submitted by /u/r42in
[visit reddit] [comments]
Hi! A newbie Here. I am working on a Gesture recognition project with mi band and I need to collect the sensor data from the band. Is there any way to get realtime data from the accelerometer of the band?
Notify App for Mi Band does track realtime data from the accelerometer but is there a way to get that realtime data on the pc for gesture recognition?
Any advice would on this would be really appreciated.
submitted by /u/infinitypenetrator
[visit reddit] [comments]
Using AI researchers have developed a new method for turning X-ray data into 3D visualizations, hundreds of times faster than traditional methods.
A team of scientists from Argonne National Laboratory developed a new method for turning X-ray data into visible, 3D images with the help of AI. The study, published in Applied Physics Reviews, develops a computational framework capable of taking data from the lab’s Advanced Photon Source (APS) and creating 3D visualizations hundreds of times faster than traditional methods.
“In order to make full use of what the upgraded APS will be capable of, we have to reinvent data analytics. Our current methods are not enough to keep up. Machine learning can make full use and go beyond what is currently possible,” Mathew Cherukara, a computational scientist at Argonne and study coauthor, said in a press release.
The advancement could have wide-ranging benefits to many areas of study relying on sizable amounts of 3D data, ranging from astronomy to nanoscale imaging.
Described as one of the most technologically complex machines in the world, the APS uses extremely bright X-ray beams to help researchers see the structure of materials at the molecular and atomic level. As these beams of light bounce off an object, detectors collect them in the form of data. With time and complex computations, this data is converted into images, revealing the object’s structure.
However, detectors are unable to capture all the beam data, leaving missing pieces of information. The researchers fill this gap by using neural networks that train computer models to identify objects and visualize an image, based on the raw data it is fed.
With 3D images this can be extremely timely due to the amount of information processed.
“We used computer simulations to create crystals of different shapes and sizes, and we converted them into images and diffraction patterns for the neural network to learn. The ease of quickly generating many realistic crystals for training is the benefit of simulations,” said Henry Chan, an Argonne postdoctoral researcher, and study coauthor.
The work for the new computational framework, known as 3D-CDI-NN, was developed using GPU resources at Argonne’s Joint Laboratory for System Evaluation, consisting of NVIDIA A100 and RTX 8000 GPUs.
“This paper… greatly facilitates the imaging process. We want to know what a material is, and how it changes over time, and this will help us make better pictures of it as we make measurements,” said Stephan Hruszkewycz, study coauthor and physicist with Argonne’s Materials Science Division.
Read more >>>
Read the full article in Applied Physics Reviews >>>
In this update, we look at the ways NVIDIA TensorRT and the Triton Inference Server can help your business deploy high-performance models with resilience at scale.
In Case You Missed It (ICYMI) is a series in which we spotlight essential talks, whitepapers, blogs, and success stories showcasing NVIDIA technologies accelerating real world solutions.
In this update, we look at the ways NVIDIA TensorRT and the Triton Inference Server can help your business deploy high-performance models with resilience at scale. We start with an in-depth, step-by-step introduction to TensorRT and Triton. Next, we dig into exactly how Triton and Clara Deploy complement each other in your healthcare use cases. Finally, to round things out our whitepaper covers exactly what you’ll need to know when migrating your applications to Triton.
TensorRT and Triton in Practice
On-Demand: Inception Café – Accelerating Deep Learning Inference with NVIDIA TensorRT and Triton
A step-by-step walkthrough applying NVIDIA TensorRT and Triton in conjunction with NVIDIA Clara Deploy.
Watch >
Whitepaper: Inception Café – Migrating Your Medical AI App to Triton
This whitepaper explores the end-to-end process of migrating an existing medical AI application to Triton.
Read >
On-Demand: Introduction to TensorRT and Triton A Walkthrough of Optimizing Your First Deep Learning Inference Model
An overview of TensorRT optimization of a PyTorch model followed by deployment of the optimized model using Triton. By the end of this workshop, developers will see the substantial benefits of integrating TensorRT and get started on optimizing their own deep learning models.
Watch >
Clara Imaging
On-Demand: Clara Train 4.0 – 101 Getting Started
This session provides a walk through the Clara Train SDK features and capabilities with a set of Jupyter Notebooks covering a range of topics, including Medical Model Archives (MMARs), AI-assisted annotation, and AutoML. These features help data scientists quickly annotate, train, and optimize hyperparameters for their deep learning model.
Watch >
On-Demand: Clara Train 4.0 – 201 Federated Learning
This session delivers an overview of federated learning, a distributed AI model development technique that allows models to be created without transferring data outside of hospitals or imaging centers. The session will finish with a walkthrough of Clara Train federated learning capabilities by going through a set of Jupyter Notebooks.
Watch >
On-Demand: Medical Imaging AI with MONAI Bootcamp
MONAI is a freely available, community-supported, open-source PyTorch-based framework for deep learning in medical imaging. It provides domain-optimized foundational capabilities for developing medical imaging training workflows in a native PyTorch paradigm. This MONAI Bootcamp offers medical imaging researchers an architectural deep dive of MONAI and finishes with a walkthrough of MONAI’s capabilities through a set of four Jupyter Notebooks.
Watch >
Clara Guardian
On-Demand: Clara Guardian 101: A Hello World Walkthrough on the Jetson Platform
NVIDIA Clara Guardian provides healthcare-specific pretrained models and sample applications that can significantly reduce the time-to-solution for developers building smart-hospital applications. It targets three categories—public safety (thermal screening, mask detection, and social distancing monitoring), patient care (patient monitoring, fall detection, and patient engagement), and operational efficiency (operating room workflow automation, surgery analytics, and contactless control). In this session, attendees will get a walkthrough of how to use Clara Guardian on the Jetson NX platform, including how to use the pretrained models for tasks like automatic speech recognition and body pose estimation.
Watch >
Clara Parabricks
On-Demand: GPU-Accelerated Genomics Using Clara Parabricks, Gary Burnett
NVIDIA Clara Parabricks is a software suite for performing secondary analysis of next generation sequencing (NGS) DNA and RNA data. A major benefit of Parabricks is that it is designed to deliver results at blazing fast speeds and low cost. Parabricks can analyze whole human genomes in under 30 minutes, compared to about 30 hours for 30x WGS data. In this session, attendees will take a guided tour of the Parabricks suite featuring live examples and real world applications.
Watch >
Clara AGX
On-Demand: Using Ethernet to Stream High-Throughput, Low-Latency Medical Sensor Data
Medical sensors in various medical devices generate high-throughput data. System designers are challenged to move the sensor data to the GPU for processing. Over the last decade, Ethernet speeds have increased from 10G to 100G, enabling new ways to meet this challenge. We’ll explore three technologies from NVIDIA that make streaming high-throughput medical sensor data over Ethernet easy and efficient — NVIDIA Networking ConnectX NICs, Rivermax SDK with GPUDirect, and Clara AGX. Learn about the capabilities of each of these technologies and explore examples of how these technologies can be leveraged by several different types of medical devices. Finally, a step-by-step demo will walk attendees through installing the software, initializing a link, and testing for throughput and CPU overhead.
Watch >
Recommended Hardware

Delivers real-time AI and imaging for medical devices. By combining low-powered, NVIDIA Jetson AGX Xavier and RTX GPU with the NVIDIA Clara AGX SDK and the NVIDIA EGX stack, it’s easy to securely provision and remotely manage fleets of distributed medical instruments.
Learn more >
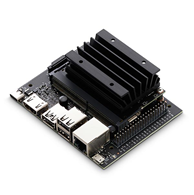
Discover the power of AI and robotics with the NVIDIA Jetson Nano 2GB Developer Kit. It’s small, powerful, and priced for everyone.
Learning by doing is key for anyone new to AI and robotics, and this developer kit is ideal for hands-on projects.
Learn more >
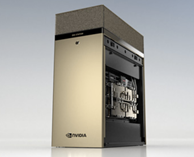
Data science teams need a dedicated AI resource that isn’t at the mercy of other areas within their organization: a purpose-built AI system without compromise that can handle all the jobs that busy data scientists can throw at it, an accelerated AI platform fully optimized across hardware and software for maximum performance.
Learn more >
Inception Spotlight
NEW on NGC: Simplify and Unify Biomedical Analytics with Vyasa
Learn how Vyasa Analytics leverages Clara Discovery, Triton Inference Server, RAPIDS, and DGX to develop solutions for pharmaceutical and biotechnology companies. Vyasa Analytics solutions are available from the NVIDIA NGC catalog for rapid evaluation and deployment.
Read >
Do you have a startup? Join NVIDIA Inception’s global network of over 8,000 startups.
Introduction RAPIDS Accelerator for Apache Spark v21.06 is here! You may notice right away that we’ve had a huge leap in version number since we announced our last release. Don’t worry, you haven’t missed anything. RAPIDS Accelerator is built on cuDF, part of the RAPIDS ecosystem. RAPIDS transitioned to calendar versioning (CalVer) in the last … Continued
Introduction
RAPIDS Accelerator for Apache Spark v21.06 is here! You may notice right away that we’ve had a huge leap in version number since we announced our last release. Don’t worry, you haven’t missed anything. RAPIDS Accelerator is built on cuDF, part of the RAPIDS ecosystem. RAPIDS transitioned to calendar versioning (CalVer) in the last release, and, from now on, our releases will follow the same convention.
We like CalVer because it is simple, and this new release is all about making your data science work simple as well. Of course, we’ve made changes to accommodate new versions of Apache Spark, but we’ve also simplified installation. We’ve added a profiling tool so it’s easier to identify the best workloads to run on the GPU.
There is a host of new functions to make life working with data easier. And we’ve expanded our community: if you are a Cloudera or an Azure user, GPU acceleration is more straightforward than ever. Let’s get into the details.
Updates
We added support for Apache Spark version 3.1.2 and Databricks 8.2ML GPU runtime.
To simplify the installation of the plug-in, we now have a single RAPIDS cuDF jar that works with all versions of NVIDIA CUDA 11.x. The jar was tested with CUDA 11.0 and 11.2 and relies on CUDA enhanced compatibility to work with any version of CUDA 11.
Profling and qualification tool
The RAPIDS Accelerator for Apache Spark now has an early release of the tool to analyze Spark logs to find jobs that are a good fit for GPU acceleration, as well as, profile jobs running with the plug-in. If applied to a single application, the tool looks at CPU event logs. In the case of multiple applications, the tool filters out individual application event logs and then provides information about the percentage of the runtime spent in SQL/Dataframe operations. It also calculates a breakdown of runtime spent on IO compared to Computation compared to Shuffle for these operations.
The profiling tool gives users information to help them debug their jobs. Key information it provides is Spark version, Spark properties (including those provided by the plug-in). Hadoop properties, lists of failed jobs and failed executors, query duration comparisons for all the queries in the input event logs, and the data format and storage type used.
As the tool is still in the early stages, we are excited to hear back from users on how we can improve the tool and their experience.
New functionality
This new release has additional functionality for arrays and structs. We can now sort on struct keys, have structs with map values, and cache structs. We can support concatenation of array columns, creation of 2D arrays, partition on arrays, and more. We’ve also expanded windowing lead/lag to support arrays. And range windows now support non-timestamp order by expressions. One of the important scaling capabilities is enabling large joins (for example, joins with large skew) to spill out of GPU memory and complete successfully. In addition, GPUDIRECT Storage has been integrated into the plug-in to enable Direct Memory Access (DMA) between storage and GPUs with increased bandwidth and lower latency to reduce I/O overhead for spilling and improve performance. For a detailed list of new features, please refer to the 21.06.0 changelog on the GitHub site.
Growing community
NVIDIA and Cloudera have continued to expand their partnership. Cloudera Data Platform (CDP) integration with RAPIDS Accelerator will be generally available on CDP PVC Base 7.1.6 release from July 15. With this integration, on-prem (private cloud) customers can accelerate their ETL workloads with NVIDIA-Certified systems and Cloudera Data Platform. See the press release from Cloudera here and check out our joint webinar here.
We’re also excited to let you know that NVIDIA and Microsoft have teamed to bring RAPIDS Accelerator to Azure Synapse. Support for Accelerator is now built-in, and customers can use NVIDIA GPUs for Apache Spark applications with no-code change and with an experience identical to a CPU cluster.
Coming soon
RAPIDS Accelerator for Apache Spark is a year old and growing fast. Our next release will expand read/write support for Parquet and ORC data formats, add operator support for Struct, Map, and List data types, increase stability and deliver an initial implementation of Out of Core Group by operations. Look forward to our next release in August, and in the meantime, follow all our developments on GitHub and blogs.
As part of NVIDIA’s collaboration with Mozilla Common Voice, the models trained on this and other public datasets are made available for free via an open-source toolkit called NVIDIA NeMo.
NVIDIA and Mozilla are proud to announce the latest release of the Common Voice dataset, with over 13,000 hours of crowd-sourced speech data, and adding another 16 languages to the corpus.
Common Voice is the world’s largest open data voice dataset and designed to democratize voice technology. It is used by researchers, academics, and developers around the world. Contributors mobilize their own communities to donate speech data to the MCV public database, which anyone can then use to train voice-enabled technology. As part of NVIDIA’s collaboration with Mozilla Common Voice, the models trained on this and other public datasets are made available for free via an open-source toolkit called NVIDIA NeMo.
Highlights of this release include:
- Common Voice dataset release is now 13,905 hours, an increase of 4,622 hours from the previous release.
- Introduces 16 new languages to the Common Voice dataset: Basaa, Slovak, Northern Kurdish, Bulgarian, Kazakh, Bashkir, Galician, Uyghur, Armenian, Belarusian, Urdu, Guarani, Serbian, Uzbek, Azerbaijani, Hausa.
- The top five languages by total hours are English (2,630 hours), Kinyarwanda (2,260), German (1,040), Catalan (920), and Esperanto (840).
- Languages that have increased the most by percentage are Thai (almost 20x growth, from 12 hours to 250 hours), Luganda (9x growth, from 8 hours to 80 hours), Esperanto (more than 7x growth, from 100 hours to 840 hours), and Tamil (more than 8x growth, from 24 hours to 220 hours).
- The dataset now features over 182,000 unique voices, a 25% growth in contributor community in just six months.
Pretrained Models:
NVIDIA has released multilingual speech recognition models in NGC for free as part of the partnership mission to democratize voice technology. NeMo is an open-source toolkit for researchers developing state-of-the-art conversational AI models. Researchers can further fine-tune these models on multilingual datasets. See an example in this notebook that fine tunes an English speech recognition model on the MCV Japanese dataset.
Contribute Your Voice, and Validate Samples:
The dataset relies on the amazing effort and contribution from many communities across the world. Take the time to feed back into the dataset by recording your voice and validating samples from other contributors: https://commonvoice.mozilla.org/speak
You can download the latest MCV dataset from https://commonvoice.mozilla.org/datasets, including the repo for full stats https://github.com/common-voice/cv-dataset/, and NVIDIA NeMo from NGC Catalog and GitHub.
Dataset ‘Ask Me Anything’:
August 4, 2021 from 3:00 – 4:00 p.m. UTC / 2:00 – 3:00 p.m. EDT / 11:00 a.m. – 12:00 p.m. PDT:
In celebration of the dataset release, on August 4th Mozilla is hosting an AMA discussion with Lead Engineer Jenny Zhang. Jenny will be available to answer your questions live, to join and ask a question please use the following AMA discourse topic.
Hello guys,
So I am doing a project where I have to identify an object and then identify its colour and the bounding box must be of the same colour as of the detected object. Please help if you guys have any ideas.
submitted by /u/BeerThePain
[visit reddit] [comments]
What output nodes should I use?
For context, I’m working with Mozilla’s DeepSpeech (version 0.1.0) to transcribe audios into text. I have a checkpoint folder with these files: model.data-00000, model.index, and model.meta
I need to convert the data file to a .pb file (protobuf). I’ve tried all the links in stack overflow, but nothing seems to be working. I’m guessing that maybe I’m getting my output nodes wrong, so is there a way to know what output nodes are relevant? I have over a 100 in the data file and if I convert all of that to .pb, it’ll be 1.5 GB in size. And if I try to transcribe with such a large frozen model, DeepSpeech gives me a “core dumped” error. So is there a way to know what output nodes are relevant?
I’m not sure if my question makes sense as my brain is scrambled from sitting with this for days now.
Please help anyone! And thank you!
submitted by /u/Due-Grade-756
[visit reddit] [comments]
This is a guest submitted post by Natnael Kebede, Co-founder and Chief NERD at New Era Research and Development Center It was one year ago in a random conversation that a friend told me about a piece of hardware in excitement. At that point I never imagined how that conversation would have the potential to … Continued
This is a guest submitted post by Natnael Kebede, Co-founder and Chief NERD at New Era Research and Development Center
It was one year ago in a random conversation that a friend told me about a piece of hardware in excitement. At that point I never imagined how that conversation would have the potential to impact my life. My name is Natnael Kebede, the Co-founder and Chief NERD at New Era Research and Development (NERD) Center in Ethiopia. NERD is a center that provides a hacker space, educational content, and research for Ethiopian youth to create a better Ethiopia Africa and World
That piece of hardware was the Jetson Nano. I went over to my house and started researching about the Jetson Nano that night. From that point onward, I could not stop following up and researching about the NVIDIA edge computing concept. This is a story about how a single conversation helped me build a career and a community around an idea.
The conversation we had with my friend was about edge computing disrupting the new AI-development environment. For a country like Ethiopia, AI is usually considered a luxury than a necessity. This is due to the perception of universities, investors, startups and the government have about AI. All of them think about expensive high-performance computers and lack of experienced professionals in the field. Very soon, I realized how the edge computing solution could be a peaceful weapon to change the attitude towards AI in Ethiopia. Me and my partner decided to buy the Jetson Nano and put our name on the map.
The learning process was much easier and efficient when we started experimenting with the Jetson hands-on. It was coincidentally by that time we were invited to the first Ethiopian AI Summit to showcase any project related to AI. We decided to go for building an edge solution. Our research team side of the problem was to build a system that reads a streamed video, and use the Jetson to identify any desired inference at the edge. The application could range from counting cars in a connected traffic junctions and detecting license plates, to an agricultural solution where any flying or ground vehicle feeding a video of a farm to detect plantation health issues/counting fruits. We started off with the traffic management system. We organized a team of three engineering interns and myself to build a prototype in less than eight weeks.

We got the whole thing figured out after going through a lot of reading. Finding answers was not the easiest thing as it was rare to find publications on the Nano. Meanwhile, a friend gave our contact to one of the NVIDIA Emerging Chapters Program leads, and we had a conversation about the program. It was the best timing. Although we wish we had known about the program earlier to access the DLI (NVIDIA Deep Learning Institute) courses, we kept going with a hope that the program will enable future projects with hands-on experience and technical training. The project was finally presented at the Summit and we had the chance to pitch the idea of edge computing to the Prime Minster of Ethiopia His Excellency Dr. Abiy Ahmed. We received promising feedback from him in regards to taking the project forward into implementation on the years to come.

After the Summit we knew we needed more talent to recruit and inspire. We launched a short-term training on the Jetson Nano. It took our team eight weeks to build the traffic management system. We made sure the training takes the same duration of time for the students to build a final project at the end. We value open-sourcing the project. The output from these trainings are projects to be open-source so we can build our community bigger. Since we have limited resources, we only provided 25 students in three groups. Currently, we registered 13 students and the first batch is currently taking trainings. We are using the free DLI courses we were granted as part of the Emerging Chapters Program as guideline to our curriculum. We will soon provide them with free course vouchers after filtering consistent training participants.

Our goal is to create a community of enthusiasts, hobbyist, engineers and developers passionate about edge computing solutions in Agriculture and Smart City projects. We do this by consistently engaging with the tech community in Addis Ababa, Ethiopia. We organized an open seminar last week where we showcased our projects and also talked about edge computing. We were able to inspire more students for our program. Our team members are all enjoying the free DLI courses and we will be coming up with something much larger very soon. I want to personally thank the NVIDIA Emerging Chapters Program for all the support and resources. On behalf of our team, we are grateful to be part of the program. Very soon we would love to present our work for other partners!
The AI-based traffic control system is now on GitHub.
To learn more about NERD and to stay up-to-date, follow us on Instagram @nerds_center.
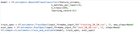
![]() |
I am a little bit confused about the the usage of steps in train with boosted trees. I get the meaning of step when I use it with a incremental model like neural net, but I don’t get when to use it with n_trees of the boosted trees. I guess that 1 step means that I train the whole n_trees one time. Am I correct? submitted by /u/Tokukawa |