Posted by Cibu Johny, Software Engineer, Google Research and Saumya Dalal, Product Manager, Google Geo
Nearly 75% of India’s population — which possesses the second highest number of internet users in the world — interacts with the web primarily using Indian languages, rather than English. Over the next five years, that number is expected to rise to 90%. In order to make Google Maps as accessible as possible to the next billion users, it must allow people to use it in their preferred language, enabling them to explore anywhere in the world.
However, the names of most Indian places of interest (POIs) in Google Maps are not generally available in the native scripts of the languages of India. These names are often in English and may be combined with acronyms based on the Latin script, as well as Indian language words and names. Addressing such mixed-language representations requires a transliteration system that maps characters from one script to another, based on the source and target languages, while accounting for the phonetic properties of the words as well.
For example, consider a user in Ahmedabad, Gujarat, who is looking for a nearby hospital, KD Hospital. They issue the search query, કેડી હોસ્પિટલ, in the native script of Gujarati, the 6th most widely spoken language in India. Here, કેડી (“kay-dee”) is the sounding out of the acronym KD, and હોસ્પિટલ is “hospital”. In this search, Google Maps knows to look for hospitals, but it doesn’t understand that કેડી is KD, hence it finds another hospital, CIMS. As a consequence of the relative sparsity of names available in the Gujarati script for places of interest (POIs) in India, instead of their desired result, the user is shown a result that is further away.
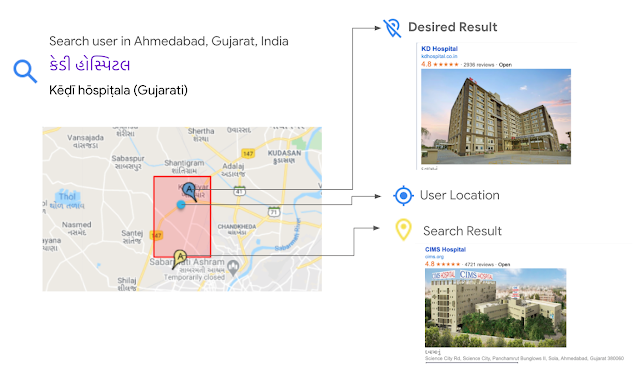
To address this challenge, we have built an ensemble of learned models to transliterate names of Latin script POIs into 10 languages prominent in India: Hindi, Bangla, Marathi, Telugu, Tamil, Gujarati, Kannada, Malayalam, Punjabi, and Odia. Using this ensemble, we have added names in these languages to millions of POIs in India, increasing the coverage nearly twenty-fold in some languages. This will immediately benefit millions of existing Indian users who don’t speak English, enabling them to find doctors, hospitals, grocery stores, banks, bus stops, train stations and other essential services in their own language.
Transliteration vs. Transcription vs. Translation
Our goal was to design a system that will transliterate from a reference Latin script name into the scripts and orthographies native to the above-mentioned languages. For example, the Devanagari script is the native script for both Hindi and Marathi (the language native to Nagpur, Maharashtra). Transliterating the Latin script names for NIT Garden and Chandramani Garden, both POIs in Nagpur, results in एनआईटी गार्डन and चंद्रमणी गार्डन, respectively, depending on the specific language’s orthography in that script.
It is important to note that the transliterated POI names are not translations. Transliteration is only concerned with writing the same words in a different script, much like an English language newspaper might choose to write the name Горбачёв from the Cyrillic script as “Gorbachev” for their readers who do not read the Cyrillic script. For example, the second word in both of the transliterated POI names above is still pronounced “garden”, and the second word of the Gujarati example earlier is still “hospital” — they remain the English words “garden” and “hospital”, just written in the other script. Indeed, common English words are frequently used in POI names in India, even when written in the native script. How the name is written in these scripts is largely driven by its pronunciation; so एनआईटी from the acronym NIT is pronounced “en-aye-tee”, not as the English word “nit”. Knowing that NIT is a common acronym from the region is one piece of evidence that can be used when deriving the correct transliteration.
Note also that, while we use the term transliteration, following convention in the NLP community for mapping directly between writing systems, romanization in South Asian languages regardless of the script is generally pronunciation-driven, and hence one could call these methods transcription rather than transliteration. The task remains, however, mapping between scripts, since pronunciation is only relatively coarsely captured in the Latin script for these languages, and there remain many script-specific correspondences that must be accounted for. This, coupled with the lack of standard spelling in the Latin script and the resulting variability, is what makes the task challenging.
Transliteration Ensemble
We use an ensemble of models to automatically transliterate from the reference Latin script name (such as NIT Garden or Chandramani Garden) into the scripts and orthographies native to the above-mentioned languages. Candidate transliterations are derived from a pair of sequence-to-sequence (seq2seq) models. One is a finite-state model for general text transliteration, trained in a manner similar to models used by Gboard on-device for transliteration keyboards. The other is a neural long short-term memory (LSTM) model trained, in part, on the publicly released Dakshina dataset. This dataset contains Latin and native script data drawn from Wikipedia in 12 South Asian languages, including all but one of the languages mentioned above, and permits training and evaluation of various transliteration methods. Because the two models have such different characteristics, together they produce a greater variety of transliteration candidates.
To deal with the tricky phenomena of acronyms (such as the “NIT” and “KD” examples above), we developed a specialized transliteration module that generates additional candidate transliterations for these cases.
For each native language script, the ensemble makes use of specialized romanization dictionaries of varying provenance that are tailored for place names, proper names, or common words. Examples of such romanization dictionaries are found in the Dakshina dataset.
Scoring in the Ensemble
The ensemble combines scores for the possible transliterations in a weighted mixture, the parameters of which are tuned specifically for POI name accuracy using small targeted development sets for such names.
For each native script token in candidate transliterations, the ensemble also weights the result according to its frequency in a very large sample of on-line text. Additional candidate scoring is based on a deterministic romanization approach derived from the ISO 15919 romanization standard, which maps each native script token to a unique Latin script string. This string allows the ensemble to track certain key correspondences when compared to the original Latin script token being transliterated, even though the ISO-derived mapping itself does not always perfectly correspond to how the given native script word is typically written in the Latin script.
In aggregate, these many moving parts provide substantially higher quality transliterations than possible for any of the individual methods alone.
Coverage
The following table provides the per-language quality and coverage improvements due to the ensemble over existing automatic transliterations of POI names. The coverage improvement measures the increase in items for which an automatic transliteration has been made available. Quality improvement measures the ratio of updated transliterations that were judged to be improvements versus those that were judged to be inferior to existing automatic transliterations.
|
Coverage |
Quality |
Language |
Improvement |
Improvement |
Hindi |
3.2x |
1.8x |
Bengali |
19x |
3.3x |
Marathi |
19x |
2.9x |
Telugu |
3.9x |
2.6x |
Tamil |
19x |
3.6x |
Gujarati |
19x |
2.5x |
Kannada |
24x |
2.3x |
Malayalam |
24x |
1.7x |
Odia |
960x |
—* |
Punjabi |
24x |
—* |
Conclusion
As with any machine learned system, the resulting automatic transliterations may contain a few errors or infelicities, but the large increase in coverage in these widely spoken languages marks a substantial expansion of the accessibility of information within Google Maps in India. Future work will include using the ensemble for transliteration of other classes of entities within Maps and its extension to other languages and scripts, including Perso-Arabic scripts, which are also commonly used in the region.
Acknowledgments
This work was a collaboration between the authors and Jacob Farner, Jonathan Herbert, Anna Katanova, Andre Lebedev, Chris Miles, Brian Roark, Anurag Sharma, Kevin Wang, Andy Wildenberg, and many others.
