![]() |
submitted by /u/-j4ckK- [visit reddit] [comments] |
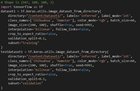
![]() |
submitted by /u/-j4ckK- [visit reddit] [comments] |
Advances in machine learning (ML) often come with advances in hardware and computing systems. For example, the growth of ML-based approaches in solving various problems in vision and language has led to the development of application-specific hardware accelerators (e.g., Google TPUs and Edge TPUs). While promising, standard procedures for designing accelerators customized towards a target application require manual effort to devise a reasonably accurate simulator of hardware, followed by performing many time-intensive simulations to optimize the desired objective (e.g., optimizing for low power usage or latency when running a particular application). This involves identifying the right balance between total amount of compute and memory resources and communication bandwidth under various design constraints, such as the requirement to meet an upper bound on chip area usage and peak power. However, designing accelerators that meet these design constraints is often result in infeasible designs. To address these challenges, we ask: “Is it possible to train an expressive deep neural network model on large amounts of existing accelerator data and then use the learned model to architect future generations of specialized accelerators, eliminating the need for computationally expensive hardware simulations?”
In “Data-Driven Offline Optimization for Architecting Hardware Accelerators”, accepted at ICLR 2022, we introduce PRIME, an approach focused on architecting accelerators based on data-driven optimization that only utilizes existing logged data (e.g., data leftover from traditional accelerator design efforts), consisting of accelerator designs and their corresponding performance metrics (e.g., latency, power, etc) to architect hardware accelerators without any further hardware simulation. This alleviates the need to run time-consuming simulations and enables reuse of data from past experiments, even when the set of target applications changes (e.g., an ML model for vision, language, or other objective), and even for unseen but related applications to the training set, in a zero-shot fashion. PRIME can be trained on data from prior simulations, a database of actually fabricated accelerators, and also a database of infeasible or failed accelerator designs1. This approach for architecting accelerators — tailored towards both single- and multi-applications — improves performance upon state-of-the-art simulation-driven methods by about 1.2x-1.5x, while considerably reducing the required total simulation time by 93% and 99%, respectively. PRIME also architects effective accelerators for unseen applications in a zero-shot setting, outperforming simulation-based methods by 1.26x.
The PRIME Approach for Architecting Accelerators
Perhaps the simplest possible way to use a database of previously designed accelerators for hardware design is to use supervised machine learning to train a prediction model that can predict the performance objective for a given accelerator as input. Then, one could potentially design new accelerators by optimizing the performance output of this learned model with respect to the input accelerator design. Such an approach is known as model-based optimization. However, this simple approach has a key limitation: it assumes that the prediction model can accurately predict the cost for every accelerator that we might encounter during optimization! It is well established that most prediction models trained via supervised learning misclassify adversarial examples that “fool” the learned model into predicting incorrect values. Similarly, it has been shown that even optimizing the output of a supervised model finds adversarial examples that look promising under the learned model2, but perform terribly under the ground truth objective.
To address this limitation, PRIME learns a robust prediction model that is not prone to being fooled by adversarial examples (that we will describe shortly), which would be otherwise found during optimization. One can then simply optimize this model using any standard optimizer to architect simulators. More importantly, unlike prior methods, PRIME can also utilize existing databases of infeasible accelerators to learn what not to design. This is done by augmenting the supervised training of the learned model with additional loss terms that specifically penalize the value of the learned model on the infeasible accelerator designs and adversarial examples during training. This approach resembles a form of adversarial training.
In principle, one of the central benefits of a data-driven approach is that it should enable learning highly expressive and generalist models of the optimization objective that generalize over target applications, while also potentially being effective for new unseen applications for which a designer has never attempted to optimize accelerators. To train PRIME so that it generalizes to unseen applications, we modify the learned model to be conditioned on a context vector that identifies a given neural net application we wish to accelerate (as we discuss in our experiments below, we choose to use high-level features of the target application: such as number of feed-forward layers, number of convolutional layers, total parameters, etc. to serve as the context), and train a single, large model on accelerator data for all applications designers have seen so far. As we will discuss below in our results, this contextual modification of PRIME enables it to optimize accelerators both for multiple, simultaneous applications and new unseen applications in a zero-shot fashion.
Does PRIME Outperform Custom-Engineered Accelerators?
We evaluate PRIME on a variety of actual accelerator design tasks. We start by comparing the optimized accelerator design architected by PRIME targeted towards nine applications to the manually optimized EdgeTPU design. EdgeTPU accelerators are primarily optimized towards running applications in image classification, particularly MobileNetV2, MobileNetV3 and MobileNetEdge. Our goal is to check if PRIME can design an accelerator that attains a lower latency than a baseline EdgeTPU accelerator3, while also constraining the chip area to be under 27 mm2 (the default for the EdgeTPU accelerator). Shown below, we find that PRIME improves latency over EdgeTPU by 2.69x (up to 11.84x in t-RNN Enc), while also reducing the chip area usage by 1.50x (up to 2.28x in MobileNetV3), even though it was never trained to reduce chip area! Even on the MobileNet image-classification models, for which the custom-engineered EdgeTPU accelerator was optimized, PRIME improves latency by 1.85x.
Comparing latencies (lower is better) of accelerator designs suggested by PRIME and EdgeTPU for single-model specialization. |
The chip area (lower is better) reduction compared to a baseline EdgeTPU design for single-model specialization. |
Designing Accelerators for New and Multiple Applications, Zero-Shot
We now study how PRIME can use logged accelerator data to design accelerators for (1) multiple applications, where we optimize PRIME to design a single accelerator that works well across multiple applications simultaneously, and in a (2) zero-shot setting, where PRIME must generate an accelerator for new unseen application(s) without training on any data from such applications. In both settings, we train the contextual version of PRIME, conditioned on context vectors identifying the target applications and then optimize the learned model to obtain the final accelerator. We find that PRIME outperforms the best simulator-driven approach in both settings, even when very limited data is provided for training for a given application but many applications are available. Specifically in the zero-shot setting, PRIME outperforms the best simulator-driven method we compared to, attaining a reduction of 1.26x in latency. Further, the difference in performance increases as the number of training applications increases.
The average latency (lower is better) of test applications under zero-shot setting compared to a state-of-the-art simulator-driven approach. The text on top of each bar shows the set of training applications. |
Closely Analyzing an Accelerator Designed by PRIME
To provide more insight to hardware architecture, we examine the best accelerator designed by PRIME and compare it to the best accelerator found by the simulator-driven approach. We consider the setting where we need to jointly optimize the accelerator for all nine applications, MobileNetEdge, MobileNetV2, MobileNetV3, M4, M5, M64, t-RNN Dec, and t-RNN Enc, and U-Net, under a chip area constraint of 100 mm2. We find that PRIME improves latency by 1.35x over the simulator-driven approach.
Per application latency (lower is better) for the best accelerator design suggested by PRIME and state-of-the-art simulator-driven approach for a multi-task accelerator design. PRIME reduces the average latency across all nine applications by 1.35x over the simulator-driven method. |
As shown above, while the latency of the accelerator designed by PRIME for MobileNetEdge, MobileNetV2, MobileNetV3, M4, t-RNN Dec, and t-RNN Enc are better, the accelerator found by the simulation-driven approach yields a lower latency in M5, M6, and U-Net. By closely inspecting the accelerator configurations, we find that PRIME trades compute (64 cores for PRIME vs. 128 cores for the simulator-driven approach) for larger Processing Element (PE) memory size (2,097,152 bytes vs. 1,048,576 bytes). These results show that PRIME favors PE memory size to accommodate the larger memory requirements in t-RNN Dec and t-RNN Enc, where large reductions in latency were possible. Under a fixed area budget, favoring larger on-chip memory comes at the expense of lower compute power in the accelerator. This reduction in the accelerator’s compute power leads to higher latency for the models with large numbers of compute operations, namely M5, M6, and U-Net.
Conclusion
The efficacy of PRIME highlights the potential for utilizing the logged offline data in an accelerator design pipeline. A likely avenue for future work is to scale this approach across an array of applications, where we expect to see larger gains because simulator-driven approaches would need to solve a complex optimization problem, akin to searching for needle in a haystack, whereas PRIME can benefit from generalization of the surrogate model. On the other hand, we would also note that PRIME outperforms prior simulator-driven methods we utilize and this makes it a promising candidate to be used within a simulator-driven method. More generally, training a strong offline optimization algorithm on offline datasets of low-performing designs can be a highly effective ingredient in at the very least, kickstarting hardware design, versus throwing out prior data. Finally, given the generality of PRIME, we hope to use it for hardware-software co-design, which exhibits a large search space but plenty of opportunity for generalization. We have also released both the code for training PRIME and the dataset of accelerators.
Acknowledgments
We thank our co-authors Sergey Levine, Kevin Swersky, and Milad Hashemi for their advice, thoughts and suggestions. We thank James Laudon, Cliff Young, Ravi Narayanaswami, Berkin Akin, Sheng-Chun Kao, Samira Khan, Suvinay Subramanian, Stella Aslibekyan, Christof Angermueller, and Olga Wichrowskafor for their help and support, and Sergey Levine for feedback on this blog post. In addition, we would like to extend our gratitude to the members of “Learn to Design Accelerators”, “EdgeTPU”, and the Vizier team for providing invaluable feedback and suggestions. We would also like to thank Tom Small for the animated figure used in this post.
1The infeasible accelerator designs stem from build errors in silicon or compilation/mapping failures. ↩
2This is akin to adversarial examples in supervised learning – these examples are close to the data points observed in the training dataset, but are misclassified by the classifier. ↩
3The performance metrics for the baseline EdgeTPU accelerator are extracted from an industry-based hardware simulator tuned to match the performance of the actual hardware. ↩
4These are proprietary object-detection models, and we refer to them as M4 (indicating Model 4), M5, and M6 in the paper. ↩
Sign up now for DLI workshops at GTC and learn new technical skills from top experts across a range of fields including NLP, data science, deep learning, and more.
Are you looking to grow your technical skills with hands-on, instructor-led training? The NVIDIA Deep Learning Institute (DLI) is offering full-day workshops at NVIDIA GTC, March 21-24. Register for a workshop and learn how to create cutting-edge GPU-accelerated applications in AI, data science, or accelerated computing.
Each hands-on session gives you access to a fully configured GPU-accelerated server in the cloud, while you work with an instructor. Along with learning new skills, you will also earn an NVIDIA Deep Learning Institute certificate of subject matter competency, which can easily be added to your LinkedIn profile.
During GTC, all DLI workshops are offered at a special price of $149 (normally $500/seat.) NVIDIA Certified Instructors, who are technical experts in their fields, will be leading all eight DLI workshops.
Below are three subject matter experts looking forward to working with you next week.
David joined NVIDIA 3 years ago as a Senior Solutions Architect (SA) and plays a key role in a variety of strategic Data Science initiatives. He recently transitioned to the NVIDIA Inception Program teams, where he helps startup companies disrupt their market with accelerated computing and AI. He also completed a rotational assignment with the NVIDIA AI Applications team to develop better language models for neural machine translation. David’s technical specialty is NLP applications with GPU-accelerated SDKs.
“It happens pretty often that I’m approached by a client with a fantastic idea for a deep learning (DL) application but who isn’t quite sure how to get started. That’s one of the reasons I enjoy teaching the Fundamentals of Deep Learning course. I know I’m enabling people to do their best work and create something novel with DL. Each time we approach the end of a full day of hands-on labs, I can sense the ‘a-ha!’ moment as students learn how to use our SDKs and practice training DL models. When they enthusiastically want to take their finished code and final project home with them, I’m so pleased they’ll have a starting point for their idea!”
David earned a BS in Electrical Engineering from the University of Illinois Urbana-Champaign, and an MS from National Technological University. Before NVIDIA, he spent 25 years at Motorola and the Johns Hopkins Applied Physics Laboratory.
David is teaching the Fundamentals of Deep Learning workshop at GTC.
As a Solutions Architect on the Financial Services team, David is helping payment companies adopt GPUs and AI. He teaches workshops focused on NLP applications, using models critical to understanding customer and market behavior. According to David, the wide-ranging use cases of NLP also provide an amazing opportunity to learn from students in completely distinct fields from the one he spends time with in his ‘day job.’
“I have a passion for teaching, which comes from both the joy of seeing others learn as well as the interaction with such a diverse range of students. DLI provides a great environment to lay the vital foundation in complex topics like deep learning and CUDA programming. I hope participants in my classes can easily grasp the most important concepts, no matter the level they came in at. Leading workshops at GTC, customer sites, and even internally at NVIDIA, introduces me to such varied backgrounds and experiences, my teaching provides me an opportunity to learn as well.”
David earned both a BS and MS in Computer Engineering from Northwestern University.
He is teaching the Building Transformer Based Natural Language Processing Applications workshop at GTC.
Along with being a Solutions Architect, Gunter manages many of the platform admin tasks for Europe, the Middle East, and Africa (EMEA) DLI workshops. While in that role he is actively spinning up GPU instances, minimizing downtime time for students and helping them get to work in their notebooks quickly.
“From my first day at NVIDIA, it was clear that teaching courses through DLI would be a simple, but highly efficient way to scale technical training for developers. Teaching the basics of CUDA or deep learning in rooms packed with eager students is certainly not always easy, but is a satisfying and rewarding experience. Using notebooks in the cloud helps students focus on the class, instead of spending time with CUDA drivers and SDK installation.”
He has a Master’s degree in geophysics from the Institut de Physique du Globe, in Paris and a PhD in seismology on the use of neural networks for interpreting geophysical data.
Gunter is certified to teach five DLI courses, and will be helping teach the Applications of AI for Predictive Maintenance workshop at GTC.
For a complete list of the 25 instructor-led workshops at GTC, visit the GTC Session Catalog. Several workshops are available in Taiwanese, Korean, and Japanese for attendees in their respective time zones.
It’s never been easier to be a PC gamer. GeForce NOW is your gateway into PC gaming. With the power of NVIDIA GeForce GPUs in the cloud, any gamer can stream titles from the top digital games stores — even on low-powered hardware. Evolve to the PC gaming ranks this GFN Thursday and get ready Read article >
The post Everyone’s a PC Gamer This GFN Thursday appeared first on NVIDIA Blog.
Hi Tensorflow Experts,
I have been working on two codes, one in TF1, the other one in TF2. I did some research about the TensorFlow architecture the difference between these two, maybe you could check if my understanding is correct?
In version 1, graphs need to be created manually by the user. In version 2, the API has been made more user-friendly and the graph creation is now automated in Keras. Has Keras been created explicitly for TensorFlow 2, or does it exist independently from it?
The eager execution mode basically breaks the graph approach to create a more “classical” computation scheme. It is used per default in pure high-level TensorFlow, since here the computations have a smaller impact on performance. On the other hand, in Keras, eager mode is switched off and behaves more or less like TF1. Is this correct?
submitted by /u/ThoughtfulTopQuark
[visit reddit] [comments]
I have extracted audio embeddings from Google Audioset corpus.
Now, I want to use these audio embeddings for training my own model (CNN). I have some confusion about these audio embeddings.
Also, please share some helpful resources about working with Google audioset corpus if possible.
submitted by /u/sab_1120
[visit reddit] [comments]
I am trying to build a program that will classify objects, and I want my clients to be able to add extra objects freely. However, from my knowledge, this requires the retraining of the entire neural network, and this is very expensive.
Is there a network where we would be able to add more options to the image classifier without retraining or little training?
submitted by /u/Unwantediosuser
[visit reddit] [comments]
Partnering with NVIDIA and the ICC, Photon Commerce is creating the world’s most intelligent financial AI platform for instant B2B payments, invoices, statements, and receipts.
The business-to-business (B2B) payments ecosystem is massive, with $25 trillion in payments flowing between businesses each year. Photon Commerce, a financial AI platform company, empowers fintech leaders to process B2B payments, invoices, statements, and receipts instantly.
Over two-thirds of B2B transactions are processed through automated clearing house payments (a type of electronic payment) and checks. Yet, these transactions can take up to 3 days to clear. This has created a need for real-time payments that are processed instantaneously and safely, eliminating the risk of delinquent payments.
Partnering with NVIDIA and the International Chamber of Commerce, Photon Commerce guides payment processors, neobanks, and credit card fintechs on how to train and invent the world’s most intelligent AI for payments, invoices, and commerce.
Why is the use of AI crucial in payment processing? Card-not-present payments, such as those made online or over the phone, are costly for merchants, requiring manual entry and approval. AI-powered payments also work remotely but are instantaneous and secure.
Additionally, two out of three merchants today do not accept credit cards due to fees. Not even Amazon is willing to pay these expenses at times. The solution lies in real-time payments and request-for-payment offerings. These low-cost payment systems provide fraud-free payment options for 30 million merchants in the US.
Photon’s AI offers one-click bill pay for customers’ credit card lenders and leading core payment processors. These entities handle trillions of dollars in payments for the majority of banks and merchants.
One such customer, Settle is a leader in receivables finance, payables finance, and bill pay for eCommerce merchants like Italic, Huron, Brightland, and Branch.
Pioneering a Buy-Now-Pay-Later solution for B2B and eCommerce merchants, Settle Founder and CEO, Alek Koenig claims Photon’s invoice automation technology is a ‘godsend.’
“Photon’s solution enabled us to improve user experience, capture greater revenues, and significantly reduce manual keying of invoice and payment data. Before Photon, we were just typing up each invoice manually,” said Koenig.
Settle’s AI-based financial services and solutions achieved meteoric growth, especially among small to midsized businesses. The company raised nearly $100M from top-tier investors, such as Kleiner Perkins, within only 2 years of its inception.
AI accelerated processing forms the underpinning of Photon Commerce’s AI solution capable of tackling unstructured or semistructured data and serving their customers. Photon’s base models for enterprise workloads start with 16 NVIDIA V100 GPUs. Depending on throughput, bandwidth, and power factors, Photon’s deep learning machines readily scale to 64 NVIDIA V100 GPUs or more.
GPU-accelerated computing has been critical to Photon’s machine learning models, both for training and inference. Photon’s deep learning was trained on NVIDIA V100s, providing 55x faster performance than CPU servers and 24x faster performance during inference.
Custom development and production boxes or clusters are provisioned either in the cloud, hybrid cloud, or on-prem deployments. Docker containers use Kubernetes to provide container orchestration across clusters during the scaling of models. Photon’s API architecture runs through a data pipeline of file validation, document classification, computer vision, then NLP. Photon’s NLP transformer models are autoregressive in architecture, employing model and data parallelism.
AI payment solution concepts are key to enabling end-to-end traceability, visibility, and scaling to high-transaction volumes needed for eCommerce merchants, and logistics companies for trade finance solutions.
Below are three examples of Photon’s AI solutions improving payment systems.
The value of extracting information from documents, particularly in the context of finance, for unstructured and semistructured data is enormous. Companies and individuals can process invoices, receipts, and forms with little to no-human interaction, saving time and money. Photon Commerce’s AI technology solves this problem by automatically reading, understanding, approving, and paying any invoice using computer vision and NLP.
Business documents are messy and each company has different Enterprise resource planning systems, record portals, and formats. These systems often break down with disputes, errors, and fraud happening daily. Photon Commerce’s solutions standardize any invoice, bill, or payment document in the world, regardless of language or format. This facilitates instant approvals, payments, and straight-through-processing.
Businesses and trade partners can now speak the same language. Photon’s NLP understands that a “vendor”, “supplier”, “seller”, “beneficiary”, “merchant”, and “卖方” are generally synonyms, referring to the same “object” called as Named Entity Recognition. Photon’s reconciliation AI can instantly flip any purchase order into an invoice, or match purchase orders, invoices, receipts, remittances, shipping labels, bills-of-lading, proof-of-deliveries, and rate confirmations seamlessly together.
Reach out to nvidia@photoncommerce.com and learn more about how AI accelerates payments, invoicing, and trade collaboration between businesses.
Hi all,
I’m tuning the hyperparameters of a Tensorflow model in Google AI platform, but I have the following problem: For the evaluation metric I want to optimize, it seems like only the metric value at the end of the training is reported to the hyperparameter optimizer, instead of the best value achieved during training. Is it possible to create a metric, which will track the maximum value over the entire training?
submitted by /u/CodeAllDay1337
[visit reddit] [comments]
Hey. I’m having this error with the import of the package below:
ModuleNotFoundError: No module named ‘object_detection.utils’; ‘object_detection’ is not a package
All help is appreciated
submitted by /u/dalpendre
[visit reddit] [comments]