![]() |
submitted by /u/1991viet [visit reddit] [comments] |
Trying to run GPT-2-master, getting error
Hi there! im very new at this so if i’ve left anything out please let me know!
so far ive downloaded the file, used cd to get into it and then have done this:
conda create -n py36 python=3.6 anaconda pip3 install tensorflow==1.12.0 pip3 install -r requirements.txt python3 download_model.py 124M
and upon running
python3 src/interactive_conditional_samples.py --top_k 40
Anaconda returns
C:ProgramDataAnaconda3envspy36libsite-packagestensorflowpythonframeworkdtypes.py:523: FutureWarning: Passing (type, 1) or '1type' as a synonym of type is deprecated; in a future version of numpy, it will be understood as (type, (1,)) / '(1,)type'. _np_qint8 = np.dtype([("qint8", np.int8, 1)]) C:ProgramDataAnaconda3envspy36libsite-packagestensorflowpythonframeworkdtypes.py:524: FutureWarning: Passing (type, 1) or '1type' as a synonym of type is deprecated; in a future version of numpy, it will be understood as (type, (1,)) / '(1,)type'. _np_quint8 = np.dtype([("quint8", np.uint8, 1)]) C:ProgramDataAnaconda3envspy36libsite-packagestensorflowpythonframeworkdtypes.py:525: FutureWarning: Passing (type, 1) or '1type' as a synonym of type is deprecated; in a future version of numpy, it will be understood as (type, (1,)) / '(1,)type'. _np_qint16 = np.dtype([("qint16", np.int16, 1)]) C:ProgramDataAnaconda3envspy36libsite-packagestensorflowpythonframeworkdtypes.py:526: FutureWarning: Passing (type, 1) or '1type' as a synonym of type is deprecated; in a future version of numpy, it will be understood as (type, (1,)) / '(1,)type'. _np_quint16 = np.dtype([("quint16", np.uint16, 1)]) C:ProgramDataAnaconda3envspy36libsite-packagestensorflowpythonframeworkdtypes.py:527: FutureWarning: Passing (type, 1) or '1type' as a synonym of type is deprecated; in a future version of numpy, it will be understood as (type, (1,)) / '(1,)type'. _np_qint32 = np.dtype([("qint32", np.int32, 1)]) C:ProgramDataAnaconda3envspy36libsite-packagestensorflowpythonframeworkdtypes.py:532: FutureWarning: Passing (type, 1) or '1type' as a synonym of type is deprecated; in a future version of numpy, it will be understood as (type, (1,)) / '(1,)type'. np_resource = np.dtype([("resource", np.ubyte, 1)]) 2021-03-15 16:39:25.892397: I tensorflow/core/platform/cpu_feature_guard.cc:141] Your CPU supports instructions that this TensorFlow binary was not compiled to use: AVX2 Traceback (most recent call last): File "src/interactive_conditional_samples.py", line 91, in <module> fire.Fire(interact_model) File "C:ProgramDataAnaconda3envspy36libsite-packagesfirecore.py", line 141, in Fire component_trace = _Fire(component, args, parsed_flag_args, context, name) File "C:ProgramDataAnaconda3envspy36libsite-packagesfirecore.py", line 471, in _Fire target=component.__name__) File "C:ProgramDataAnaconda3envspy36libsite-packagesfirecore.py", line 681, in _CallAndUpdateTrace component = fn(*varargs, **kwargs) File "src/interactive_conditional_samples.py", line 65, in interact_model temperature=temperature, top_k=top_k, top_p=top_p File "C:UserslouisOneDriveDesktopgpt-2-testsrcsample.py", line 74, in sample_sequence past, prev, output = body(None, context, context) File "C:UserslouisOneDriveDesktopgpt-2-testsrcsample.py", line 66, in body logits = top_p_logits(logits, p=top_p) File "C:UserslouisOneDriveDesktopgpt-2-testsrcsample.py", line 28, in top_p_logits sorted_logits = tf.sort(logits, direction='DESCENDING', axis=-1) AttributeError: module 'tensorflow' has no attribute 'sort'
I have no idea what any of this means. if someone could help me out it would mean a lot! đ
submitted by /u/LouisHendrich
[visit reddit] [comments]
Call it Moneyball for deep learning. New York Times writer Cade Metz tells the funny, inspiring â and ultimately triumphant â tale of how a dogged group of AI researchers bet their careers on the long-dismissed technology of deep learning. The AI Podcast · Author Cade Metz Talks About His New Book âGenius Makersâ â Read article >
The post In âGenius Makersâ Cade Metz Tells Tale of Those Behind the Unlikely Rise of Modern AI appeared first on The Official NVIDIA Blog.
The world of bicycle racing has changed. Aggressive cyclists expect their bikes to meet their every need, no matter how detailed and precise. And meeting these needs requires an entirely new approach. Predator Cycling engineers and manufactures high-end custom-built carbon fiber bicycles that have garnered praise from championship cyclists around the world. For the past Read article >
The post Racing Ahead, Predator Cycling Speeds Design and Development of Custom Bikes with Real-Time Rendering appeared first on The Official NVIDIA Blog.
As GeForce NOW enters its second year and rapidly approaches 10 million members, weâre setting our sights on fresh milestones and adding new membership offerings. First up is a new premium offering, Priority membership, which receives the same benefits as Founders members. These include priority access to gaming sessions, extended session lengths and RTX ON Read article >
The post GeForce NOW Gets New Priority Memberships and More appeared first on The Official NVIDIA Blog.
This month we spotlight Lokman Abbas Turki, lecturer and researcher at Sorbonne University in Paris, France.
âMeet the Researcherâ is a monthly series in which we spotlight researchers who are using GPUs to accelerate their work. This month we spotlight Lokman Abbas Turki, lecturer and researcher at Sorbonne University in Paris, France.
What area of research is your lab focused on?
If I had to sum it up in a few words, I would say computer science probability. More precisely, I apply probability and high performance computing to computationally complex problems in mathematical finance, and recently to increase the resilience of asymmetric cryptosystems against side-channel attacks. Quantitative (mathematical) finance has the desirable property of usually using Monte Carlo-based methods which are most suited to parallel architecture. Besides, studying numerically the resilience of cryptosystems is only satisfactory when conducted at a large-scale and thus has to be efficiently distributed on parallel machines.
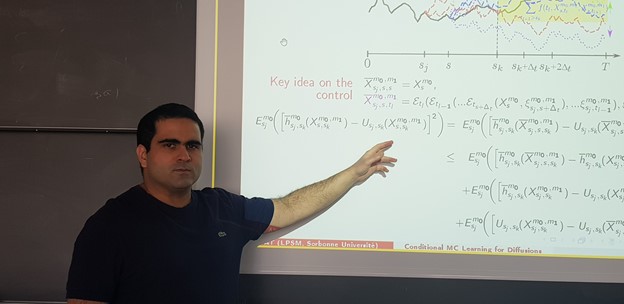
What motivated you to pursue this research area?
It originally started during my engineering studies in signal processing at SupĂ©lec (a French graduate school of engineering). I was impressed by the beauty of some mathematical results and the technicalities of their implementation on machines, especially the work of Young Turks at Bell Labs that included famous names like Claude Shannon, Richard Hamming, and John Tukey. Then, during my Masterâs degree in Mathematics 2007/2008, I was fortunate enough to work at CERAMICS research center of Ăcole des Ponts on the parallelization of finance simulations on GPUs. I originally started using CG shading language then CUDA on one GPU, then CUDA+OpenMP+MPI on a cluster of GPUs.
Once I learned how to program on GPUs and had significant speedup, I could not quit using them. Also, I was convinced that the gaming community cannot stop getting bigger which ensures that GPUs are here to stay, unlike other co-processors. As a result, I continued my research in probability with a special focus on the scalability of the proposed methods on massively parallel architectures.
Tell us about a few of your current research projects.
I usually find myself involved in different projects that allow me to have continuous collaborations with colleagues in both Mathematics and Computer Science. For example, until last year I participated in the ANR project ARRAND studying the impact of randomization in asymmetric cryptography. Since 2016, I nurture various collaborations with CrĂ©dit Agricole in probability/high performance computing/deep learning applied to finance. Some resulting codes are provided to Premia consortium project led by INRIA. Since September 2020, I also started participating in the chair Stress Testing established between Ăcole Polytechnique and BNP Paribas.
What problems or challenges does your research address?
In the last two years, I spent the main part of my research on the following problems:
In asymmetric cryptography, as the continuation of the work [1], my Ph.D. student and I studied a sophisticated version of Template attacks based on a relaxed conditional maximum likelihood estimator.
In the Monte Carlo simulation of nonlinear parabolic PDEs (Partial Differential Equations) [2], we present a new conditional learning method that allows us to control the bias.
In high performance computing, as the continuation of the work [3], we are gradually improving and extending the batch parallel divide and conquer algorithm for eigenvalues.
In deep learning [4], we show a new method to reduce the variance of the loss estimator and thus accelerate the convergence to the optimal choice of the network parameters.
The solution of problem 3 is used in problems 1 and 2. The solution to problem 4 allows unconditional learning presented in problem 2.
What is the (expected) impact of your work on the field/community/world?
In research, I really like being at the interface of various disciplines. Although very involved, I contribute to generating collaborations between colleagues specialized in different areas. For example, at Sorbonne Université, I have various collaborations with members from the probability/statistics laboratory LPSM and also with members from computer science research institute LIP6. In both applied mathematics and computer science communities, I defend the fact that the old sequential deterministic methods are becoming less and less relevant because of the massively parallel architecture that we have today and their democratization through public cloud computing. As alternatives, I argue for the use of stochastic methods based on Monte Carlo that is more scalable and can be more naturally combined with neural networks.
In teaching activities, my âProgramming GPUsâ course is a meaningful success since it allows me to explain the new paradigms of parallelization to about 150 students/researchers each year coming from various specialties in mathematics and computer science. Among all my courses, âProgramming GPUsâ is the one that evolved the most. Started 9 years ago, its first version was an introduction to CUDA then it changed into the use of GPUs for parabolic PDEs (Partial Differential Equations) simulation. The current version is rather dedicated to batch parallel processing and will soon include elements from deep learning.
What technological breakthroughs are you most proud of from your work?
In the various collaborations I had, we were able to push the envelope a bit further. For example, for parabolic PDEs in [2], we simulate and control the bias of very complicated high-dimensional problems that cannot be efficiently simulated using other methods. It is worth mentioning that the very competitive execution times that we present in [2] are possible because of the parallelization of the CUDA of the batch processing strategies presented in [3]. Regarding contribution [4] in which we use deep learning with an inexpensive over-simulation trick, we are first to propose a training that converges (cf. flash video) for a very high dimensional problem of CVA (Credit Valuation Adjustment) simulation.
How have you used NVIDIA technology either in your current or previous research?Â
An efficient CUDA parallelization of my codes on NVIDIA GPUs provides me the computing power that speeds up the critical parts of my algorithms by a factor that always exceeds 20 even when compared to a vectorization using AVX on CPUs. The speedup exceeds sometimes 200 for embarrassingly parallel highly caching operations. Consequently, each algorithm that I write or that I supervise is automatically implemented using either C++/CUDA or Python/CUDA.
Whatâs next for your research?
In the chair Stress Testing, I will be involved in exploring new methods for hedging risks of extreme events. The majority of current methods for high quantiles computation are essentially sequential. With a colleague at LISN (Laboratoire Interdisciplinaire des Sciences du Numérique), we already started exploring a new parallel promising method that trains NNs (Neural Networks) using nested Monte Carlo. In the numerical probability community, the use of NNs with Monte Carlo is becoming a standard. However, the current contributions implement NNs for their ability to provide a solution to complex problems and not for their genericity to be reused by other applications (automation) and for a large variety of data (scalability). Automation and scalability are key features in AI that help to overcome the difficulty of high quantiles computation.
Any advice for new researchers?
Avoid working on anything that is not scalable with respect to an increasing size of data or not scalable with respect to greater computing power. Moreover, as each scalability needs either sufficient technical skills in probability/statistics or low-level programming capabilities, make sure to have an edge by mastering the latest advances in both of these fields.
References
[1] J. Courtois, L. AbbasâTurki and J.âC. Bajard (2019): Resilience of randomized RNS arithmetic with respect to side-channel leaks of cryptographic computation, IEEE Transactions on Computers, vol. 68 (12), pp. 1720-1730.
[2] L. A. Abbas-Turki, B. Diallo and G. PagĂšs (2020): Conditional Monte Carlo Learning for Diffusions I & II https://hal.archives-ouvertes.fr/hal-02959492/ & https://hal.archives-ouvertes.fr/hal-02959494/
[3] L. A. Abbas-Turki and S. Graillat (2017): Resolving small random symmetric linear systems on graphics processing units, The Journal of Supercomputing, vol. 73, pp. 1360-1386.
[4] L. A. Abbas-Turki, S. Crépey and B. Saadeddine (2020): Deep X-Valuation Adjustments (XVAs) Analysis, presented in NVIDIA GTC and QuantMinds International.
Building on the announcement at RSNA 2019, XNAT, the most widely-used open-source informatics platform for imaging research, announced the beta release of XNAT Machine Learning (XNAT ML).
XNAT, one of the most widely-used open-source informatics platforms for imaging research, announced the general release of XNAT 1.8 on March 10. Building on the announcement of XNAT ML Beta in the summer of 2020, XNAT will accelerate the creation of AI models by providing an end-to-end development platform, enabling faster collaborations between data science and clinical teams.
XNAT, in collaboration with NVIDIA, Radiologics, and the ICR Imaging Informatics group, announced the general availability of XNAT 1.8, adding support for model training and AI-assisted annotation workflows. This integration was first announced at the RSNA 2019 conference, where a proof of concept was demonstrated using models and APIs from the NVIDIA Clara Imaging framework with accelerated GPU computing.
The XNAT release introduces new capabilities with the XNAT imaging research platform, accelerated by GPUs:
- Assemble training-specific collections of imaging data files into specific training projects to build balanced data cohorts
- Draw new segmentations and annotations on that data, using NVIDIA Clara Trainâs AI-assisted annotation
- Install and configure pre-trained models from Clara Train, available through NVIDIA NGC into the XNAT ML environment
- Train models with annotated datasets using training framework provided by NVIDIA Clara TrainÂ
NVIDIA Clara Imaging is an application framework that accelerates the development and deployment of AI in medical imaging. Built for anyone building AI models, Clara Imaging offers pre-trained models, collaborative techniques to train robust AI models without sharing patient data across institutions, and end-to-end software for scalable and modular AI deployments.
Explore sessions covering topics ranging across domain-specific use cases, to the fundamentals of how GPU computing works and whatâs new in the latest developer tools.
From weather forecasting and energy exploration, to computational chemistry and molecular dynamics, NVIDIA compute and networking technologies are optimizing nearly 2,000 applications across a broad-range of scientific domains and industries. By leveraging GPU-powered parallel processing, users can accelerate advanced, large-scale applications efficiently and reliably, paving the way to scientific discovery.
Explore sessions across a variety of topics ranging across domain-specific use cases, to the fundamentals of how GPU computing works and whatâs new in the latest developer tools.
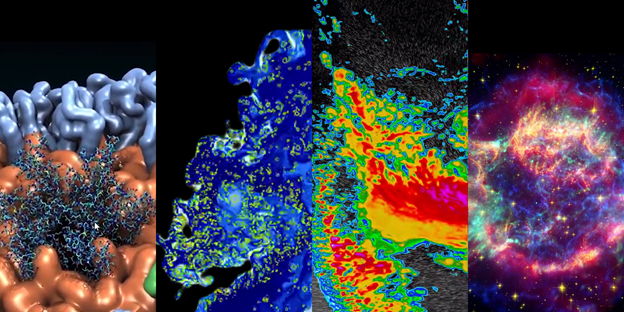
- Convergence of AI and HPC to Solve Grand Challenge Science Problems
The response to the COVID-19 pandemic poses a Grand Challenge Science problem with immediate impact for global health and well-being. Weâll discuss the award-winning work that was recognized at the recent SC20 event with the Gordon Bell for COVID competition, and provide insight into how the methods were applied, and their future plans.
Tom Gibbs, Manager, Developer Relations, NVIDIA
Rommie Amaro, Professor, UCSD
Arvind Ramanathan, Professor, ANL
James Phillips, Senior Research Programmer, University of Illinois
Lillian Chong, Associate Professor, University of Pittsburgh
Thomas Miller, CEO, Entos
- Mixed-Precision Machine Learning Method for Environmental Applications on GPUs
A primary machine learning algorithm for spatial statistics is the maximum log-likelihood estimation (MLE) function, whose central data structure is a dense covariance matrix that requires two operations: inverse and determinant evaluation. To reduce the time complexity, we migrate MLE to three-precision approximation (double/single/half) by exploiting the loss of correlation with distance. We exploit the advantages of NVIDIA Tensor Core technology to accelerate spatial modeling on large-scale soil moisture and wind speed real datasets.
Hatem Ltaief, Principal Research Scientist, KAUST
Sameh Abdulah, Research Scientist, KAUST
- Introducing Developer Tools for Arm and NVIDIA Systems
Explore the role of key tools and toolchains on Arm servers, from Arm, NVIDIA and elsewhere â and show how each tool fits in the end-to-end journey to production science and simulation.
David Lecomber, Senior Director, Arm
- Physics-Guided Deep Learning for Fluid Dynamics
This talk will demonstrate the advantages of using a Turbulent-Flow Net and Equivariant Net approach to a variety of physical systems including fluid and traffic dynamics.
Rose Yu, Assistant Professor, University of California, San Diego
- How GPU Computing Works
Come for an introduction to GPU computing by the lead architect of CUDA. Weâll walk through the internals of how the GPU works and why CUDA is the way that it is, and connect the dots between physical hardware and parallel computing.
Stephen Jones, CUDA Architect, NVIDIA
Register today for free and start building your schedule.You can also explore featured HPC sessions here and GPU programming sessions here.
AI agent plays Contra
![]() |
submitted by /u/1991viet [visit reddit] [comments] |
Trying to run GPT-2-master, getting error
Hi there! im very new at this so if i’ve left anything out please let me know!
so far ive downloaded the file, used cd to get into it and then have done this:
conda create -n py36 python=3.6 anaconda pip3 install tensorflow==1.12.0 pip3 install -r requirements.txt python3 download_model.py 124M
and upon running
python3 src/interactive_conditional_samples.py --top_k 40
Anaconda returns
C:ProgramDataAnaconda3envspy36libsite-packagestensorflowpythonframeworkdtypes.py:523: FutureWarning: Passing (type, 1) or '1type' as a synonym of type is deprecated; in a future version of numpy, it will be understood as (type, (1,)) / '(1,)type'. _np_qint8 = np.dtype([("qint8", np.int8, 1)]) C:ProgramDataAnaconda3envspy36libsite-packagestensorflowpythonframeworkdtypes.py:524: FutureWarning: Passing (type, 1) or '1type' as a synonym of type is deprecated; in a future version of numpy, it will be understood as (type, (1,)) / '(1,)type'. _np_quint8 = np.dtype([("quint8", np.uint8, 1)]) C:ProgramDataAnaconda3envspy36libsite-packagestensorflowpythonframeworkdtypes.py:525: FutureWarning: Passing (type, 1) or '1type' as a synonym of type is deprecated; in a future version of numpy, it will be understood as (type, (1,)) / '(1,)type'. _np_qint16 = np.dtype([("qint16", np.int16, 1)]) C:ProgramDataAnaconda3envspy36libsite-packagestensorflowpythonframeworkdtypes.py:526: FutureWarning: Passing (type, 1) or '1type' as a synonym of type is deprecated; in a future version of numpy, it will be understood as (type, (1,)) / '(1,)type'. _np_quint16 = np.dtype([("quint16", np.uint16, 1)]) C:ProgramDataAnaconda3envspy36libsite-packagestensorflowpythonframeworkdtypes.py:527: FutureWarning: Passing (type, 1) or '1type' as a synonym of type is deprecated; in a future version of numpy, it will be understood as (type, (1,)) / '(1,)type'. _np_qint32 = np.dtype([("qint32", np.int32, 1)]) C:ProgramDataAnaconda3envspy36libsite-packagestensorflowpythonframeworkdtypes.py:532: FutureWarning: Passing (type, 1) or '1type' as a synonym of type is deprecated; in a future version of numpy, it will be understood as (type, (1,)) / '(1,)type'. np_resource = np.dtype([("resource", np.ubyte, 1)]) 2021-03-15 16:39:25.892397: I tensorflow/core/platform/cpu_feature_guard.cc:141] Your CPU supports instructions that this TensorFlow binary was not compiled to use: AVX2 Traceback (most recent call last): File "src/interactive_conditional_samples.py", line 91, in <module> fire.Fire(interact_model) File "C:ProgramDataAnaconda3envspy36libsite-packagesfirecore.py", line 141, in Fire component_trace = _Fire(component, args, parsed_flag_args, context, name) File "C:ProgramDataAnaconda3envspy36libsite-packagesfirecore.py", line 471, in _Fire target=component.__name__) File "C:ProgramDataAnaconda3envspy36libsite-packagesfirecore.py", line 681, in _CallAndUpdateTrace component = fn(*varargs, **kwargs) File "src/interactive_conditional_samples.py", line 65, in interact_model temperature=temperature, top_k=top_k, top_p=top_p File "C:UserslouisOneDriveDesktopgpt-2-testsrcsample.py", line 74, in sample_sequence past, prev, output = body(None, context, context) File "C:UserslouisOneDriveDesktopgpt-2-testsrcsample.py", line 66, in body logits = top_p_logits(logits, p=top_p) File "C:UserslouisOneDriveDesktopgpt-2-testsrcsample.py", line 28, in top_p_logits sorted_logits = tf.sort(logits, direction='DESCENDING', axis=-1) AttributeError: module 'tensorflow' has no attribute 'sort'
I have no idea what any of this means. if someone could help me out it would mean a lot! đ
submitted by /u/LouisHendrich
[visit reddit] [comments]