submitted by /u/MLtinkerer
[visit reddit] [comments]
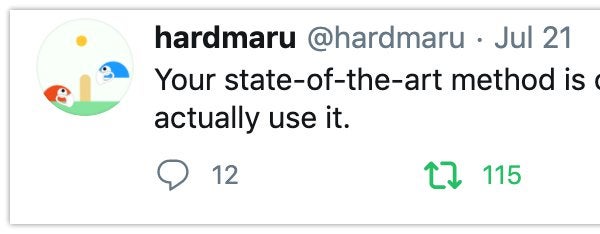
submitted by /u/MLtinkerer
[visit reddit] [comments]
For my thesis, I am attempting to detect faults/inconsistencies in 3D prints.
Generating data takes a long time (because the print process takes a long time). For this reason my dataset is limited. I’ve got two classes which each have about 100-150 images each. This adds up to a total of about 250-300 images.Then I augmented those images 8 times with rotations and flips. I first tried to train on EfficientdetD0 but the results were pretty disappointing. Perhaps only a quarter of errors were getting detected.
Someone on this subreddit told me I should use an architecture like ” SSD ResNet50 V1 FPN 640×640 (RetinaNet50) ” because this appearantly works better for small datasets, though I don’t know why. So I went and tried it. I trained with the same parameters as before and for roughly the same time. The results were even worse.
Right now I would like to train a model from scratch and compare the results to the results I got using transfer learning, however I have no clue on how I should get started with this. I’ve been googling about but I haven’t found a clear explanation just yet. Could somebody please point me in the right direction?
Also why should SSD ResNet50 V1 FPN 640×640 (RetinaNet50) work better with smaller datasets, and why shouldn’t I just use ResNet 151? From what I’ve gathered this should work better than 50 in my case because it goed deeper, no?
submitted by /u/007Nick700
[visit reddit] [comments]
submitted by /u/ashmortar
[visit reddit] [comments]
NVIDIA will host a conference call on Wednesday, February 24, at 2 p.m. PT (5 p.m. …
Model-free reinforcement learning has been successfully demonstrated across a range of domains, including robotics, control, playing games and autonomous vehicles. These systems learn by simple trial and error and thus require a vast number of attempts at a given task before solving it. In contrast, model-based reinforcement learning (MBRL) learns a model of the environment (often referred to as a world model or a dynamics model) that enables the agent to predict the outcomes of potential actions, which reduces the amount of environment interaction needed to solve a task.
In principle, all that is strictly necessary for planning is to predict future rewards, which could then be used to select near-optimal future actions. Nevertheless, many recent methods, such as Dreamer, PlaNet, and SimPLe, additionally leverage the training signal of predicting future images. But is predicting future images actually necessary, or helpful? What benefit do visual MBRL algorithms actually derive from also predicting future images? The computational and representational cost of predicting entire images is considerable, so understanding whether this is actually useful is of profound importance for MBRL research.
In “Models, Pixels, and Rewards: Evaluating Design Trade-offs in Visual Model-Based Reinforcement Learning”, we demonstrate that predicting future images provides a substantial benefit, and is in fact a key ingredient in training successful visual MBRL agents. We developed a new open-source library, called the World Models Library, which enabled us to rigorously evaluate various world model designs to determine the relative impact of image prediction on returned rewards for each.
World Models Library
The World Models Library, designed specifically for visual MBRL training and evaluation, enables the empirical study of the effects of each design decision on the final performance of an agent across multiple tasks on a large scale. The library introduces a platform-agnostic visual MBRL simulation loop and the APIs to seamlessly define new world-models, planners and tasks or to pick and choose from the existing catalog, which includes agents (e.g., PlaNet), video models (e.g., SV2P), and a variety of DeepMind Control tasks and planners, such as CEM and MPPI.
Using the library, developers can study the effect of a varying factor in MBRL, such as the model design or representation space, on the performance of the agent on a suite of tasks. The library supports the training of the agents from scratch, or on a pre-collected set of trajectories, as well as evaluation of a pre-trained agent on a given task. The models, planning algorithms and the tasks can be easily mixed and matched to any desired combination.
To provide the greatest flexibility for users, the library is built using the NumPy interface, which enables different components to be implemented in either TensorFlow, Pytorch or JAX. Please look at this colab for a quick introduction.
Impact of Image Prediction
Using the World Models Library, we trained multiple world models with different levels of image prediction. All of these models use the same input (previously observed images) to predict an image and a reward, but they differ on what percentage of the image they predict. As the number of image pixels predicted by the agent increases, the agent performance as measured by the true reward generally improves.
Interestingly, the correlation between reward prediction accuracy and agent performance is not as strong, and in some cases a more accurate reward prediction can even result in lower agent performance. At the same time, there is a strong correlation between image reconstruction error and the performance of the agent.
This phenomenon is directly related to exploration, i.e., when the agent attempts more risky and potentially less rewarding actions in order to collect more information about the unknown options in the environment. This can be shown by testing and comparing models in an offline setup (i.e., learning policies from pre-collected datasets, as opposed to online RL, which learns policies by interacting with an environment). An offline setup ensures that there is no exploration and all of the models are trained on the same data. We observed that models that fit the data better usually perform better in the offline setup, and surprisingly, these may not be the same models that perform the best when learning and exploring from scratch.
Conclusion
We have empirically demonstrated that predicting images can substantially improve task performance over models that only predict the expected reward. We have also shown that the accuracy of image prediction strongly correlates with the final task performance of these models. These findings can be used for better model design and can be particularly useful for any future setting where the input space is high-dimensional and collecting data is expensive.
If you’d like to develop your own models and experiments, head to our repository and colab where you’ll find instructions on how to reproduce this work and use or extend the World Models Library.
Acknowledgement:
We would like to give special recognition to multiple researchers in the Google Brain team and co-authors of the paper: Mohammad Taghi Saffar, Danijar Hafner, Harini Kannan, Chelsea Finn and Sergey Levine.
To measure the impact of outdoor advertising, Brazil-based DataVisiooh, a member of NVIDIA Inception, developed an AI solution to capture performance analytics in real-time.
“Our platform goes beyond simply counting the flow of people and vehicles and shows advanced data such as demographic information (gender, age group), screen viewing time, exposure time, and even how many people looked at the screen.” the company said.
The company’s platform uses NVIDIA Jetson modules with TensorFlow and TensorRT to process the various cameras and sensors at the edge.
DataVisiooh says their system will help support marketing agencies and companies with campaign performance verification and media planning.
“Our real-time performance data and dashboards, collected continuously via sensors and processed by our proprietary algorithms, are the most up-to-date on the market,” the company said.
Not many can claim to be a computer programmer, nonfiction author and poet, but Brian Christian has established himself as all three. Christian has just released his newest book, The Alignment Problem, which delves into the disparity that occurs when AI models don’t do exactly what they’re intended to do. The book follows on the Read article >
The post Making Machines More Human: Author Brian Christian Talks the Alignment Problem appeared first on The Official NVIDIA Blog.
![]() |
submitted by /u/KayRice [visit reddit] [comments] |
I’m using image_dataset_from_directory method to load images from file. And I’ve prepared an label.csv file for each image filename.
However, after my first training, all of the prediction makes predict for same class. I’d checked up the directory folder and don’t know why.
I want to check up the label and image pair to see if any bug, but I don’t find a simple way to do this, so I combine the other custom image_dataset_from_directory function that returns image_path, and compare with the order of given label:
def image_dataset_from_directory(directory, labels='inferred', label_mode='int', class_names=None, color_mode='rgb', batch_size=32, image_size=(256, 256), shuffle=True, seed=None, validation_split=None, subset=None, interpolation='bilinear', follow_links=False): if labels != 'inferred': if not isinstance(labels, (list, tuple)): raise ValueError( '`labels` argument should be a list/tuple of integer labels, of ' 'the same size as the number of image files in the target ' 'directory. If you wish to infer the labels from the subdirectory ' 'names in the target directory, pass `labels="inferred"`. ' 'If you wish to get a dataset that only contains images ' '(no labels), pass `label_mode=None`.') if class_names: raise ValueError('You can only pass `class_names` if the labels are ' 'inferred from the subdirectory names in the target ' 'directory (`labels="inferred"`).') if label_mode not in {'int', 'categorical', 'binary', None}: raise ValueError( '`label_mode` argument must be one of "int", "categorical", "binary", ' 'or None. Received: %s' % (label_mode,)) if color_mode == 'rgb': num_channels = 3 elif color_mode == 'rgba': num_channels = 4 elif color_mode == 'grayscale': num_channels = 1 else: raise ValueError( '`color_mode` must be one of {"rbg", "rgba", "grayscale"}. ' 'Received: %s' % (color_mode,)) interpolation = image_preprocessing.get_interpolation(interpolation) dataset_utils.check_validation_split_arg( validation_split, subset, shuffle, seed) if seed is None: seed = np.random.randint(1e6) image_paths, labels, class_names = dataset_utils.index_directory( directory, labels, formats=WHITELIST_FORMATS, class_names=class_names, shuffle=shuffle, seed=seed, follow_links=follow_links) if label_mode == 'binary' and len(class_names) != 2: raise ValueError( 'When passing `label_mode="binary", there must exactly 2 classes. ' 'Found the following classes: %s' % (class_names,)) image_paths, labels = dataset_utils.get_training_or_validation_split( image_paths, labels, validation_split, subset) dataset = paths_and_labels_to_dataset( image_paths=image_paths, image_size=image_size, num_channels=num_channels, labels=labels, label_mode=label_mode, num_classes=len(class_names), interpolation=interpolation) if shuffle: # Shuffle locally at each iteration dataset = dataset.shuffle(buffer_size=batch_size * 8, seed=seed) dataset = dataset.batch(batch_size) # Users may need to reference `class_names`. dataset.class_names = class_names return dataset, image_paths
And this is data loader:
train_dataset, train_path = image_dataset_from_directory( img_path, labels = labels, label_mode = 'binary', validation_split = 0.2, color_mode = 'rgb', subset = 'training', image_size = (IMAGE_WIDTH, IMAGE_HEIGHT), batch_size = BATCH_SIZE, seed = 123 )
While I print the image and label pair, it’s not matching:
for i in range(len(train_path)): filename = train_path[i].split('/')[-1] print('File: {} label: {}'.format(filename, labels[i])) It returns: File: class_1_img.png label: 1 File: class_1_img.png label: 2 ...
The unmatching image-label pair make the training meaningless.
How could I load image from directory and give label list to make them match order?
submitted by /u/Laurence-Lin
[visit reddit] [comments]
Today, we use the convLSTM introduced in a previous post to predict El Niño-Southern Oscillation (ENSO).
El Niño, la Niña
ENSO refers to a changing pattern of sea surface temperatures and sea-level pressures occurring in the equatorial Pacific. From its three overall states, probably the best-known is El Niño. El Niño occurs when surface water temperatures in the eastern Pacific are higher than normal, and the strong winds that normally blow from east to west are unusually weak. The opposite conditions are termed La Niña. Everything in-between is classified as normal.
ENSO has great impact on the weather worldwide, and routinely harms ecosystems and societies through storms, droughts and flooding, possibly resulting in famines and economic crises. The best societies can do is try to adapt and mitigate severe consequences. Such efforts are aided by accurate forecasts, the further ahead the better.
Here, deep learning (DL) can potentially help: Variables like sea surface temperatures and pressures are given on a spatial grid – that of the earth – and as we know, DL is good at extracting spatial (e.g., image) features. For ENSO prediction, architectures like convolutional neural networks (Ham, Kim, and Luo (2019)) or convolutional-recurrent hybrids1 are habitually used. One such hybrid is just our convLSTM; it operates on sequences of features given on a spatial grid. Today, thus, we’ll be training a model for ENSO forecasting. This model will have a convLSTM for its central ingredient.
Before we start, a note. While our model fits well with architectures described in the relevant papers, the same cannot be said for amount of training data used. For reasons of practicality, we use actual observations only; consequently, we end up with a small (relative to the task) dataset. In contrast, research papers tend to make use of climate simulations2, resulting in significantly more data to work with.
From the outset, then, we don’t expect stellar performance. Nevertheless, this should make for an interesting case study, and a useful code template for our readers to apply to their own data.
Data
We will attempt to predict monthly average sea surface temperature in the Niño 3.4 region3, as represented by the Niño 3.4 Index, plus categorization as one of El Niño, La Niña or neutral4. Predictions will be based on prior monthly sea surface temperatures spanning a large portion of the globe.
On the input side, public and ready-to-use data may be downloaded from Tokyo Climate Center; as to prediction targets, we obtain index and classification here.
Input and target data both are provided monthly. They intersect in the time period ranging from 1891-01-01 to 2020-08-01; so this is the range of dates we’ll be zooming in on.
Input: Sea Surface Temperatures
Monthly sea surface temperatures are provided in a latitude-longitude grid of resolution 1°. Details of how the data were processed are available here.
Data files are available in GRIB format; each file contains averages computed for a single month. We can either download individual files or generate a text file of URLs for download. In case you’d like to follow along with the post, you’ll find the contents of the text file I generated in the appendix. Once you’ve saved these URLs to a file, you can have R get the files for you like so:
purrr::walk( readLines("files"), function(f) download.file(url = f, destfile = basename(f)) )
From R, we can read GRIB files using stars. For example:
# let's just quickly load all libraries we require to start with library(torch) library(tidyverse) library(stars) library(viridis) library(ggthemes) torch_manual_seed(777) read_stars(file.path(grb_dir, "sst189101.grb"))
stars object with 2 dimensions and 1 attribute attribute(s): sst189101.grb Min. :-274.9 1st Qu.:-272.8 Median :-259.1 Mean :-260.0 3rd Qu.:-248.4 Max. :-242.8 NA's :21001 dimension(s): from to offset delta refsys point values x 1 360 0 1 Coordinate System importe... NA NULL [x] y 1 180 90 -1 Coordinate System importe... NA NULL [y]
So in this GRIB file, we have one attribute – which we know to be sea surface temperature – on a two-dimensional grid. As to the latter, we can complement what stars tells us with additional info found in the documentation:
The east-west grid points run eastward from 0.5ºE to 0.5ºW, while the north-south grid points run northward from 89.5ºS to 89.5ºN.
We note a few things we’ll want to do with this data. For one, the temperatures seem to be given in Kelvin, but with minus signs.5 We’ll remove the minus signs and convert to degrees Celsius for convenience. We’ll also have to think about what to do with the NAs that appear for all non-maritime coordinates.
Before we get there though, we need to combine data from all files into a single data frame. This adds an additional dimension, time, ranging from 1891/01/01 to 2020/01/12:
grb <- read_stars( file.path(grb_dir, map(readLines("files", warn = FALSE), basename)), along = "time") %>% st_set_dimensions(3, values = seq(as.Date("1891-01-01"), as.Date("2020-12-01"), by = "months"), names = "time" ) grb
stars object with 3 dimensions and 1 attribute attribute(s), summary of first 1e+05 cells: sst189101.grb Min. :-274.9 1st Qu.:-273.3 Median :-258.8 Mean :-260.0 3rd Qu.:-247.8 Max. :-242.8 NA's :33724 dimension(s): from to offset delta refsys point values x 1 360 0 1 Coordinate System importe... NA NULL [x] y 1 180 90 -1 Coordinate System importe... NA NULL [y] time 1 1560 NA NA Date NA 1891-01-01,...,2020-12-01
Let’s visually inspect the spatial distribution of monthly temperatures for one year, 2020:
ggplot() + geom_stars(data = grb %>% filter(between(time, as.Date("2020-01-01"), as.Date("2020-12-01"))), alpha = 0.8) + facet_wrap("time") + scale_fill_viridis() + coord_equal() + theme_map() + theme(legend.position = "none")
(#fig:unnamed-chunk-5)Monthly sea surface temperatures, 2020/01/01 – 2020/01/12.
Target: Niño 3.4 Index
For the Niño 3.4 Index, we download the monthly data and, among the provided features, zoom in on two: the index itself (column NINO34_MEAN) and PHASE, which can be E (El Niño), L (La Niño) or N (neutral).
nino <- read_table2("ONI_NINO34_1854-2020.txt", skip = 9) %>% mutate(month = as.Date(paste0(YEAR, "-", `MON/MMM`, "-01"))) %>% select(month, NINO34_MEAN, PHASE) %>% filter(between(month, as.Date("1891-01-01"), as.Date("2020-08-01"))) %>% mutate(phase_code = as.numeric(as.factor(PHASE))) nrow(nino)
1556
Next, we look at how to get the data into a format convenient for training and prediction.
Preprocessing Input
First, we remove all input data for points in time where ground truth data are still missing.
sst <- grb %>% filter(time <= as.Date("2020-08-01"))
Next, as is done by e.g. Ham, Kim, and Luo (2019), we only use grid points between 55° south and 60° north. This has the additional advantage of reducing memory requirements.
sst <- grb %>% filter(between(y,-55, 60)) dim(sst)
360, 115, 1560
As already alluded to, with the little data we have we can’t expect much in terms of generalization. Still, we set aside a small portion of the data for validation, since we’d like for this post to serve as a useful template to be used with bigger datasets.
sst_train <- sst %>% filter(time < as.Date("1990-01-01")) sst_valid <- sst %>% filter(time >= as.Date("1990-01-01"))
From here on, we work with R arrays.
sst_train <- as.tbl_cube.stars(sst_train)$mets[[1]] sst_valid <- as.tbl_cube.stars(sst_valid)$mets[[1]]
Conversion to degrees Celsius is not strictly necessary, as initial experiments showed a slight performance increase due to normalizing the input, and we’re going to do that anyway. Still, it reads nicer to humans than Kelvin.
sst_train <- sst_train + 273.15 quantile(sst_train, na.rm = TRUE)
0% 25% 50% 75% 100% -1.8000 12.9975 21.8775 26.8200 34.3700
Not at all surprisingly, global warming is evident from inspecting temperature distribution on the validation set (which was chosen to span the last thirty-one years).
sst_valid <- sst_valid + 273.15 quantile(sst_valid, na.rm = TRUE)
0% 25% 50% 75% 100% -1.800 13.425 22.335 27.240 34.870
The next-to-last step normalizes both sets according to training mean and variance.
train_mean <- mean(sst_train, na.rm = TRUE) train_sd <- sd(sst_train, na.rm = TRUE) sst_train <- (sst_train - train_mean) / train_sd sst_valid <- (sst_valid - train_mean) / train_sd
Finally, what should we do about the NA entries? We set them to zero, the (training set) mean. That may not be enough of an action though: It means we’re feeding the network roughly 30% misleading data. This is something we’re not done with yet.
sst_train[is.na(sst_train)] <- 0 sst_valid[is.na(sst_valid)] <- 0
Target
The target data are split analogously. Let’s check though: Are phases (categorizations) distributedly similarly in both sets?
nino_train <- nino %>% filter(month < as.Date("1990-01-01")) nino_valid <- nino %>% filter(month >= as.Date("1990-01-01")) nino_train %>% group_by(phase_code, PHASE) %>% summarise(count = n(), avg = mean(NINO34_MEAN))
# A tibble: 3 x 4 # Groups: phase_code [3] phase_code PHASE count avg <dbl> <chr> <int> <dbl> 1 1 E 301 27.7 2 2 L 333 25.6 3 3 N 554 26.7
nino_valid %>% group_by(phase_code, PHASE) %>% summarise(count = n(), avg = mean(NINO34_MEAN))
# A tibble: 3 x 4 # Groups: phase_code [3] phase_code PHASE count avg <dbl> <chr> <int> <dbl> 1 1 E 93 28.1 2 2 L 93 25.9 3 3 N 182 27.2
This doesn’t look too bad. Of course, we again see the overall rise in temperature, irrespective of phase.
Lastly, we normalize the index, same as we did for the input data.
train_mean_nino <- mean(nino_train$NINO34_MEAN) train_sd_nino <- sd(nino_train$NINO34_MEAN) nino_train <- nino_train %>% mutate(NINO34_MEAN = scale(NINO34_MEAN, center = train_mean_nino, scale = train_sd_nino)) nino_valid <- nino_valid %>% mutate(NINO34_MEAN = scale(NINO34_MEAN, center = train_mean_nino, scale = train_sd_nino))
On to the torch dataset.
Torch dataset
The dataset is responsible for correctly matching up inputs and targets.
Our goal is to take six months of global sea surface temperatures and predict the Niño 3.4 Index for the following month. Input-wise, the model will expect the following format semantics:
batch_size * timesteps * width * height * channels, where
batch_size is the number of observations worked on in one round of computations,
timesteps chains consecutive observations from adjacent months,
width and height together constitute the spatial grid, and
channels corresponds to available visual channels in the “image”.
In .getitem(), we select the consecutive observations, starting at a given index, and stack them in dimension one. (One, not two, as batches will only start to exist once the dataloader comes into play.)
Now, what about the target? Our ultimate goal was – is – predicting the Niño 3.4 Index. However, as you see we define three targets: One is the index, as expected; an additional one holds the spatially-gridded sea surface temperatures for the prediction month. Why? Our main instrument, the most prominent constituent of the model, will be a convLSTM, an architecture designed for spatial prediction. Thus, to train it efficiently, we want to give it the opportunity to predict values on a spatial grid. So far so good; but there’s one more target, the phase/category. This was added for experimentation purposes: Maybe predicting both index and phase helps in training?
Finally, here is the code for the dataset. In our experiments, we based predictions on inputs from the preceding six months (n_timesteps <- 6). This is a parameter you might want to play with, though.
n_timesteps <- 6 enso_dataset <- dataset( name = "enso_dataset", initialize = function(sst, nino, n_timesteps) { self$sst <- sst self$nino <- nino self$n_timesteps <- n_timesteps }, .getitem = function(i) { x <- torch_tensor(self$sst[, , i:(n_timesteps + i - 1)]) # (360, 115, n_timesteps) x <- x$permute(c(3,1,2))$unsqueeze(2) # (n_timesteps, 1, 360, 115)) y1 <- torch_tensor(self$sst[, , n_timesteps + i])$unsqueeze(1) # (1, 360, 115) y2 <- torch_tensor(self$nino$NINO34_MEAN[n_timesteps + i]) y3 <- torch_tensor(self$nino$phase_code[n_timesteps + i])$squeeze()$to(torch_long()) list(x = x, y1 = y1, y2 = y2, y3 = y3) }, .length = function() { nrow(self$nino) - n_timesteps } ) valid_ds <- enso_dataset(sst_valid, nino_valid, n_timesteps)
Dataloaders
After the custom dataset, we create the – pretty typical – dataloaders, making use of a batch size of 4.
batch_size <- 4 train_dl <- train_ds %>% dataloader(batch_size = batch_size, shuffle = TRUE) valid_dl <- valid_ds %>% dataloader(batch_size = batch_size)
Next, we proceed to model creation.
Model
The model’s main ingredient is the convLSTM introduced in a prior post. For convenience, we reproduce the code in the appendix.
Besides the convLSTM, the model makes use of three convolutional layers, a batchnorm layer and five linear layers. The logic is the following.
First, the convLSTM job is to predict the next month’s sea surface temperatures on the spatial grid. For that, we almost just return its final state, – almost: We use self$conv1 to reduce the number channels to one.
For predicting index and phase, we then need to flatten the grid, as we require a single value each. This is where the additional conv layers come in. We do hope they’ll aid in learning, but we also want to reduce the number of parameters a bit, downsizing the grid (strides = 2 and strides = 3, resp.) a bit before the upcoming torch_flatten().
Once we have a flat structure, learning is shared between the tasks of index and phase prediction (self$linear), until finally their paths split (self$cont and self$cat, resp.), and they return their separate outputs.
(The batchnorm? I’ll comment on that in the Discussion.)
model <- nn_module( initialize = function(channels_in, convlstm_hidden, convlstm_kernel, convlstm_layers) { self$n_layers <- convlstm_layers self$convlstm <- convlstm( input_dim = channels_in, hidden_dims = convlstm_hidden, kernel_sizes = convlstm_kernel, n_layers = convlstm_layers ) self$conv1 <- nn_conv2d( in_channels = 32, out_channels = 1, kernel_size = 5, padding = 2 ) self$conv2 <- nn_conv2d( in_channels = 32, out_channels = 32, kernel_size = 5, stride = 2 ) self$conv3 <- nn_conv2d( in_channels = 32, out_channels = 32, kernel_size = 5, stride = 3 ) self$linear <- nn_linear(33408, 64) self$b1 <- nn_batch_norm1d(num_features = 64) self$cont <- nn_linear(64, 128) self$cat <- nn_linear(64, 128) self$cont_output <- nn_linear(128, 1) self$cat_output <- nn_linear(128, 3) }, forward = function(x) { ret <- self$convlstm(x) layer_last_states <- ret[[2]] last_hidden <- layer_last_states[[self$n_layers]][[1]] next_sst <- last_hidden %>% self$conv1() c2 <- last_hidden %>% self$conv2() c3 <- c2 %>% self$conv3() flat <- torch_flatten(c3, start_dim = 2) common <- self$linear(flat) %>% self$b3() %>% nnf_relu() next_temp <- common %>% self$cont() %>% nnf_relu() %>% self$cont_output() next_nino <- common %>% self$cat() %>% nnf_relu() %>% self$cat_output() list(next_sst, next_temp, next_nino) } )
Next, we instantiate a pretty small-ish model. You’re more than welcome to experiment with larger models, but training time as well as GPU memory requirements will increase.
net <- model( channels_in = 1, convlstm_hidden = c(16, 16, 32), convlstm_kernel = c(3, 3, 5), convlstm_layers = 3 ) device <- torch_device(if (cuda_is_available()) "cuda" else "cpu") net <- net$to(device = device) net
An `nn_module` containing 2,389,605 parameters. ── Modules ─────────────────────────────────────────────────────────────────────────────── ● convlstm: <nn_module> #182,080 parameters ● conv1: <nn_conv2d> #801 parameters ● conv2: <nn_conv2d> #25,632 parameters ● conv3: <nn_conv2d> #25,632 parameters ● linear: <nn_linear> #2,138,176 parameters ● b1: <nn_batch_norm1d> #128 parameters ● cont: <nn_linear> #8,320 parameters ● cat: <nn_linear> #8,320 parameters ● cont_output: <nn_linear> #129 parameters ● cat_output: <nn_linear> #387 parameters
Training
We have three model outputs. How should we combine the losses?
Given that the main goal is predicting the index, and the other two outputs are essentially means to an end, I found the following combination rather effective:
# weight for sea surface temperature prediction lw_sst <- 0.2 # weight for prediction of El Nino 3.4 Index lw_temp <- 0.4 # weight for phase prediction lw_nino <- 0.4
The training process follows the pattern seen in all torch posts so far: For each epoch, loop over the training set, backpropagate, check performance on validation set.
But, when we did the pre-processing, we were aware of an imminent problem: the missing temperatures for continental areas, which we set to zero. As a sole measure, this approach is clearly insufficient. What if we had chosen to use latitude-dependent averages? Or interpolation? Both may be better than a global average, but both have their problems as well. Let’s at least alleviate negative consequences by not using the respective pixels for spatial loss calculation. This is taken care of by the following line below:
sst_loss <- nnf_mse_loss(sst_output[sst_target != 0], sst_target[sst_target != 0])
Here, then, is the complete training code.
optimizer <- optim_adam(net$parameters, lr = 0.001) num_epochs <- 50 train_batch <- function(b) { optimizer$zero_grad() output <- net(b$x$to(device = device)) sst_output <- output[[1]] sst_target <- b$y1$to(device = device) sst_loss <- nnf_mse_loss(sst_output[sst_target != 0], sst_target[sst_target != 0]) temp_loss <- nnf_mse_loss(output[[2]], b$y2$to(device = device)) nino_loss <- nnf_cross_entropy(output[[3]], b$y3$to(device = device)) loss <- lw_sst * sst_loss + lw_temp * temp_loss + lw_nino * nino_loss loss$backward() optimizer$step() list(sst_loss$item(), temp_loss$item(), nino_loss$item(), loss$item()) } valid_batch <- function(b) { output <- net(b$x$to(device = device)) sst_output <- output[[1]] sst_target <- b$y1$to(device = device) sst_loss <- nnf_mse_loss(sst_output[sst_target != 0], sst_target[sst_target != 0]) temp_loss <- nnf_mse_loss(output[[2]], b$y2$to(device = device)) nino_loss <- nnf_cross_entropy(output[[3]], b$y3$to(device = device)) loss <- lw_sst * sst_loss + lw_temp * temp_loss + lw_nino * nino_loss list(sst_loss$item(), temp_loss$item(), nino_loss$item(), loss$item()) } for (epoch in 1:num_epochs) { net$train() train_loss_sst <- c() train_loss_temp <- c() train_loss_nino <- c() train_loss <- c() coro::loop(for (b in train_dl) { losses <- train_batch(b) train_loss_sst <- c(train_loss_sst, losses[[1]]) train_loss_temp <- c(train_loss_temp, losses[[2]]) train_loss_nino <- c(train_loss_nino, losses[[3]]) train_loss <- c(train_loss, losses[[4]]) }) cat( sprintf( "nEpoch %d, training: loss: %3.3f sst: %3.3f temp: %3.3f nino: %3.3f n", epoch, mean(train_loss), mean(train_loss_sst), mean(train_loss_temp), mean(train_loss_nino) ) ) net$eval() valid_loss_sst <- c() valid_loss_temp <- c() valid_loss_nino <- c() valid_loss <- c() coro::loop(for (b in valid_dl) { losses <- valid_batch(b) valid_loss_sst <- c(valid_loss_sst, losses[[1]]) valid_loss_temp <- c(valid_loss_temp, losses[[2]]) valid_loss_nino <- c(valid_loss_nino, losses[[3]]) valid_loss <- c(valid_loss, losses[[4]]) }) cat( sprintf( "nEpoch %d, validation: loss: %3.3f sst: %3.3f temp: %3.3f nino: %3.3f n", epoch, mean(valid_loss), mean(valid_loss_sst), mean(valid_loss_temp), mean(valid_loss_nino) ) ) torch_save(net, paste0( "model_", epoch, "_", round(mean(train_loss), 3), "_", round(mean(valid_loss), 3), ".pt" )) }
When I ran this, performance on the training set decreased in a not-too-fast, but continuous way, while validation set performance kept fluctuating. For reference, total (composite) losses looked like this:
Epoch Training Validation 10 0.336 0.633 20 0.233 0.295 30 0.135 0.461 40 0.099 0.903 50 0.061 0.727
Thinking of the size of the validation set – thirty-one years, or equivalently, 372 data points – those fluctuations may not be all too surprising.
Predictions
Now losses tend to be abstract; let’s see what actually gets predicted. We obtain predictions for index values and phases like so …
net$eval() pred_index <- c() pred_phase <- c() coro::loop(for (b in valid_dl) { output <- net(b$x$to(device = device)) pred_index <- c(pred_index, output[[2]]$to(device = "cpu")) pred_phase <- rbind(pred_phase, as.matrix(output[[3]]$to(device = "cpu"))) })
… and combine these with the ground truth, stripping off the first..