under the hood
VRSS does not require any developer integration. The entire feature is implemented and handled inside the NVIDIA display driver, provided that the application is compatible and profiled by NVIDIA.
The NVIDIA display driver handles several pieces of functionality internally:
- Frame resources tracking—The driver keeps track of the resources encountered per frame and builds a heuristic model to flag a scenario where VRS could be invoked. Specifically, it notes the MSAA level to configure the VRS shading rate: the supersampling factor to be used at the center of the image. It provides render target parameters for configuring the VRS mask.
- Frame render monitoring—This involves measuring the rendering load across frames, the current frame rate of the application, the target frame rate based on the HMD refresh rate, and so on. This also computes the rendering stats required for the Adaptive mode.
- VRS enablement—As mentioned earlier, VRS gives you the ability to configure shading rate across the render surface. This is done with a shading rate mask and shading rate lookup table. For more information, see Turing Variable Rate Shading in VRWorks. The VRS infrastructure setup handles the configuration of the VRS mask and VRS shading rate table lookup. The VRS framework is configured based on the performance stats:
- VRS mask—The size of the central region mask is configured based on the available headroom.
- VRS shading rates—Configured based on the MSAA buffer sample count.
- Gaze data per frame—For VRSS 2, a component supplies the latest gaze data per frame. This involves a direct data transfer between the driver and the eye-tracking vendor platform. This data is used to configure the VRS foveation mask.

Developer guidance
For an application to take advantage of VRSS, you must submit your game or application to NVIDIA for VRSS validation. If approved, the game or application is added to an approved list within the NVIDIA driver.
Here are the benefits for a VRSS-supported game or application:
- The foveated region is now dynamic: Integration with Tobii Eye Tracking software.
- Zero coding: No developer integration required to work with game or application.
- Improved user experience: The user experiences VR content with added clarity.
- Ease of use: User controlled on/off supersampling in the NVIDIA Control Panel.
- Performance modes: Adaptive (fps priority) or Always On.
- No maintenance: Technology encapsulated at the driver level.
Pavlov VR | L.A. Noire VR | Mercenary 2: Silicon Rising |
Robo Recall | Eternity Warriors VR | Mercenary 2: Silicon Rising |
Serious Sam VR: The Last Hope | Hot Dogs Horseshoes & Hand Grenades | Special Force VR: Infinity War |
Talos Principle VR | Boneworks | Doctor Who: The Edge of time-VR |
Battlewake | Lone Echo | VRChat |
Job Simulator | Rec Room | PokerStars VR |
Spiderman Homecoming VR | Rick & Morty Simulator: Virtual Rick-ality | Budget Cuts 2: Mission Insolvency |
In Death | Skeet: VR Target Shooting | The Walking Dead: Saints & Sinners |
Killing Floor: Incursion | SpiderMan far from home | Onward VR |
Space Pirate Trainer | Sairento VR | Medal of Honor: Above and Beyond |
The Soulkeeper VR | Raw Data | Sniper Elite VR |
Game and application compatibility
To make use of VRSS, applications must meet the following requirements:
- Turing architecture
- DirectX 11 VR applications
- Forward rendered with MSAA
- Eye-tracking software integrated with VRSS
Supersampling needs an MSAA buffer. The level of supersampling is based on the underlying number of samples used in the MSAA buffer. The foveated region is shaded 2x for 2x MSAA, 4x supersampled for 4x MSAA, and so on. The maximum shading rate applied for supersampling is 8x. The higher the MSAA level, the greater the supersampling effect will be.
Content suitability
Content that benefits from supersampling benefits from VRSS as well. Supersampling not only mitigates aliasing but also brings out details in an image. The degree of quality improvement varies across content.

Supersampling shines when it encounters the following types of content:
- High-resolution textures
- High frequency materials
- Textures with alpha channels – fences, foliage, menu icons, text, and so on
Conversely, supersampling does not improve IQ for:
- Flat shaded geometry
- Textures and materials with low level of detail
Come onboard!
VRSS leverages VRS technology for selective supersampling and does not require application integration. VRSS is available in NVIDIA Driver R465, and applications are required to support DX11, forward rendering, and MSAA.
Submit your game and application to NVIDIA for VRSS consideration.
If you want finer-grained control of how VRS is applied, we recommend using the explicit programming APIs of VRWorks – Variable Rate Shading (VRS). Accessing the full power of VRS, you can implement a variety of shader sampling rate optimizations, including lens-matched shading, content-adaptive shading, and gaze-tracked shading.